ChatGPT topic one GPT family evolution history
Timeline
June 2018
OpenAI released the GPT-1 model with 110 million parameters.
In November 2018
OpenAI released the GPT-2 model with 1.5 billion parameters, but due to concerns about abuse, all the code and data of the model are not open to the public.
February 2019
OpenAI opened some code and data of the GPT-2 model, but access is still restricted.
June 10, 2019
OpenAI released the GPT-3 model with 175 billion parameters and provided access to some partners.
September 2019
OpenAI opened all the code and data of GPT-2 and released a larger version.
In May 2020
OpenAI announced the launch of the beta version of the GPT-3 model, which has 175 billion parameters and is the largest natural language processing model to date.
March 2022
OpenAI released InstructGPT, using Instruction Tuning
November 30, 2022
OpenAI passed the GPT-3.5 series of large-scale languages The new conversational AI model ChatGPT is officially released after fine-tuning the model.
December 15, 2022
The first update to ChatGPT, which improves overall performance and adds new features to save and view historical conversation records.
January 9, 2023
ChatGPT was updated for the second time, improving the authenticity of answers and adding a new "stop generation" function.
January 21, 2023
OpenAI released a paid version of ChatGPT Professional that is limited to some users.
January 30, 2023
The third update of ChatGPT not only improves the authenticity of answers, but also improves mathematical skills.
February 2, 2023
OpenAI officially launched the ChatGPT paid version subscription service. The new version responds faster and runs more stably than the free version.
March 15, 2023
OpenAI shockingly launched the large-scale multi-modal model GPT-4, which can not only read text, but also recognize images and generate text results. It is now connected ChatGPT is open to Plus users.
GPT-1: Pre-trained model based on one-way Transformer
Before the emergence of GPT, NLP models were mainly trained based on large amounts of annotated data for specific tasks. This will lead to some limitations:
Large-scale high-quality annotation data is not easy to obtain;
The model is limited to the training it has received, and its generalization ability is insufficient;
It cannot be executed Out-of-the-box tasks limit the practical application of the model.
In order to overcome these problems, OpenAI embarked on the path of pre-training large models. GPT-1 is the first pre-trained model released by OpenAI in 2018. It adopts a one-way Transformer model and uses more than 40GB of text data for training. The key features of GPT-1 are: generative pre-training (unsupervised) and discriminative task fine-tuning (supervised). First, we used unsupervised learning pre-training and spent 1 month on 8 GPUs to enhance the language capabilities of the AI system from a large amount of unlabeled data and obtain a large amount of knowledge. Then we conducted supervised fine-tuning and compared it with large data sets. Integrated to improve system performance in NLP tasks. GPT-1 showed excellent performance in text generation and understanding tasks, becoming one of the most advanced natural language processing models at the time.
GPT-2: Multi-task pre-training model
Since the single-task model lacks generalization and multi-task learning requires a large number of effective training pairs, GPT-2 is It has been expanded and optimized on the basis of GPT-1, removing supervised learning and only retaining unsupervised learning. GPT-2 uses larger text data and more powerful computing resources for training, and the parameter size reaches 150 million, far exceeding the 110 million parameters of GPT-1. In addition to using larger data sets and larger models to learn, GPT-2 also proposes a new and more difficult task: zero-shot learning (zero-shot), which is to directly apply pre-trained models to many downstream Task. GPT-2 has demonstrated excellent performance on multiple natural language processing tasks, including text generation, text classification, language understanding, etc.
GPT-3: Creating new natural language generation and understanding capabilities
GPT-3 is the latest in the GPT series of models A model that uses a larger parameter scale and richer training data. The parameter scale of GPT-3 reaches 1.75 trillion, which is more than 100 times that of GPT-2. GPT-3 has shown amazing capabilities in natural language generation, dialogue generation and other language processing tasks. In some tasks, it can even create new forms of language expression.
GPT-3 proposes a very important concept: In-context learning. The specific content will be explained in the next tweet.
InstructGPT & ChatGPT
The training of InstructGPT/ChatGPT is divided into 3 steps, and the data required for each step is slightly different. We will introduce them separately below.
Start with a pre-trained language model and apply the following three steps.
Step 1: Supervised fine-tuning SFT: Collect demonstration data and train a supervised policy. Our tagger provides a demonstration of the desired behavior on the input prompt distribution. We then use supervised learning to fine-tune the pre-trained GPT-3 model on these data.
Step 2: Reward Model training. Collect comparative data and train a reward model. We collected a dataset of comparisons between model outputs, where labelers indicate which output they prefer for a given input. We then train a reward model to predict human-preferred outputs.
Step 3: Reinforcement learning via proximal policy optimization (PPO) on the reward model: use the output of the RM as the scalar reward. We use the PPO algorithm to fine-tune the supervision strategy to optimize this reward.
Steps 2 and 3 can be iterated continuously; more comparison data is collected on the current optimal strategy, which is used to train a new RM, and then a new strategy.
The prompts in the first two steps come from user usage data on OpenAI’s online API and handwritten by hired annotators. The last step is all sampled from the API data. The specific data of InstructGPT:
1. SFT data set
The SFT data set is used to train the first step The supervised model uses the new data collected to fine-tune GPT-3 according to the training method of GPT-3. Because GPT-3 is a generative model based on prompt learning, the SFT dataset is also a sample composed of prompt-reply pairs. Part of the SFT data comes from users of OpenAI’s PlayGround, and the other part comes from the 40 labelers employed by OpenAI. And they trained the labeler. In this dataset, the annotator's job is to write instructions themselves based on the content.
2. RM data set
The RM data set is used to train the reward model in step 2. We also need to set a reward target for the training of InstructGPT/ChatGPT. This reward goal does not have to be differentiable, but it must align as comprehensively and realistically as possible with what we need the model to generate. Naturally, we can provide this reward through manual annotation. Through artificial pairing, we can give lower scores to the generated content involving bias to encourage the model not to generate content that humans do not like. The approach of InstructGPT/ChatGPT is to first let the model generate a batch of candidate texts, and then use the labeler to sort the generated content according to the quality of the generated data.
3. PPO data set
The PPO data of InstructGPT is not annotated. It comes from GPT-3 API users. There are different types of generation tasks provided by different users, with the highest proportion including generation tasks (45.6%), QA (12.4%), brainstorming (11.2%), dialogue (8.4%), etc.
Appendix:
Sources of various capabilities of ChatGPT:
The above is the detailed content of ChatGPT topic one GPT family evolution history. For more information, please follow other related articles on the PHP Chinese website!

Hot AI Tools

Undresser.AI Undress
AI-powered app for creating realistic nude photos

AI Clothes Remover
Online AI tool for removing clothes from photos.

Undress AI Tool
Undress images for free

Clothoff.io
AI clothes remover

Video Face Swap
Swap faces in any video effortlessly with our completely free AI face swap tool!

Hot Article

Hot Tools

Notepad++7.3.1
Easy-to-use and free code editor

SublimeText3 Chinese version
Chinese version, very easy to use

Zend Studio 13.0.1
Powerful PHP integrated development environment

Dreamweaver CS6
Visual web development tools

SublimeText3 Mac version
God-level code editing software (SublimeText3)

Hot Topics










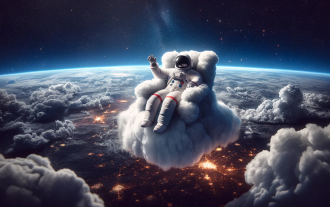
DALL-E 3 was officially introduced in September of 2023 as a vastly improved model than its predecessor. It is considered one of the best AI image generators to date, capable of creating images with intricate detail. However, at launch, it was exclus
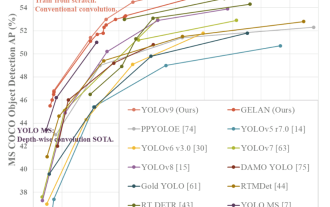
Today's deep learning methods focus on designing the most suitable objective function so that the model's prediction results are closest to the actual situation. At the same time, a suitable architecture must be designed to obtain sufficient information for prediction. Existing methods ignore the fact that when the input data undergoes layer-by-layer feature extraction and spatial transformation, a large amount of information will be lost. This article will delve into important issues when transmitting data through deep networks, namely information bottlenecks and reversible functions. Based on this, the concept of programmable gradient information (PGI) is proposed to cope with the various changes required by deep networks to achieve multi-objectives. PGI can provide complete input information for the target task to calculate the objective function, thereby obtaining reliable gradient information to update network weights. In addition, a new lightweight network framework is designed
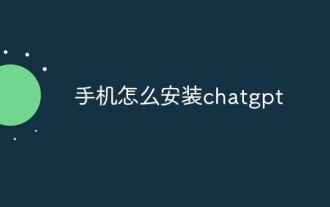
Installation steps: 1. Download the ChatGTP software from the ChatGTP official website or mobile store; 2. After opening it, in the settings interface, select the language as Chinese; 3. In the game interface, select human-machine game and set the Chinese spectrum; 4 . After starting, enter commands in the chat window to interact with the software.
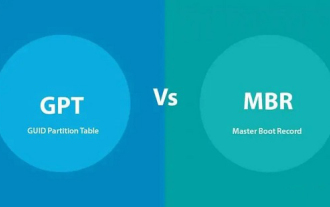
When partitioning their own systems, due to the different hard drives used by users, many users do not know whether the win10 partition format is gpt or mbr. For this reason, we have brought you a detailed introduction to help you understand the difference between the two. Win10 partition format gpt or mbr: Answer: If you are using a hard drive exceeding 3 TB, you can use gpt. gpt is more advanced than mbr, but mbr is still better in terms of compatibility. Of course, this can also be chosen according to the user's preferences. The difference between gpt and mbr: 1. Number of supported partitions: 1. MBR supports up to 4 primary partitions. 2. GPT is not limited by the number of partitions. 2. Supported hard drive size: 1. MBR only supports up to 2TB
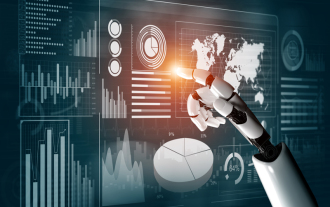
As artificial intelligence and machine learning technologies continue to develop, companies and organizations have begun to actively explore innovative strategies to leverage these technologies to enhance competitiveness. K8sGPT[2] is one of the most powerful tools in this field. It is a GPT model based on k8s, which combines the advantages of k8s orchestration with the excellent natural language processing capabilities of the GPT model. What is K8sGPT? Let’s look at an example first: According to the K8sGPT official website: K8sgpt is a tool designed for scanning, diagnosing and classifying kubernetes cluster problems. It integrates SRE experience into its analysis engine to provide the most relevant information. Through the application of artificial intelligence technology, K8sgpt continues to enrich its content and help users understand more quickly and accurately.
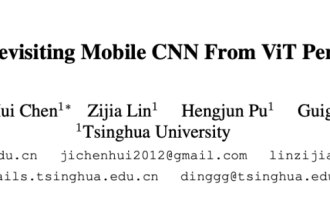
Paper address: https://arxiv.org/abs/2307.09283 Code address: https://github.com/THU-MIG/RepViTRepViT performs well in the mobile ViT architecture and shows significant advantages. Next, we explore the contributions of this study. It is mentioned in the article that lightweight ViTs generally perform better than lightweight CNNs on visual tasks, mainly due to their multi-head self-attention module (MSHA) that allows the model to learn global representations. However, the architectural differences between lightweight ViTs and lightweight CNNs have not been fully studied. In this study, the authors integrated lightweight ViTs into the effective
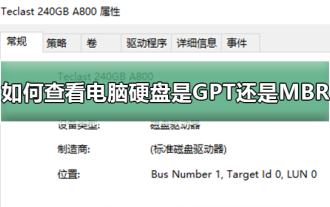
How to check whether a computer hard disk is a GPT partition or an MBR partition? When we use a computer hard disk, we need to distinguish between GPT and MBR. In fact, this checking method is very simple. Let's take a look with me. How to check whether the computer hard disk is GPT or MBR 1. Right-click 'Computer' on the desktop and click "Manage" 2. Find "Disk Management" in "Management" 3. Enter Disk Management to see the general status of our hard disk, then How to check the partition mode of my hard disk, right-click "Disk 0" and select "Properties" 4. Switch to the "Volume" tab in "Properties", then we can see the "Disk Partition Form" and you can see it as Problems related to MBR partition win10 disk How to convert MBR partition to GPT partition >
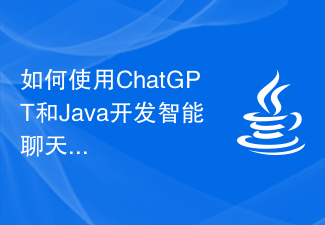
In this article, we will introduce how to develop intelligent chatbots using ChatGPT and Java, and provide some specific code examples. ChatGPT is the latest version of the Generative Pre-training Transformer developed by OpenAI, a neural network-based artificial intelligence technology that can understand natural language and generate human-like text. Using ChatGPT we can easily create adaptive chats
