How to use Pandas for data analysis in Python
First, make sure you have the Pandas library installed. If not, please use the following command to install it:
pip install pandas
1. Import the Pandas library
import pandas as pd
2. Read data
Using Pandas, you can easily read a variety of data Format, including CSV, Excel, JSON and HTML, etc. The following is an example of reading a CSV file:
data = pd.read_csv('data.csv')
The reading methods of other data formats are similar, such as reading Excel files:
data = pd.read_excel('data.xlsx')
3. View data
You can use head()
function to view the first few rows of data (default is 5 rows):
print(data.head())
You can also use the tail()
function to view the last few rows of data, And info()
and describe()
functions to view the statistical information of the data:
print(data.tail()) print(data.info()) print(data.describe())
4. Select data
There are many ways to select data , the following are some common methods:
Select a column:
data['column_name']
Select multiple columns :
data[['column1', 'column2']]
Select a row:
data.loc[row_index]
Select a value:
data.loc[row_index, 'column_name']
Select by condition:
data [data['column_name'] > value]
5. Data cleaning
Before data analysis, the data usually needs to be cleaned. The following are some commonly used data cleaning methods:
Remove null values:
data.dropna()
Replace null values Value:
data.fillna(value)
Rename column name:
data.rename(columns={'old_name': 'new_name'})
Data type conversion:
data['column_name'].astype(new_type)
Remove duplicates Value:
data.drop_duplicates()
6. Data analysis
Pandas provides rich data analysis functions. The following are some common methods:
Calculate the mean:
data['column_name'].mean()
Calculate the median:
data['column_name'].median()
Calculate the mode:
data['column_name'].mode()
Calculate standard deviation:
data['column_name'].std()
Calculate correlation:
data. corr()
Data grouping:
data.groupby('column_name')
7. Data Visualization
Pandas makes it easy to transform data into visual charts. First, you need to install the Matplotlib library:
pip install matplotlib
Then, use the following code to create a chart:
import matplotlib.pyplot as plt data['column_name'].plot(kind='bar') plt.show()
Other visualization chart types include line charts, pie charts, histograms, etc.:
data['column_name'].plot(kind='line') data['column_name'].plot(kind='pie') data['column_name'].plot(kind='hist') plt.show()
8. Export data
Pandas can export data to a variety of formats, such as CSV, Excel, JSON, HTML, etc. The following is an example of exporting data to a CSV file:
data.to_csv('output.csv', index=False)
The export method for other data formats is similar, such as exporting to an Excel file:
data.to_excel('output.xlsx', index=False)
9. Practical cases
us Assume that you already have a sales data (sales_data.csv), the next goal is to analyze the data. First, we need to read the data:
import pandas as pd data = pd.read_csv('sales_data.csv')
Then, we can clean and analyze the data. For example, we can calculate the sales of each product:
data['sales_amount'] = data['quantity'] * data['price']
Next, we can analyze which product has the highest sales:
max_sales = data.groupby('product_name')['sales_amount'].sum().idxmax() print(f'最高销售额的产品是:{max_sales}')
Finally, we can export the results to a CSV file:
data.to_csv('sales_analysis.csv', index=False)
The above is the detailed content of How to use Pandas for data analysis in Python. For more information, please follow other related articles on the PHP Chinese website!

Hot AI Tools

Undresser.AI Undress
AI-powered app for creating realistic nude photos

AI Clothes Remover
Online AI tool for removing clothes from photos.

Undress AI Tool
Undress images for free

Clothoff.io
AI clothes remover

Video Face Swap
Swap faces in any video effortlessly with our completely free AI face swap tool!

Hot Article

Hot Tools

Notepad++7.3.1
Easy-to-use and free code editor

SublimeText3 Chinese version
Chinese version, very easy to use

Zend Studio 13.0.1
Powerful PHP integrated development environment

Dreamweaver CS6
Visual web development tools

SublimeText3 Mac version
God-level code editing software (SublimeText3)

Hot Topics










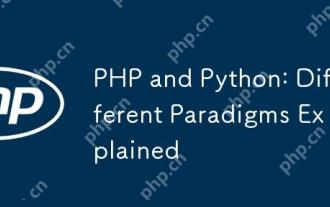
PHP is mainly procedural programming, but also supports object-oriented programming (OOP); Python supports a variety of paradigms, including OOP, functional and procedural programming. PHP is suitable for web development, and Python is suitable for a variety of applications such as data analysis and machine learning.
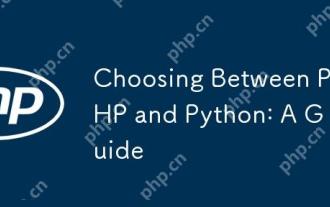
PHP is suitable for web development and rapid prototyping, and Python is suitable for data science and machine learning. 1.PHP is used for dynamic web development, with simple syntax and suitable for rapid development. 2. Python has concise syntax, is suitable for multiple fields, and has a strong library ecosystem.
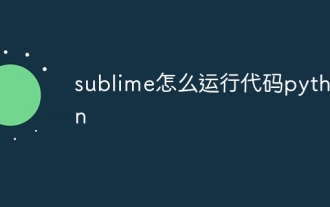
To run Python code in Sublime Text, you need to install the Python plug-in first, then create a .py file and write the code, and finally press Ctrl B to run the code, and the output will be displayed in the console.
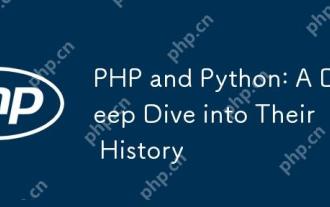
PHP originated in 1994 and was developed by RasmusLerdorf. It was originally used to track website visitors and gradually evolved into a server-side scripting language and was widely used in web development. Python was developed by Guidovan Rossum in the late 1980s and was first released in 1991. It emphasizes code readability and simplicity, and is suitable for scientific computing, data analysis and other fields.
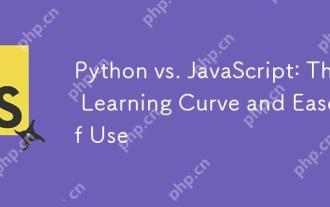
Python is more suitable for beginners, with a smooth learning curve and concise syntax; JavaScript is suitable for front-end development, with a steep learning curve and flexible syntax. 1. Python syntax is intuitive and suitable for data science and back-end development. 2. JavaScript is flexible and widely used in front-end and server-side programming.
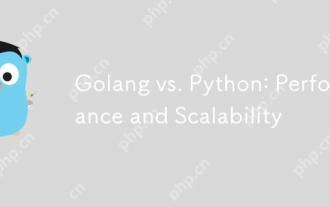
Golang is better than Python in terms of performance and scalability. 1) Golang's compilation-type characteristics and efficient concurrency model make it perform well in high concurrency scenarios. 2) Python, as an interpreted language, executes slowly, but can optimize performance through tools such as Cython.
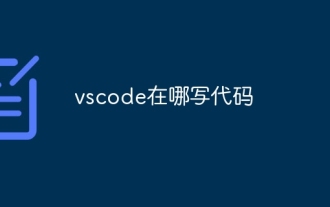
Writing code in Visual Studio Code (VSCode) is simple and easy to use. Just install VSCode, create a project, select a language, create a file, write code, save and run it. The advantages of VSCode include cross-platform, free and open source, powerful features, rich extensions, and lightweight and fast.
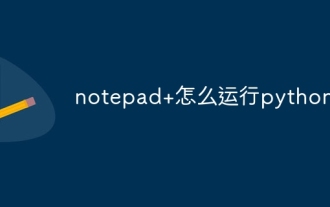
Running Python code in Notepad requires the Python executable and NppExec plug-in to be installed. After installing Python and adding PATH to it, configure the command "python" and the parameter "{CURRENT_DIRECTORY}{FILE_NAME}" in the NppExec plug-in to run Python code in Notepad through the shortcut key "F6".
