What are the common current limiting algorithms in Java?
01 Fixed Window
Fixed window, also known as fixed window (also known as counter algorithm, Fixed Window) current limiting algorithm, is the simplest current limiting algorithm. It is controlled by a counter maintained within a unit time. The maximum number of visits within a time unit.
Assuming that the number of requests per minute is limited to no more than 60, set a counter. When the request arrives, if the counter reaches the threshold, the request will be rejected, otherwise the counter will be incremented by 1; the counter will be reset to 0 every minute. The code is implemented as follows:
public class CounterRateLimiter extends MyRateLimiter { /** * 每秒限制请求数 */ private final long permitsPerSecond; /** * 上一个窗口的开始时间 */ public long timestamp = System.currentTimeMillis(); /** * 计数器 */ private int counter; public CounterRateLimiter(long permitsPerSecond) { this.permitsPerSecond = permitsPerSecond; } @Override public synchronized boolean tryAcquire() { long now = System.currentTimeMillis(); // 窗口内请求数量小于阈值,更新计数放行,否则拒绝请求 if (now - timestamp < 1000) { if (counter < permitsPerSecond) { counter++; return true; } else { return false; } } // 时间窗口过期,重置计数器和时间戳 counter = 0; timestamp = now; return true; } }
The biggest advantage of the fixed window is that it is easy to implement; and the memory footprint is small, we only need to store the count in the time window; it can ensure that more latest requests are processed, and will not be due to The accumulation of old requests causes new requests to be starved to death. Of course, it also faces critical problems. When two windows meet, the instantaneous traffic may be 2n.
02 Sliding window
In order to prevent instantaneous traffic, the fixed window can be further divided into multiple grids, and each time it moves backward a small grid, instead of fixing the window size, this is sliding Window (Sliding Window).
For example, each minute can be divided into 6 cells of 10 seconds. A counter is maintained in each cell, and the window slides forward one cell at a time. Whenever a request arrives, it can be released as long as the sum of the counts of all cells in the window does not exceed the threshold. The transmission of data packets in the TCP protocol also uses sliding windows for flow control.
The implementation is as follows:
public class SlidingWindowRateLimiter extends MyRateLimiter { /** * 每分钟限制请求数 */ private final long permitsPerMinute; /** * 计数器, k-为当前窗口的开始时间值秒,value为当前窗口的计数 */ private final TreeMap<Long, Integer> counters; public SlidingWindowRateLimiter(long permitsPerMinute) { this.permitsPerMinute = permitsPerMinute; this.counters = new TreeMap<>(); } @Override public synchronized boolean tryAcquire() { // 获取当前时间的所在的子窗口值; 10s一个窗口 long currentWindowTime = LocalDateTime.now().toEpochSecond(ZoneOffset.UTC) / 10 * 10; // 获取当前窗口的请求总量 int currentWindowCount = getCurrentWindowCount(currentWindowTime); if (currentWindowCount >= permitsPerMinute) { return false; } // 计数器 + 1 counters.merge(currentWindowTime, 1, Integer::sum); return true; } /** * 获取当前窗口中的所有请求数(并删除所有无效的子窗口计数器) * * @param currentWindowTime 当前子窗口时间 * @return 当前窗口中的计数 */ private int getCurrentWindowCount(long currentWindowTime) { // 计算出窗口的开始位置时间 long startTime = currentWindowTime - 50; int result = 0; // 遍历当前存储的计数器,删除无效的子窗口计数器,并累加当前窗口中的所有计数器之和 Iterator<Map.Entry<Long, Integer>> iterator = counters.entrySet().iterator(); while (iterator.hasNext()) { Map.Entry<Long, Integer> entry = iterator.next(); if (entry.getKey() < startTime) { iterator.remove(); } else { result += entry.getValue(); } } return result; } }
The sliding window solves the problem of instantaneous traffic peaks in the counter. In fact, the counter algorithm is also a type of sliding window, but the window is not divided into finer-grained units. Comparing the counters, it can be seen that when the granularity of window division is finer, the flow control is more precise and strict.
However, when the traffic in the window reaches the threshold, the traffic will be cut off instantly. In actual applications, the flow limiting effect we want is often not to cut off the traffic all at once, but to allow the traffic to enter the system smoothly.
03 Leaky Bucket Algorithm
How to limit the flow more smoothly? Let's take a look at the Leaky Bucket algorithm. Requests are injected into the leaky bucket at any speed like water, and the bucket will leak water at a fixed rate; when the injection speed continues to be greater than the leakage speed, the leaky bucket will become full. , at this time newly incoming requests will be discarded. Current limiting and shaping are the two core capabilities of the leaky bucket algorithm.
The implementation is as follows:
public class LeakyBucketRateLimiter extends MyRateLimiter { // 桶的容量 private final int capacity; // 漏出速率 private final int permitsPerSecond; // 剩余水量 private long leftWater; // 上次注入时间 private long timeStamp = System.currentTimeMillis(); public LeakyBucketRateLimiter(int permitsPerSecond, int capacity) { this.capacity = capacity; this.permitsPerSecond = permitsPerSecond; } @Override public synchronized boolean tryAcquire() { //1. 计算剩余水量 long now = System.currentTimeMillis(); long timeGap = (now - timeStamp) / 1000; leftWater = Math.max(0, leftWater - timeGap * permitsPerSecond); timeStamp = now; // 如果未满,则放行;否则限流 if (leftWater < capacity) { leftWater += 1; return true; } return false; } }
This is not a complete implementation of the leaky bucket algorithm. The above code only verifies whether the traffic will be abandoned, that is, tryAcquire returns true to indicate that the leaky bucket has not been full, otherwise it means that the leaky bucket is full and the request is discarded.
If you want to leak traffic at a constant rate, you should usually implement it with a FIFO queue. When tryAcquire returns true, the request is queued, and then the request is taken out of the queue at a fixed frequency for processing. The sample code is as follows:
@Test public void testLeakyBucketRateLimiter() throws InterruptedException { ScheduledExecutorService scheduledExecutorService = Executors.newSingleThreadScheduledExecutor(); ExecutorService singleThread = Executors.newSingleThreadExecutor(); LeakyBucketRateLimiter rateLimiter = new LeakyBucketRateLimiter(20, 20); // 存储流量的队列 Queue<Integer> queue = new LinkedList<>(); // 模拟请求 不确定速率注水 singleThread.execute(() -> { int count = 0; while (true) { count++; boolean flag = rateLimiter.tryAcquire(); if (flag) { queue.offer(count); System.out.println(count + "--------流量被放行--------"); } else { System.out.println(count + "流量被限制"); } try { Thread.sleep((long) (Math.random() * 1000)); } catch (InterruptedException e) { e.printStackTrace(); } } }); // 模拟处理请求 固定速率漏水 scheduledExecutorService.scheduleAtFixedRate(() -> { if (!queue.isEmpty()) { System.out.println(queue.poll() + "被处理"); } }, 0, 100, TimeUnit.MILLISECONDS); // 保证主线程不会退出 while (true) { Thread.sleep(10000); } }
The purpose of the leaky bucket algorithm is mainly to smooth burst traffic and provide a mechanism to ensure that burst traffic in the network is integrated into smooth and stable traffic.
However, because leaky buckets control traffic too strictly, system resources cannot be fully used in some scenarios, because the leakage rate of leaky buckets is fixed, even if the downstream can handle greater traffic at a certain moment, Leaky buckets also do not allow burst traffic to pass through.
04Token Bucket
How to allow burst traffic while limiting the traffic rate? Learn about the token bucket algorithm! The token bucket algorithm sends tokens to the token bucket at a constant rate. When the request arrives, it tries to get the token from the token bucket. Only when the token is obtained can it be released, otherwise it will be rejected.
The token bucket has the following characteristics:
1. Tokens are issued at a constant rate. Assuming that the current limit rate is v/s, it means that one token is issued every 1/v seconds
2. Assume that the token bucket capacity is b. If the token bucket is full, the new token will be discarded
3. The prerequisite for the request to pass the current limiter is the token bucket There are tokens in
There are two parameters worthy of attention in the token bucket algorithm, namely the current limit rate v/s, and the token bucket capacity b; the rate a represents the current limit of the current limiter under normal circumstances. rate, and b is the abbreviation of burst, indicating the maximum burst traffic allowed by the current limiter.
For example, b=10. When the token bucket is full, there are 10 available tokens. At this time, 10 requests are allowed to pass through the flow limiter at the same time (a certain degree of traffic burst is allowed). These 10 requests After the token is consumed instantly, subsequent traffic can only pass through the flow limiter at the rate r.
The implementation is as follows:
public class TokenBucketRateLimiter extends MyRateLimiter { /** * 令牌桶的容量「限流器允许的最大突发流量」 */ private final long capacity; /** * 令牌发放速率 */ private final long generatedPerSeconds; /** * 最后一个令牌发放的时间 */ long lastTokenTime = System.currentTimeMillis(); /** * 当前令牌数量 */ private long currentTokens; public TokenBucketRateLimiter(long generatedPerSeconds, int capacity) { this.generatedPerSeconds = generatedPerSeconds; this.capacity = capacity; } /** * 尝试获取令牌 * * @return true表示获取到令牌,放行;否则为限流 */ @Override public synchronized boolean tryAcquire() { /** * 计算令牌当前数量 * 请求时间在最后令牌是产生时间相差大于等于额1s(为啥时1s?因为生成令牌的最小时间单位时s),则 * 1. 重新计算令牌桶中的令牌数 * 2. 将最后一个令牌发放时间重置为当前时间 */ long now = System.currentTimeMillis(); if (now - lastTokenTime >= 1000) { long newPermits = (now - lastTokenTime) / 1000 * generatedPerSeconds; currentTokens = Math.min(currentTokens + newPermits, capacity); lastTokenTime = now; } if (currentTokens > 0) { currentTokens--; return true; } return false; } }
What you need to know is that the very easy to think of implementation is the producer-consumer pattern; use a producer thread to regularly add tokens to the blocking queue, and try to The thread that passes the current limiter acts as a consumer thread, and is only allowed to pass the current limiter if it obtains a token from the blocking queue.
Due to the uncertainty of thread scheduling, the timer error is very large in high concurrency scenarios. At the same time, the timer itself will create scheduling threads, which will also affect the performance of the system.
05 Sliding log
Sliding log is a relatively "unpopular" but really easy to use current limiting algorithm. The sliding log rate limiting algorithm needs to record the timestamp of the request, which is usually stored using an ordered collection. We can track all the user's requests within a time period in a single ordered collection.
假设我们要限制给定T时间内的请求不超过N,我们只需要存储最近T时间之内的请求日志,每当请求到来时判断最近T时间内的请求总数是否超过阈值。
实现如下:
public class SlidingLogRateLimiter extends MyRateLimiter { /** * 每分钟限制请求数 */ private static final long PERMITS_PER_MINUTE = 60; /** * 请求日志计数器, k-为请求的时间(秒),value当前时间的请求数量 */ private final TreeMap<Long, Integer> requestLogCountMap = new TreeMap<>(); @Override public synchronized boolean tryAcquire() { // 最小时间粒度为s long currentTimestamp = LocalDateTime.now().toEpochSecond(ZoneOffset.UTC); // 获取当前窗口的请求总数 int currentWindowCount = getCurrentWindowCount(currentTimestamp); if (currentWindowCount >= PERMITS_PER_MINUTE) { return false; } // 请求成功,将当前请求日志加入到日志中 requestLogCountMap.merge(currentTimestamp, 1, Integer::sum); return true; } /** * 统计当前时间窗口内的请求数 * * @param currentTime 当前时间 * @return - */ private int getCurrentWindowCount(long currentTime) { // 计算出窗口的开始位置时间 long startTime = currentTime - 59; // 遍历当前存储的计数器,删除无效的子窗口计数器,并累加当前窗口中的所有计数器之和 return requestLogCountMap.entrySet() .stream() .filter(entry -> entry.getKey() >= startTime) .mapToInt(Map.Entry::getValue) .sum(); } }
滑动日志能够避免突发流量,实现较为精准的限流;同样更加灵活,能够支持更加复杂的限流策略,如多级限流,每分钟不超过100次,每小时不超过300次,每天不超过1000次,我们只需要保存最近24小时所有的请求日志即可实现。
灵活并不是没有代价的,带来的缺点就是占用存储空间要高于其他限流算法。
06分布式限流
以上几种限流算法的实现都仅适合单机限流。虽然给每台机器平均分配限流配额可以达到限流的目的,但是由于机器性能,流量分布不均以及计算数量动态变化等问题,单机限流在分布式场景中的效果总是差强人意。
分布式限流最简单的实现就是利用中心化存储,即将单机限流存储在本地的数据存储到同一个存储空间中,如常见的Redis等。
当然也可以从上层流量入口进行限流,Nginx代理服务就提供了限流模块,同样能够实现高性能,精准的限流,其底层是漏桶算法。
The above is the detailed content of What are the common current limiting algorithms in Java?. For more information, please follow other related articles on the PHP Chinese website!

Hot AI Tools

Undresser.AI Undress
AI-powered app for creating realistic nude photos

AI Clothes Remover
Online AI tool for removing clothes from photos.

Undress AI Tool
Undress images for free

Clothoff.io
AI clothes remover

Video Face Swap
Swap faces in any video effortlessly with our completely free AI face swap tool!

Hot Article

Hot Tools

Notepad++7.3.1
Easy-to-use and free code editor

SublimeText3 Chinese version
Chinese version, very easy to use

Zend Studio 13.0.1
Powerful PHP integrated development environment

Dreamweaver CS6
Visual web development tools

SublimeText3 Mac version
God-level code editing software (SublimeText3)

Hot Topics










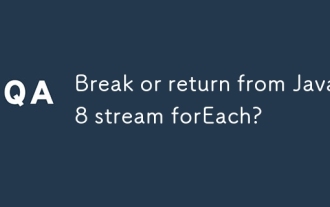
Java 8 introduces the Stream API, providing a powerful and expressive way to process data collections. However, a common question when using Stream is: How to break or return from a forEach operation? Traditional loops allow for early interruption or return, but Stream's forEach method does not directly support this method. This article will explain the reasons and explore alternative methods for implementing premature termination in Stream processing systems. Further reading: Java Stream API improvements Understand Stream forEach The forEach method is a terminal operation that performs one operation on each element in the Stream. Its design intention is
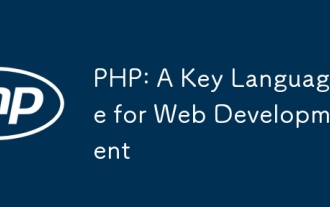
PHP is a scripting language widely used on the server side, especially suitable for web development. 1.PHP can embed HTML, process HTTP requests and responses, and supports a variety of databases. 2.PHP is used to generate dynamic web content, process form data, access databases, etc., with strong community support and open source resources. 3. PHP is an interpreted language, and the execution process includes lexical analysis, grammatical analysis, compilation and execution. 4.PHP can be combined with MySQL for advanced applications such as user registration systems. 5. When debugging PHP, you can use functions such as error_reporting() and var_dump(). 6. Optimize PHP code to use caching mechanisms, optimize database queries and use built-in functions. 7
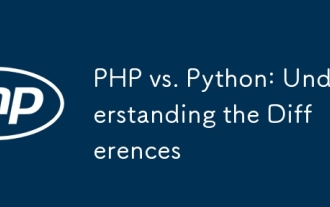
PHP and Python each have their own advantages, and the choice should be based on project requirements. 1.PHP is suitable for web development, with simple syntax and high execution efficiency. 2. Python is suitable for data science and machine learning, with concise syntax and rich libraries.
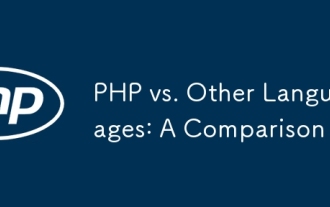
PHP is suitable for web development, especially in rapid development and processing dynamic content, but is not good at data science and enterprise-level applications. Compared with Python, PHP has more advantages in web development, but is not as good as Python in the field of data science; compared with Java, PHP performs worse in enterprise-level applications, but is more flexible in web development; compared with JavaScript, PHP is more concise in back-end development, but is not as good as JavaScript in front-end development.
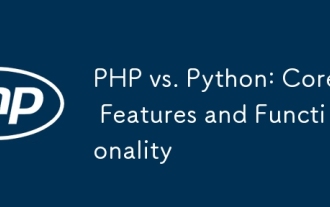
PHP and Python each have their own advantages and are suitable for different scenarios. 1.PHP is suitable for web development and provides built-in web servers and rich function libraries. 2. Python is suitable for data science and machine learning, with concise syntax and a powerful standard library. When choosing, it should be decided based on project requirements.
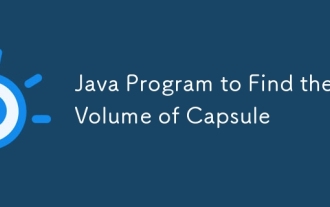
Capsules are three-dimensional geometric figures, composed of a cylinder and a hemisphere at both ends. The volume of the capsule can be calculated by adding the volume of the cylinder and the volume of the hemisphere at both ends. This tutorial will discuss how to calculate the volume of a given capsule in Java using different methods. Capsule volume formula The formula for capsule volume is as follows: Capsule volume = Cylindrical volume Volume Two hemisphere volume in, r: The radius of the hemisphere. h: The height of the cylinder (excluding the hemisphere). Example 1 enter Radius = 5 units Height = 10 units Output Volume = 1570.8 cubic units explain Calculate volume using formula: Volume = π × r2 × h (4
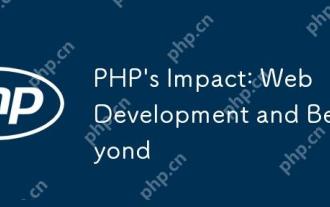
PHPhassignificantlyimpactedwebdevelopmentandextendsbeyondit.1)ItpowersmajorplatformslikeWordPressandexcelsindatabaseinteractions.2)PHP'sadaptabilityallowsittoscaleforlargeapplicationsusingframeworkslikeLaravel.3)Beyondweb,PHPisusedincommand-linescrip
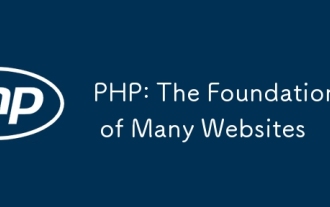
The reasons why PHP is the preferred technology stack for many websites include its ease of use, strong community support, and widespread use. 1) Easy to learn and use, suitable for beginners. 2) Have a huge developer community and rich resources. 3) Widely used in WordPress, Drupal and other platforms. 4) Integrate tightly with web servers to simplify development deployment.
