


A Chinese team successfully developed AI to predict suitable drugs for cancer patients, and the results were published in the Nature sub-journal
With just one AI, the clinical responses of 9,808 cancer patients to drugs can be fully predicted.
And the results are consistent with clinical observations.
This is the latest result CODE-AE (context-aware deconfounding autoencoder) brought by Lei Xie's team at the City University of New York.
It proposes a novel contextual autoencoding model that can predict the specific responses of different patients to drugs.
This will have a significant impact on new drug development and clinical trials.
You must know that under the traditional model, it takes nearly 10 years to develop, test, and fully market a new drug, and the funds consumed are unprecedentedly huge, easily reaching 1 billion U.S. dollars.
The cycle is so long because the reaction of new drugs in the human body is difficult to predict, and repeated trials are often required for testing.
If AI can use data to make predictions, it will significantly shorten the time to market for new drugs and reduce costs.
Currently, this research has been published in the Nature sub-journal "Nature Machine Intelligence".
Simply put, CODE-AE uses data from in vitro cell validation of new drugs to predict the response of the drug in the human body.
This avoids the dependence of AI model training on patient clinical data.
The biggest reason why AI has not been very effective in clinical response prediction in the past is that it is too difficult to collect massive and continuous clinical response data.
From a mechanism perspective, researchers divide drug biomarkers into source domain and target domain.
The source domain represents a different domain than the test sample, but has rich supervision information, which can be understood as in vitro cell verification data.
The target domain is the domain where the test sample is located. It has no labels or only a small number of labels, that is, patient data.
Map data features from different fields to the same feature space so that their distances in this space are as close as possible.
So the objective function trained on the source domain in the feature space can be transferred to the target domain to improve the accuracy in the target domain.
In the context of this research, the source domain and the target domain are both data characteristics of drug biomarkers, that is, data characteristics of drug targets.
Looking specifically at the model framework, it is mainly divided into three parts: pre-training, fine-tuning and inference.
Pre-training mainly uses self-supervised learning to build a feature encoding module to map the unlabeled gene expression profiles of in vitro cell data and patient data into the embedding space. In this way, some confounding factors can be eliminated and the latent distribution of the two data can be consistent to eliminate systematic bias.
The fine-tuning stage is to add a supervised model on the basis of pre-training and use labeled in vitro cell data for training.
Finally, in the inference stage, the patients obtained from pre-training are first disambiguated and embedded, and then the tuned model is used to predict the patient's response to the drug.
In this mode, CODE-AE has two characteristics.
First, it can extract common biological signals and private representations in incoherent samples, thereby eliminating interference caused by different data patterns.
Second, after separating the drug response signal and confounding factors, local alignment can also be achieved.
To summarize, CODE-AE can be understood as the process of selecting unique features in the incoherent data pattern embedding space of labeled and unlabeled data.
To demonstrate the effectiveness of the model, the researchers predicted the drug suitability of 9,808 cancer patients.
If the site results predicted by the model for the patient's condition are related to the drug target he uses, it proves that the prediction is correct.
The researchers then divided the patients into 100 clusters and the 59 drugs into 30 clusters.
Through this analysis method, patients with similar drug response profiles can be grouped together.
Here, we take the clustering of patients with lung squamous cell carcinoma (LSCC) and non-small cell lung cancer (NSCLC) as an example.
Among the 59 drugs, the most sensitive drugs for LSCC are gefitinib, AICAR and gemcitabine.
The targets of gefitinib and AICAR are both epidermal growth factor receptors (EGFR), and gemcitabine is often used to treat non-small cell lung cancer without EGFR mutations.
The paper stated that, consistent with the action modes of these drugs, CODE-AE found that patients using gefitinib and AICAR had similar drug response profiles.
In other words, CODE-AE has discovered the correct target for patient treatment, that is, it can predict applicable drugs.
The above research team is from the City University of New York.
The corresponding author is Lei Xie, who graduated from the University of Science and Technology of China in polymer physics.
He graduated with a master's degree in computer science from Rutgers University; his doctorate was also from Rutgers University, but with a degree in chemistry.
It is understood that the next step of the research team will be to develop the CODE-AE prediction function for the concentration and metabolism of the clinical response of new drugs.
The researchers said that the AI model may also be adapted to predict the side effects of drugs on the human body.
It is worth mentioning that the Nature sub-journal "Nature Machine Intelligence" specializes in interdisciplinary applied research in artificial intelligence and life sciences, and the average number of papers included every year is about 60.
Paper address: https://www.nature.com/articles/s42256-022-00541-0
Reference link: https://phys.org/news/2022-10 -ai-accurately-human-response-drug.html
The above is the detailed content of A Chinese team successfully developed AI to predict suitable drugs for cancer patients, and the results were published in the Nature sub-journal. For more information, please follow other related articles on the PHP Chinese website!

Hot AI Tools

Undresser.AI Undress
AI-powered app for creating realistic nude photos

AI Clothes Remover
Online AI tool for removing clothes from photos.

Undress AI Tool
Undress images for free

Clothoff.io
AI clothes remover

Video Face Swap
Swap faces in any video effortlessly with our completely free AI face swap tool!

Hot Article

Hot Tools

Notepad++7.3.1
Easy-to-use and free code editor

SublimeText3 Chinese version
Chinese version, very easy to use

Zend Studio 13.0.1
Powerful PHP integrated development environment

Dreamweaver CS6
Visual web development tools

SublimeText3 Mac version
God-level code editing software (SublimeText3)

Hot Topics
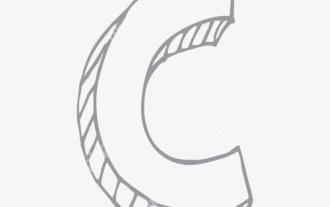
Using the chrono library in C can allow you to control time and time intervals more accurately. Let's explore the charm of this library. C's chrono library is part of the standard library, which provides a modern way to deal with time and time intervals. For programmers who have suffered from time.h and ctime, chrono is undoubtedly a boon. It not only improves the readability and maintainability of the code, but also provides higher accuracy and flexibility. Let's start with the basics. The chrono library mainly includes the following key components: std::chrono::system_clock: represents the system clock, used to obtain the current time. std::chron
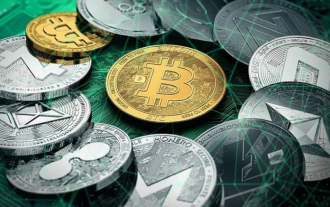
The top ten cryptocurrency trading platforms in the world include Binance, OKX, Gate.io, Coinbase, Kraken, Huobi Global, Bitfinex, Bittrex, KuCoin and Poloniex, all of which provide a variety of trading methods and powerful security measures.
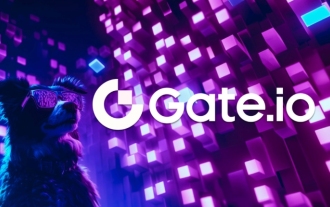
MeMebox 2.0 redefines crypto asset management through innovative architecture and performance breakthroughs. 1) It solves three major pain points: asset silos, income decay and paradox of security and convenience. 2) Through intelligent asset hubs, dynamic risk management and return enhancement engines, cross-chain transfer speed, average yield rate and security incident response speed are improved. 3) Provide users with asset visualization, policy automation and governance integration, realizing user value reconstruction. 4) Through ecological collaboration and compliance innovation, the overall effectiveness of the platform has been enhanced. 5) In the future, smart contract insurance pools, forecast market integration and AI-driven asset allocation will be launched to continue to lead the development of the industry.
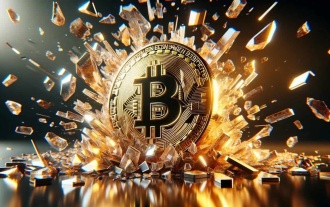
Bitcoin’s price ranges from $20,000 to $30,000. 1. Bitcoin’s price has fluctuated dramatically since 2009, reaching nearly $20,000 in 2017 and nearly $60,000 in 2021. 2. Prices are affected by factors such as market demand, supply, and macroeconomic environment. 3. Get real-time prices through exchanges, mobile apps and websites. 4. Bitcoin price is highly volatile, driven by market sentiment and external factors. 5. It has a certain relationship with traditional financial markets and is affected by global stock markets, the strength of the US dollar, etc. 6. The long-term trend is bullish, but risks need to be assessed with caution.
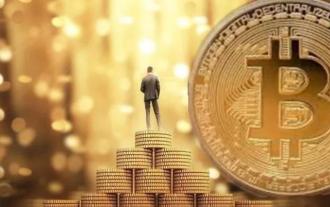
Recommended reliable digital currency trading platforms: 1. OKX, 2. Binance, 3. Coinbase, 4. Kraken, 5. Huobi, 6. KuCoin, 7. Bitfinex, 8. Gemini, 9. Bitstamp, 10. Poloniex, these platforms are known for their security, user experience and diverse functions, suitable for users at different levels of digital currency transactions
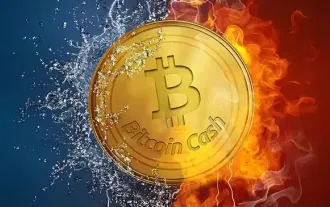
Bitcoin’s price fluctuations today are affected by many factors such as macroeconomics, policies, and market sentiment. Investors need to pay attention to technical and fundamental analysis to make informed decisions.
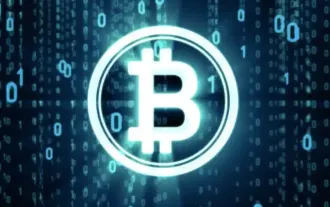
The top ten digital currency exchanges such as Binance, OKX, gate.io have improved their systems, efficient diversified transactions and strict security measures.
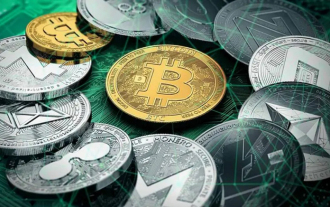
The top ten cryptocurrency exchanges in the world in 2025 include Binance, OKX, Gate.io, Coinbase, Kraken, Huobi, Bitfinex, KuCoin, Bittrex and Poloniex, all of which are known for their high trading volume and security.
