


Interpretation of Microsoft's latest HuggingGPT paper, what did you learn?
Microsoft recently published a paper on HuggingGPT. Original address: HuggingGPT: Solving AI Tasks with ChatGPT and its Friends in Hugging Face[1]. This article is an interpretation of the paper.
HuggingGPT: Solving AI Tasks with ChatGPT and its Friends in Hugging Face Translated into Chinese: HuggingGPT: Solving AI Tasks with ChatGPT and its Friends in Hugging Face.
What are its friends? After reading the paper, it should be the large language model represented by GPT4 and various expert models. The expert model mentioned in this article is relative to the general model and is a model in a specific field, such as a model in the medical field, a model in the financial field, etc.
Hugging Face is an open source machine learning community and platform.
You can quickly understand the main content of the paper by answering the following questions.
- What is the idea behind HuggingGPT and how does it work?
- ##The idea behind HuggingGPT is to use a large language model (LLM) as the controller to manage AI models and solve complex AI tasks. HuggingGPT works by leveraging LLM’s strengths in understanding and reasoning to dissect user requests and decompose them into multiple subtasks. Then, based on the description of the expert model, HuggingGPT assigns the most suitable model to each task and integrates the results of different models. The workflow of HuggingGPT includes four stages: task planning, model selection, task execution and response generation. You can find more information on pages 4 and 16 of the PDF file.
- What is the idea behind HuggingGPT and how does it work?
- The idea behind HuggingGPT is to use a Large Language Model (LLM) Act as a controller to manage AI models and solve complex AI tasks. The working principle of HuggingGPT is to take advantage of LLM's advantages in understanding and reasoning to analyze user intentions and decompose tasks into multiple sub-tasks. Then, based on the description of the expert model, HuggingGPT assigns the most suitable model to each task and integrates the results of different models. The workflow of HuggingGPT includes four stages: task planning, model selection, task execution and response generation. You can find more information on pages 4 and 16 of the PDF file.
- How does HuggingGPT use language as a common interface to enhance AI models?
- HuggingGPT uses language as a common interface to enhance AI models by using Large language models (LLM) serve as controllers to manage AI models. LLM can understand and reason about users' natural language requests, and then decompose the task into multiple sub-tasks. Based on the description of the expert model, HuggingGPT assigns the most suitable model to each sub-task and integrates the results of different models. This approach enables HuggingGPT to cover complex AI tasks in many different modalities and domains, including language, vision, speech, and other challenging tasks. You can find more information on pages 1 and 16 of the PDF file.
- How does HuggingGPT use large language models to manage existing AI models?
- HuggingGPT uses large language models as interfaces to route user requests to Expert models effectively combine the language understanding capabilities of large language models with the expertise of other expert models. The large language model acts as the brain for planning and decision-making, while the small model acts as the executor of each specific task. This collaboration protocol between models provides new ways to design general AI models. (Page 3-4)
- What kind of complex AI tasks can HuggingGPT solve?
- HuggingGPT can solve languages, images, audio A wide range of tasks in various modalities such as video and video, including various forms of tasks such as detection, generation, classification and question answering. Examples of 24 tasks that HuggingGPT can solve include text classification, object detection, semantic segmentation, image generation, question answering, text-to-speech, and text-to-video. (Page 3)
- Can HuggingGPT be used with different types of AI models, or is it limited to specific models?
- HuggingGPT is not limited to specific models AI models or visual perception tasks. It can solve tasks in any modality or domain by organizing cooperation between models through large language models. Under the planning of large language models, task processes can be effectively specified and more complex problems can be solved. HuggingGPT takes a more open approach, assigning and organizing tasks according to model descriptions. (Page 4)
HuggingGPT’s workflow includes four stages:
- Task planning: Use ChatGPT to analyze user requests, understand their intentions, and dismantle them into a solvable task.
- Model Selection: To solve the planned tasks, ChatGPT selects AI models hosted on Hugging Face based on their descriptions.
- Task execution: Call and execute each selected model and return the results to ChatGPT.
- Generate response: Finally, use ChatGPT to integrate the predictions of all models and generate Response.
Reference link
[1] HuggingGPT: Solving AI Tasks with ChatGPT and its Friends in Hugging Face: https://arxiv.org/pdf/2104.06674.pdf
The above is the detailed content of Interpretation of Microsoft's latest HuggingGPT paper, what did you learn?. For more information, please follow other related articles on the PHP Chinese website!

Hot AI Tools

Undresser.AI Undress
AI-powered app for creating realistic nude photos

AI Clothes Remover
Online AI tool for removing clothes from photos.

Undress AI Tool
Undress images for free

Clothoff.io
AI clothes remover

Video Face Swap
Swap faces in any video effortlessly with our completely free AI face swap tool!

Hot Article

Hot Tools

Notepad++7.3.1
Easy-to-use and free code editor

SublimeText3 Chinese version
Chinese version, very easy to use

Zend Studio 13.0.1
Powerful PHP integrated development environment

Dreamweaver CS6
Visual web development tools

SublimeText3 Mac version
God-level code editing software (SublimeText3)

Hot Topics










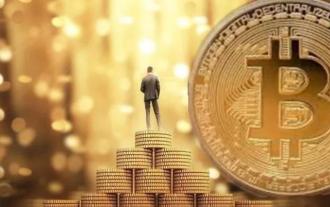
Recommended reliable digital currency trading platforms: 1. OKX, 2. Binance, 3. Coinbase, 4. Kraken, 5. Huobi, 6. KuCoin, 7. Bitfinex, 8. Gemini, 9. Bitstamp, 10. Poloniex, these platforms are known for their security, user experience and diverse functions, suitable for users at different levels of digital currency transactions
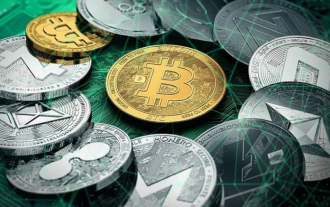
The top ten cryptocurrency trading platforms in the world include Binance, OKX, Gate.io, Coinbase, Kraken, Huobi Global, Bitfinex, Bittrex, KuCoin and Poloniex, all of which provide a variety of trading methods and powerful security measures.
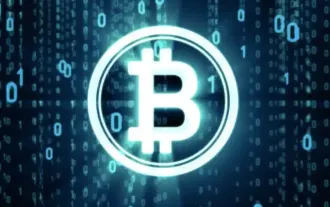
The top ten digital currency exchanges such as Binance, OKX, gate.io have improved their systems, efficient diversified transactions and strict security measures.
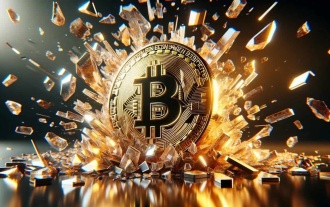
Bitcoin’s price ranges from $20,000 to $30,000. 1. Bitcoin’s price has fluctuated dramatically since 2009, reaching nearly $20,000 in 2017 and nearly $60,000 in 2021. 2. Prices are affected by factors such as market demand, supply, and macroeconomic environment. 3. Get real-time prices through exchanges, mobile apps and websites. 4. Bitcoin price is highly volatile, driven by market sentiment and external factors. 5. It has a certain relationship with traditional financial markets and is affected by global stock markets, the strength of the US dollar, etc. 6. The long-term trend is bullish, but risks need to be assessed with caution.
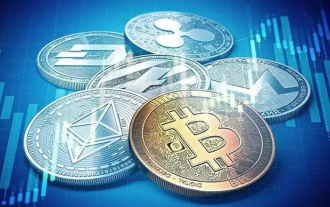
Currently ranked among the top ten virtual currency exchanges: 1. Binance, 2. OKX, 3. Gate.io, 4. Coin library, 5. Siren, 6. Huobi Global Station, 7. Bybit, 8. Kucoin, 9. Bitcoin, 10. bit stamp.
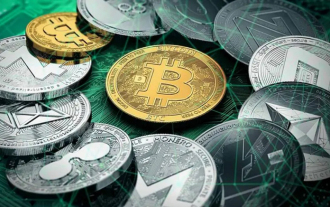
The top ten cryptocurrency exchanges in the world in 2025 include Binance, OKX, Gate.io, Coinbase, Kraken, Huobi, Bitfinex, KuCoin, Bittrex and Poloniex, all of which are known for their high trading volume and security.
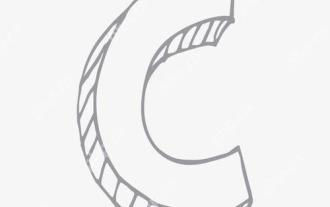
Measuring thread performance in C can use the timing tools, performance analysis tools, and custom timers in the standard library. 1. Use the library to measure execution time. 2. Use gprof for performance analysis. The steps include adding the -pg option during compilation, running the program to generate a gmon.out file, and generating a performance report. 3. Use Valgrind's Callgrind module to perform more detailed analysis. The steps include running the program to generate the callgrind.out file and viewing the results using kcachegrind. 4. Custom timers can flexibly measure the execution time of a specific code segment. These methods help to fully understand thread performance and optimize code.
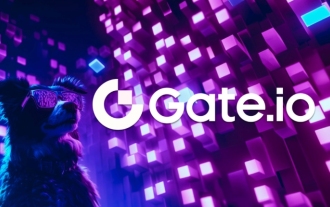
MeMebox 2.0 redefines crypto asset management through innovative architecture and performance breakthroughs. 1) It solves three major pain points: asset silos, income decay and paradox of security and convenience. 2) Through intelligent asset hubs, dynamic risk management and return enhancement engines, cross-chain transfer speed, average yield rate and security incident response speed are improved. 3) Provide users with asset visualization, policy automation and governance integration, realizing user value reconstruction. 4) Through ecological collaboration and compliance innovation, the overall effectiveness of the platform has been enhanced. 5) In the future, smart contract insurance pools, forecast market integration and AI-driven asset allocation will be launched to continue to lead the development of the industry.
