


What are the differences between llama, alpaca, vicuña and ChatGPT? Evaluation of seven large-scale ChatGPT models
Large-scale language models (LLM) are becoming popular all over the world. One of their important applications is chatting, and they are used in question and answer, customer service and many other aspects. However, chatbots are notoriously difficult to evaluate. Exactly under what circumstances these models are best used is not yet clear. Therefore, the assessment of LLM is very important.
Previously, a Medium blogger named Marco Tulio Ribeiro conducted some complex tasks on Vicuna-13B, MPT-7b-Chat and ChatGPT 3.5 test. The results show that Vicuna is a viable alternative to ChatGPT (3.5) for many tasks, while MPT is not yet ready for real-world use.
Recently, CMU associate professor Graham Neubig conducted a detailed evaluation of seven existing chatbots, produced an open source tool for automatic comparison, and finally formed an evaluation report.
In this report, the evaluator shows the preliminary evaluation and comparison results of some chatbots. The goal is to make it easier for people to understand all the recent open source models and the current status of API-based models.
Specifically, the reviewers created a new open source toolkit, Zeno Build, for evaluating LLM. The toolkit combines: (1) a unified interface for using open source LLM via Hugging Face or the online API; (2) an online interface for browsing and analyzing results using Zeno, and (3) metrics for SOTA evaluation of text using Critique.
Specific results to participate: https://zeno-ml-chatbot-report.hf .space/
The following is a summary of the evaluation results:
- The reviewers evaluated 7 language models: GPT- 2. LLaMa, Alpaca, Vicuna, MPT-Chat, Cohere Command and ChatGPT (gpt-3.5-turbo);
- These models are based on which human-like images are created on the customer service data set The responsiveness of , it is important to use a chat-tuned model with a long context window;
- In the first few turns of the conversation, the prompt project is very useful for improving the performance of the model conversation, but when there are more In the later rounds of multi-context, the effect is not so obvious;
- Even a powerful model like ChatGPT has many obvious problems, such as hallucinations and failure to explore more information, give duplicate content, etc.
- The following are the details of the review.
- Settings
Model Overview
The reviewerused DSTC11 Customer Service Dataset. DSTC11 is a dataset from the Dialogue Systems Technology Challenge that aims to support more informative and engaging task-oriented conversations by leveraging subjective knowledge in comment posts.
The DSTC11 data set contains multiple subtasks, such as multi-turn dialogue, multi-domain dialogue, etc. For example, one of the subtasks is a multi-turn dialogue based on movie reviews, where the dialogue between the user and the system is designed to help the user find movies that suit their tastes. They tested the following
7 models:
- GPT-2: A classic language model in 2019. The reviewers included it as a baseline to see how much recent advances in language modeling impact building better chat models.
- LLaMa: A language model originally trained by Meta AI using the direct language modeling objective. The 7B version of the model was used in the test, and the following open source models also use the same scale version;
- Alpaca: a model based on LLaMa, but with instruction tuning;
- Vicuna: a model based on LLaMa, further explicitly adapted for chatbot-based applications;
- MPT-Chat: a model based on something similar to A model trained from scratch in Vicuna's way, which has a more commercial license;
- Cohere Command: An API-based model launched by Cohere that is fine-tuned for command compliance;
- ChatGPT (gpt-3.5-turbo): Standard API-based chat model, developed by OpenAI.
For all models, the reviewer used the default parameter settings. These include a temperature of 0.3, a context window of 4 previous conversation turns, and a standard prompt: "You are a chatbot tasked with making small-talk with people."
Evaluation Metrics
Evaluators evaluate these models based on how closely their output resembles human customer service responses . This is done using the metrics provided by the Critique toolbox:
- #chrf: measures the overlap of strings;
- BERTScore: Measures the degree of overlap in embeddings between two discourses;
- UniEval Coherence: Predicts how coherent the output is with the previous chat turn.
They also measured length ratio, dividing the length of the output by the length of the gold standard human reply, to measure whether the chatbot was verbose.
Further analysis
In order to dig deeper into the results, the reviewer used Zeno’s analysis interface. Specifically using its report generator, which segments examples based on position in the conversation (beginning, early, middle, and late) and the gold standard length of human responses (short, medium, long), use its explore interface to view Automatically score poor examples and better understand where each model fails.
Results
What is the overall performance of the model?
According to all these metrics, gpt-3.5-turbo is the clear winner; Vicuna is the open source winner; GPT-2 and LLaMa are not very good, indicating that directly in the chat The importance of training.
These rankings also roughly match those of lmsys chat arena, which uses human A/B testing to compare models, but Zeno Build’s The results were obtained without any human scoring.
Regarding output length, the output of gpt3.5-turbo is much verbose than other models, and it seems that models tuned in the chat direction generally give verbose output .
Accuracy of Gold Standard Response Length
Next, the reviewer uses the Zeno report UI to dig deeper. First, they measured accuracy separately by the length of human responses. They classified responses into three categories: short (≤35 characters), medium (36-70 characters), and long (≥71 characters) and evaluated their accuracy individually.
gpt-3.5-turbo and Vicuna maintain accuracy even in longer dialogue rounds, while the other models suffer from a decrease in accuracy.
#The next question is how important is the context window size? The reviewers conducted experiments with Vicuna, and the context window ranged from 1-4 previous discourses. When they increased the context window, model performance increased, indicating that larger context windows are important.
The results show that longer context is especially important in the middle and later parts of the conversation, because these positions There are not so many templates for the reply, and it relies more on what has been said before.
#When trying to generate the gold standard shorter output (probably because there is more ambiguity) , more context is especially important.
How important is the prompt?
The reviewer tried 5 different prompts, 4 of which are universal and the other one is specifically tailored for customer service chat tasks in the insurance field:
- Standard: "You are a chatbot, responsible for chatting with people."
- Friendly: "You are a kind, A friendly chatbot, your task is to chat with people in a pleasant way."
- Polite: "You are a very polite chatbot, Speak very formally and try to avoid making any mistakes in your answers."
- Cynical: "You are a cynical chatbot with a very dark view of the world and usually like to point out anything Possible problems."
- Special to the insurance industry: "You are a staff member at the Rivertown Insurance Help Desk, mainly helping to resolve insurance claim issues."
In general, using these prompts, the reviewers did not detect significant differences caused by different prompts, but the "cynical" chatbot was slightly worse, and the tailor-made "insurance" chatbot Overall slightly better.
The difference brought by different prompts is especially obvious in the first turn of the dialogue, which shows that Prompts are most important when there is little other context to exploit.
Found errors and possible mitigations
Finally, the reviewer used Zeno’s exploration UI to try to pass gpt-3.5 -turbo finds possible errors. Specifically, they looked at all examples with low chrf (
Failure of Probe
Sometimes the model cannot Probe (detect) more information when actually needed. For example, the model is not yet perfect in handling numbers (the phone number must be 11 digits, and the length of the number given by the model is not consistent with the answer. match). This can be alleviated by modifying prompt to remind the model of the required length of certain information.
Duplicate content
Sometimes, the same content is repeated multiple times , for example, the chatbot said "thank you" twice here.
Answers that make sense, but not in the human way
Sometimes, This response is reasonable, just different from how humans would react.
The above are the evaluation results. Finally, the reviewers hope that this report will be helpful to researchers! If you continue to want to try other models, datasets, prompts, or other hyperparameter settings, you can jump to the chatbot example on the zeno-build repository to try it out.
The above is the detailed content of What are the differences between llama, alpaca, vicuña and ChatGPT? Evaluation of seven large-scale ChatGPT models. For more information, please follow other related articles on the PHP Chinese website!

Hot AI Tools

Undresser.AI Undress
AI-powered app for creating realistic nude photos

AI Clothes Remover
Online AI tool for removing clothes from photos.

Undress AI Tool
Undress images for free

Clothoff.io
AI clothes remover

Video Face Swap
Swap faces in any video effortlessly with our completely free AI face swap tool!

Hot Article

Hot Tools

Notepad++7.3.1
Easy-to-use and free code editor

SublimeText3 Chinese version
Chinese version, very easy to use

Zend Studio 13.0.1
Powerful PHP integrated development environment

Dreamweaver CS6
Visual web development tools

SublimeText3 Mac version
God-level code editing software (SublimeText3)

Hot Topics










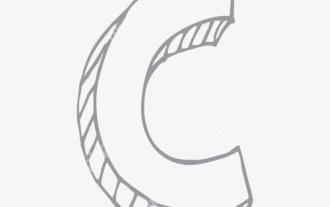
Using the chrono library in C can allow you to control time and time intervals more accurately. Let's explore the charm of this library. C's chrono library is part of the standard library, which provides a modern way to deal with time and time intervals. For programmers who have suffered from time.h and ctime, chrono is undoubtedly a boon. It not only improves the readability and maintainability of the code, but also provides higher accuracy and flexibility. Let's start with the basics. The chrono library mainly includes the following key components: std::chrono::system_clock: represents the system clock, used to obtain the current time. std::chron
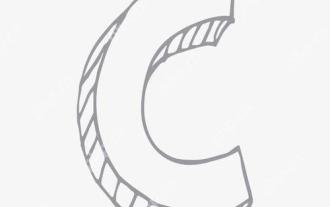
DMA in C refers to DirectMemoryAccess, a direct memory access technology, allowing hardware devices to directly transmit data to memory without CPU intervention. 1) DMA operation is highly dependent on hardware devices and drivers, and the implementation method varies from system to system. 2) Direct access to memory may bring security risks, and the correctness and security of the code must be ensured. 3) DMA can improve performance, but improper use may lead to degradation of system performance. Through practice and learning, we can master the skills of using DMA and maximize its effectiveness in scenarios such as high-speed data transmission and real-time signal processing.
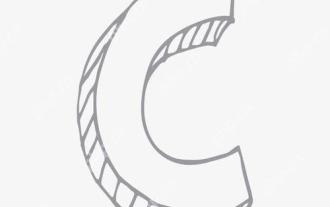
Handling high DPI display in C can be achieved through the following steps: 1) Understand DPI and scaling, use the operating system API to obtain DPI information and adjust the graphics output; 2) Handle cross-platform compatibility, use cross-platform graphics libraries such as SDL or Qt; 3) Perform performance optimization, improve performance through cache, hardware acceleration, and dynamic adjustment of the details level; 4) Solve common problems, such as blurred text and interface elements are too small, and solve by correctly applying DPI scaling.
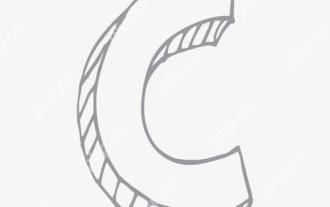
C performs well in real-time operating system (RTOS) programming, providing efficient execution efficiency and precise time management. 1) C Meet the needs of RTOS through direct operation of hardware resources and efficient memory management. 2) Using object-oriented features, C can design a flexible task scheduling system. 3) C supports efficient interrupt processing, but dynamic memory allocation and exception processing must be avoided to ensure real-time. 4) Template programming and inline functions help in performance optimization. 5) In practical applications, C can be used to implement an efficient logging system.
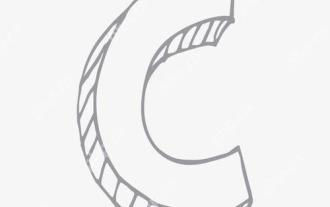
Measuring thread performance in C can use the timing tools, performance analysis tools, and custom timers in the standard library. 1. Use the library to measure execution time. 2. Use gprof for performance analysis. The steps include adding the -pg option during compilation, running the program to generate a gmon.out file, and generating a performance report. 3. Use Valgrind's Callgrind module to perform more detailed analysis. The steps include running the program to generate the callgrind.out file and viewing the results using kcachegrind. 4. Custom timers can flexibly measure the execution time of a specific code segment. These methods help to fully understand thread performance and optimize code.
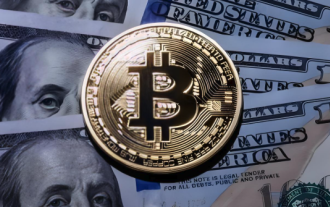
The built-in quantization tools on the exchange include: 1. Binance: Provides Binance Futures quantitative module, low handling fees, and supports AI-assisted transactions. 2. OKX (Ouyi): Supports multi-account management and intelligent order routing, and provides institutional-level risk control. The independent quantitative strategy platforms include: 3. 3Commas: drag-and-drop strategy generator, suitable for multi-platform hedging arbitrage. 4. Quadency: Professional-level algorithm strategy library, supporting customized risk thresholds. 5. Pionex: Built-in 16 preset strategy, low transaction fee. Vertical domain tools include: 6. Cryptohopper: cloud-based quantitative platform, supporting 150 technical indicators. 7. Bitsgap:
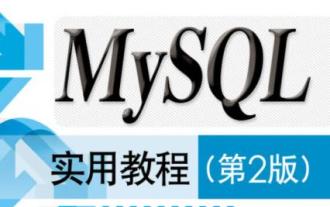
In MySQL, add fields using ALTERTABLEtable_nameADDCOLUMNnew_columnVARCHAR(255)AFTERexisting_column, delete fields using ALTERTABLEtable_nameDROPCOLUMNcolumn_to_drop. When adding fields, you need to specify a location to optimize query performance and data structure; before deleting fields, you need to confirm that the operation is irreversible; modifying table structure using online DDL, backup data, test environment, and low-load time periods is performance optimization and best practice.
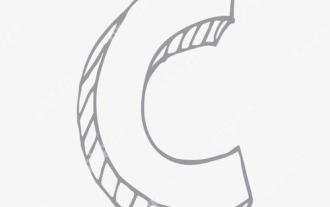
The main steps and precautions for using string streams in C are as follows: 1. Create an output string stream and convert data, such as converting integers into strings. 2. Apply to serialization of complex data structures, such as converting vector into strings. 3. Pay attention to performance issues and avoid frequent use of string streams when processing large amounts of data. You can consider using the append method of std::string. 4. Pay attention to memory management and avoid frequent creation and destruction of string stream objects. You can reuse or use std::stringstream.
