


Sudden! Li Feifei's apprentice Karpathy resigned, will Tesla's autonomous driving be in doubt?
Just now, Tesla lost another technical expert!
Andrej Karpathy, senior director of Tesla AI and head of the autonomous driving vision team, tweeted to announce that he will leave Tesla.
Previously, he had worked for Tesla for 5 years and reported directly to the big boss Musk. Among Tesla’s senior executives, he was definitely one of the top He is a veteran.
According to reports, Li Feifei’s apprentice has been on vacation for several months. He had previously said that he would return to Tesla after finishing his vacation in the near future, and the results were announced directly. has resigned.
Karpathy said:
#"I am very happy to help Tesla achieve many goals in the past five years. The decision is actually a difficult one. In the past five years, autonomous driving has completed its "graduation", starting from stumbling to find a way and driving on the streets of the city. I look forward to the future of a more powerful autonomous driving team that can continue to be glorious."
Regarding his future plans after leaving his job, he said:
"I don’t have any specific plans for the future. I may return to my long-term passion." fields, such as AI technology work, open source and education, etc."
No matter how good the technology is, it can’t keep up with Boss Ma’s bragging
This time Karpathy’s resignation is It's a critical moment for Tesla's autonomous driving department, or "trouble-ridden" is more appropriate.
Not long ago, the U.S. National Highway and Safety Administration (NHTSA) was investigating Tesla’s autonomous driving technology. Tesla's self-driving software has reportedly been linked to more than 200 crashes since July last year.
Since June 2021, Tesla vehicles have accounted for nearly 70% of reported crashes involving advanced driver assistance systems.
Two weeks ago, Tesla’s autonomous driving department laid off more than 200 employees and closed its entire office in San Mateo, California. The office's data annotation team is helping improve driver assistance technology.
Looking back at Tesla’s bumpy ride on autonomous driving, one has to lament that Andrej Karpathy’s job over the past five years has not been easy.
# Of course, a lot of trouble may have been caused by his loud-mouthed boss Musk.
When it comes to autonomous driving that Tesla is proud of, Academician Ma has always boasted and never drafted it.
As early as 2016, Musk promised Tesla fans that by the end of 2017, a self-driving car that could travel from Los Angeles to New York without touching the steering wheel would be available.
By 2019, Musk once again promised investors that by the end of 2020, Tesla would have 1 million "robot taxis" ready to hit the road. (robotaxi), defrauding Tesla of billions of dollars.
Also in 2019, Musk told investors, "Sometimes what I say is not on time, but I can finish it in the end."
The result is that as of 2022, Tesla has not yet come up with a self-driving car demonstration from "Los Angeles to New York". As for robotaxi, Musk really mentioned it a few months ago. This time It has been pushed back to 2024.
Now Tesla no longer pursues the big goal of "Los Angeles to New York", but instead develops driving assistance functions, such as traffic cruise control, lane keeping and automatic driving. Navigation and other "small goals".
#Tesla gave these features a name called fully autonomous driving "beta version."
It is said to be fully automated, but it still requires human drivers to keep their attention on the road, hold the steering wheel with both hands, and be ready to take over the driving task at any time.
Seeing that the goal of truly "fully autonomous driving" is far away, maybe Andrej Karpathy is just tired.
Li Feifei is a master disciple and a technical master who "powers with love"
Don't underestimate this Andrej Karpathy, he is an absolute man A master of buckle technology.
He first received a bachelor's degree in computer science and physics from the University of Toronto in 2009, and then obtained a master's degree from the University of British Columbia in 2011. His research was in physical analog digital controller.
When he was studying for his PhD at Stanford, Karpathy and his mentor Li Feifei jointly studied convolutional/recurrent neural network architecture and its application in computer vision, natural language processing and other intersection fields. .
The master and apprentice also designed a new course (CS231n) on Convolutional Neural Networks for Visual Recognition at Stanford University, with Karpathy personally serving as the first lecturer.
And this is the first deep learning course offered by Stanford! The number of students has also grown from 150 in 2015 to 330 in 2016 and to 750 in 2017.
Now, Stanford University’s CS231n has become a must-see basic course for deep learning beginners, especially those in the CV direction. In addition to the "star power" of the famous Li Feifei, Karpathy has personally done everything from course design to giving lectures and has made a lot of contributions.
While he was in school, he also went to Google for internships - twice, the kind that went abroad, and participated in deep learning and computer vision projects at Google.
#In 2015, he went to DeepMind for another internship in the deep reinforcement learning team.
After graduation, Karpathy joined OpenAI in 2016 as a research scientist, responsible for deep learning in computer vision, generative modeling and reinforcement learning.
"Stanford doctoral student, Li Feifei's disciple, former OpenAI researcher and one of the founders", these titles have also made him famous in Silicon Valley.
Sure enough, after only working at OpenAI for a year and a half, he was poached by Musk to Tesla and replaced former Apple executive Chris Lattner as Tesla Director of AI and Autopilot Vision.
Since Karpathy likes to study neural networks very much, he writes a lot of technical blogs in his spare time and develops multiple deep learning libraries in Javascript (such as ConvNetJS , RecurrentJS, REINFORCEjs, t-sneJS).
It has even been jokingly called ImageNet’s “human flesh reference.”
In addition, whenever he has time, he will maintain his arxiv-sanity of "powering with love", which can search and organize nearly 100,000 articles in the past 6 years A website about machine learning arXiv papers.
Academician Ma sent him off in person. Netizens: Is Tesla’s autopilot bad?
Regarding Karparthy’s departure, industry leaders and peers also tweeted their blessings.
Former boss Musk sent Karpathy farewell under this tweet, and his words were very polite:
"Thank you for Tesla Thank you for everything you have done! It is an honor to work with you."
It is probably rare that Academician Ma can be so polite to senior executives who have resigned. It seems that I really have feelings.
François Chollet, a well-known Google researcher and founder of the Keras framework, also tweeted his blessings and wished him an early start in a new stage of his career.
Netizen Ryan Huber said that Karpathy was praised by Musk just because Musk didn’t want him to fall into the enemy’s camp. After all, this man is an opponent of Tesla’s fully automated Driving someone who knows everything.
#Some netizens also questioned whether fully autonomous driving (FSD) can be realized without him?
As early as March this year, Karparthy himself tweeted that after working at Tesla for five years, he finally took the time to take a short vacation, which lasted about 4 months.
At that time, some netizens speculated whether this long vacation would be a signal that he was about to resign. After all, there were quite a few executives who took leave before leaving. Looking at it now, it turns out that... Where will Tesla's self-driving business go? As Tesla’s “cash cow” department, who will Musk choose as Karparthy’s successor?
The above is the detailed content of Sudden! Li Feifei's apprentice Karpathy resigned, will Tesla's autonomous driving be in doubt?. For more information, please follow other related articles on the PHP Chinese website!

Hot AI Tools

Undresser.AI Undress
AI-powered app for creating realistic nude photos

AI Clothes Remover
Online AI tool for removing clothes from photos.

Undress AI Tool
Undress images for free

Clothoff.io
AI clothes remover

Video Face Swap
Swap faces in any video effortlessly with our completely free AI face swap tool!

Hot Article

Hot Tools

Notepad++7.3.1
Easy-to-use and free code editor

SublimeText3 Chinese version
Chinese version, very easy to use

Zend Studio 13.0.1
Powerful PHP integrated development environment

Dreamweaver CS6
Visual web development tools

SublimeText3 Mac version
God-level code editing software (SublimeText3)

Hot Topics










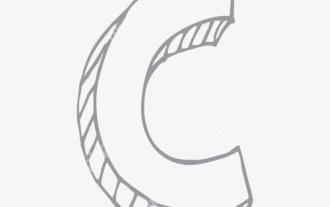
Using the chrono library in C can allow you to control time and time intervals more accurately. Let's explore the charm of this library. C's chrono library is part of the standard library, which provides a modern way to deal with time and time intervals. For programmers who have suffered from time.h and ctime, chrono is undoubtedly a boon. It not only improves the readability and maintainability of the code, but also provides higher accuracy and flexibility. Let's start with the basics. The chrono library mainly includes the following key components: std::chrono::system_clock: represents the system clock, used to obtain the current time. std::chron
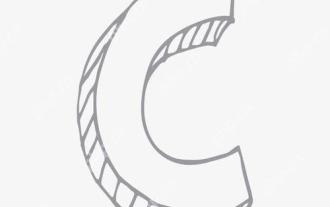
Handling high DPI display in C can be achieved through the following steps: 1) Understand DPI and scaling, use the operating system API to obtain DPI information and adjust the graphics output; 2) Handle cross-platform compatibility, use cross-platform graphics libraries such as SDL or Qt; 3) Perform performance optimization, improve performance through cache, hardware acceleration, and dynamic adjustment of the details level; 4) Solve common problems, such as blurred text and interface elements are too small, and solve by correctly applying DPI scaling.
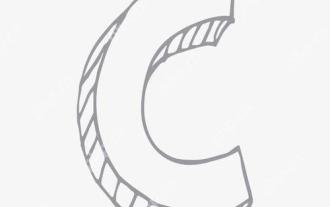
DMA in C refers to DirectMemoryAccess, a direct memory access technology, allowing hardware devices to directly transmit data to memory without CPU intervention. 1) DMA operation is highly dependent on hardware devices and drivers, and the implementation method varies from system to system. 2) Direct access to memory may bring security risks, and the correctness and security of the code must be ensured. 3) DMA can improve performance, but improper use may lead to degradation of system performance. Through practice and learning, we can master the skills of using DMA and maximize its effectiveness in scenarios such as high-speed data transmission and real-time signal processing.
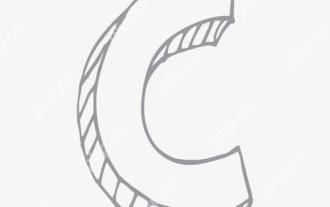
C performs well in real-time operating system (RTOS) programming, providing efficient execution efficiency and precise time management. 1) C Meet the needs of RTOS through direct operation of hardware resources and efficient memory management. 2) Using object-oriented features, C can design a flexible task scheduling system. 3) C supports efficient interrupt processing, but dynamic memory allocation and exception processing must be avoided to ensure real-time. 4) Template programming and inline functions help in performance optimization. 5) In practical applications, C can be used to implement an efficient logging system.
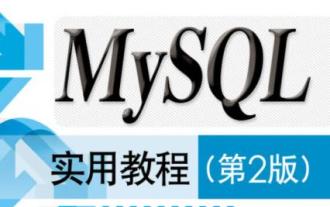
In MySQL, add fields using ALTERTABLEtable_nameADDCOLUMNnew_columnVARCHAR(255)AFTERexisting_column, delete fields using ALTERTABLEtable_nameDROPCOLUMNcolumn_to_drop. When adding fields, you need to specify a location to optimize query performance and data structure; before deleting fields, you need to confirm that the operation is irreversible; modifying table structure using online DDL, backup data, test environment, and low-load time periods is performance optimization and best practice.
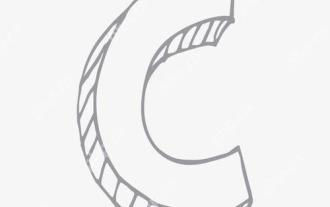
Measuring thread performance in C can use the timing tools, performance analysis tools, and custom timers in the standard library. 1. Use the library to measure execution time. 2. Use gprof for performance analysis. The steps include adding the -pg option during compilation, running the program to generate a gmon.out file, and generating a performance report. 3. Use Valgrind's Callgrind module to perform more detailed analysis. The steps include running the program to generate the callgrind.out file and viewing the results using kcachegrind. 4. Custom timers can flexibly measure the execution time of a specific code segment. These methods help to fully understand thread performance and optimize code.
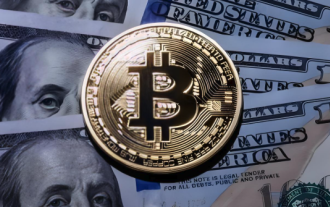
The built-in quantization tools on the exchange include: 1. Binance: Provides Binance Futures quantitative module, low handling fees, and supports AI-assisted transactions. 2. OKX (Ouyi): Supports multi-account management and intelligent order routing, and provides institutional-level risk control. The independent quantitative strategy platforms include: 3. 3Commas: drag-and-drop strategy generator, suitable for multi-platform hedging arbitrage. 4. Quadency: Professional-level algorithm strategy library, supporting customized risk thresholds. 5. Pionex: Built-in 16 preset strategy, low transaction fee. Vertical domain tools include: 6. Cryptohopper: cloud-based quantitative platform, supporting 150 technical indicators. 7. Bitsgap:
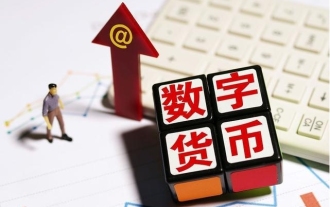
The top 10 digital virtual currency trading platforms are: 1. Binance, 2. OKX, 3. Coinbase, 4. Kraken, 5. Huobi Global, 6. Bitfinex, 7. KuCoin, 8. Gemini, 9. Bitstamp, 10. Bittrex. These platforms all provide high security and a variety of trading options, suitable for different user needs.
