OpenAI and Microsoft Sentinel Part 2: Explaining the Analysis Rules
Welcome back to our series about OpenAI and Microsoft Sentinel! Today we’ll explore another use case for OpenAI’s popular language models and take a look at the Sentinel REST API. If you don't have a Microsoft Sentinel instance yet, you can create one using a free Azure account and follow the Getting Started with Sentinel quickstart. You will also need a personal OpenAI account with an API key. get ready? let's start!
One of the tasks security practitioners face is how to quickly use analysis rules, queries, and definitions to understand what triggered an alert. For example, here is an example of a relatively short Microsoft Sentinel analysis rule written in Kusto Query Language (KQL):
Experienced KQL operators will have no difficulty Parse it out, but it still takes some time to mentally map out the keywords, log sources, actions, and event logic. People who have never used KQL may need more time to understand what this rule is designed to detect. Luckily, we have a friend who is really good at reading code and interpreting it in natural language – OpenAI’s GPT3!
GPT3 engines like DaVinci are very good at interpreting code in natural language and have extensive training in Microsoft Sentinel KQL syntax and usage. Even better, Sentinel has a built-in OpenAI connector that allows us to integrate GPT3 models into automated Sentinel playbooks! We can use this connector to add comments to Sentinel events that describe analysis rules. This will be a simple Logic App with a linear flow of operations:
# Let’s walk through Logic App, starting with triggers. We use a Microsoft Sentinel event trigger for this playbook so that we can use the Sentinel connector to extract all relevant analytics rule IDs from the event. We will use the Sentinel REST API to find the rule query text using the rule ID, which we can pass to the AI model in the text completion prompt. Finally, we will add the output of the AI model to the event as a comment.
Our first action is the "For each" logic block that acts on Sentinel's "Event-related analysis rule ID item":
Next , we need to use the Sentinel REST API to request the scheduled alert rule itself. This API endpoint is documented here: https://learn.microsoft.com/en-us/rest/api/securityinsights/stable/alert-rules/get ?tabs= HTTP . If you haven't used the Sentinel API before, you can click the green "Try It Out" button next to the code block to preview the actual request using your credentials. This is a great way to explore the API! In our case, the "Get - Alert Rules" request looks like this:
GET https://management.azure.com/subscriptions/{subscriptionId}/resourceGroups/{resourceGroupName}/providers/Microsoft.OperationalInsights/workspaces/{workspaceName}/providers/Microsoft.SecurityInsights/alertRules/{ruleId}?api-version=2022-11-01
We can make this API call using the "HTTP" action in our logic app. Fortunately, the event-related analysis rule ID item we just added to the "For each" logic block comes with almost all of the parameters pre-populated. We just need to prepend the API domain with the version specification to the end - all parameters for subscriptionID, resourceGroupName, workspaceName and ruleId will come from our dynamic content object. The actual text of my URI block is as follows:
https://management.azure.com@{items('For_each_related_Analytics_Rule_ID')}?api-version=2022-11-01
We also need to configure authentication options for HTTP operations - I am using managed identity for my logic app. The completed action block looks like this:
Now that we have the alert rule, we just need to parse the rule text so that we can pass it to GPT3. Let's use the Parse JSON action, provide it with the body content from the HTTP step, and define the schema to match the expected output of this API call. The easiest way to generate the schema is to upload a sample payload, but we don't need to include all the properties we're not interested in. I have shortened the architecture to look like this:
{"type": "object","properties": {"id": {"type": "string"},"type": {"type": "string"},"kind": {"type": "string"},"properties": {"type": "object","properties": {"severity": {"type": "string"},"query": {"type": "string"},"tactics": {},"techniques": {},"displayName": {"type": "string"},"description": {"type": "string"},"lastModifiedUtc": {"type": "string"}}}}}
So far our logic blocks look like this:
Now it’s time to get the AI involved Come in! Select "GPT3 Completes your prompt" from the OpenAI connector and write your prompt using the "query" dynamic content object from the previous Parse JSON step. We will use the latest Resolve engine and keep most of the default parameters. Our query did not show a significant difference between high and low temperature values, but we did want to increase the "max tokens" parameter to give the da Vinci model more room for long answers. The completed action should be like this:
The final step in our playbook is to add a comment to the event using the resulting text from GPT3. If you want to add an event task instead, just select the Sentinel action. Add an "Event ARM ID" dynamic content object and compose the comment message using the "Text (Completion Text)" output by the GPT3 action. The Logic App Designer automatically wraps your comment actions in a "For each" logic block. The finished comment action should look something like this:
Save your logic app and let’s try it out in an event! If all went well, our Logic App run history will show successful completion. If any problems arise, you can check the exact input and output details of each step - an invaluable troubleshooting tool! In our case it was always a green check mark:
Success! The playbook adds commentary to the event, saving our overworked security analysts a few minutes.
The above is the detailed content of OpenAI and Microsoft Sentinel Part 2: Explaining the Analysis Rules. For more information, please follow other related articles on the PHP Chinese website!

Hot AI Tools

Undresser.AI Undress
AI-powered app for creating realistic nude photos

AI Clothes Remover
Online AI tool for removing clothes from photos.

Undress AI Tool
Undress images for free

Clothoff.io
AI clothes remover

Video Face Swap
Swap faces in any video effortlessly with our completely free AI face swap tool!

Hot Article

Hot Tools

Notepad++7.3.1
Easy-to-use and free code editor

SublimeText3 Chinese version
Chinese version, very easy to use

Zend Studio 13.0.1
Powerful PHP integrated development environment

Dreamweaver CS6
Visual web development tools

SublimeText3 Mac version
God-level code editing software (SublimeText3)

Hot Topics










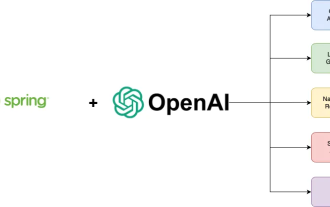
In 2023, AI technology has become a hot topic and has a huge impact on various industries, especially in the programming field. People are increasingly aware of the importance of AI technology, and the Spring community is no exception. With the continuous advancement of GenAI (General Artificial Intelligence) technology, it has become crucial and urgent to simplify the creation of applications with AI functions. Against this background, "SpringAI" emerged, aiming to simplify the process of developing AI functional applications, making it simple and intuitive and avoiding unnecessary complexity. Through "SpringAI", developers can more easily build applications with AI functions, making them easier to use and operate.
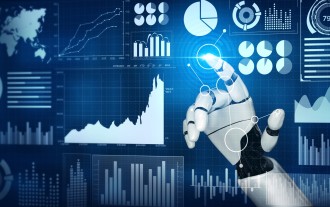
OpenAI recently announced the launch of their latest generation embedding model embeddingv3, which they claim is the most performant embedding model with higher multi-language performance. This batch of models is divided into two types: the smaller text-embeddings-3-small and the more powerful and larger text-embeddings-3-large. Little information is disclosed about how these models are designed and trained, and the models are only accessible through paid APIs. So there have been many open source embedding models. But how do these open source models compare with the OpenAI closed source model? This article will empirically compare the performance of these new models with open source models. We plan to create a data
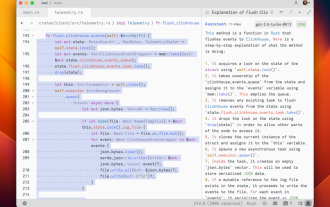
Author丨Compiled by TimAnderson丨Produced by Noah|51CTO Technology Stack (WeChat ID: blog51cto) The Zed editor project is still in the pre-release stage and has been open sourced under AGPL, GPL and Apache licenses. The editor features high performance and multiple AI-assisted options, but is currently only available on the Mac platform. Nathan Sobo explained in a post that in the Zed project's code base on GitHub, the editor part is licensed under the GPL, the server-side components are licensed under the AGPL, and the GPUI (GPU Accelerated User) The interface) part adopts the Apache2.0 license. GPUI is a product developed by the Zed team
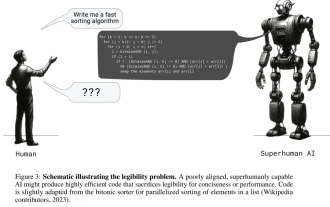
If the answer given by the AI model is incomprehensible at all, would you dare to use it? As machine learning systems are used in more important areas, it becomes increasingly important to demonstrate why we can trust their output, and when not to trust them. One possible way to gain trust in the output of a complex system is to require the system to produce an interpretation of its output that is readable to a human or another trusted system, that is, fully understandable to the point that any possible errors can be found. For example, to build trust in the judicial system, we require courts to provide clear and readable written opinions that explain and support their decisions. For large language models, we can also adopt a similar approach. However, when taking this approach, ensure that the language model generates
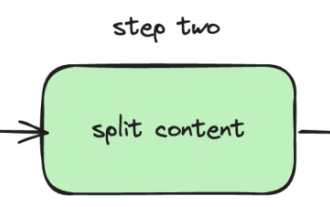
Ollama is a super practical tool that allows you to easily run open source models such as Llama2, Mistral, and Gemma locally. In this article, I will introduce how to use Ollama to vectorize text. If you have not installed Ollama locally, you can read this article. In this article we will use the nomic-embed-text[2] model. It is a text encoder that outperforms OpenAI text-embedding-ada-002 and text-embedding-3-small on short context and long context tasks. Start the nomic-embed-text service when you have successfully installed o
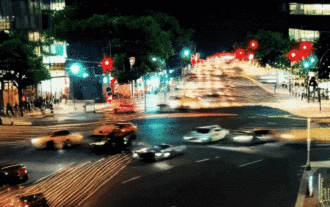
Not long ago, OpenAISora quickly became popular with its amazing video generation effects. It stood out among the crowd of literary video models and became the focus of global attention. Following the launch of the Sora training inference reproduction process with a 46% cost reduction 2 weeks ago, the Colossal-AI team has fully open sourced the world's first Sora-like architecture video generation model "Open-Sora1.0", covering the entire training process, including data processing, all training details and model weights, and join hands with global AI enthusiasts to promote a new era of video creation. For a sneak peek, let’s take a look at a video of a bustling city generated by the “Open-Sora1.0” model released by the Colossal-AI team. Open-Sora1.0
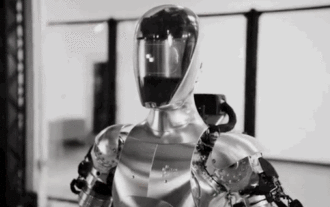
Microsoft and OpenAI were revealed to be investing large sums of money into a humanoid robot startup at the beginning of the year. Among them, Microsoft plans to invest US$95 million, and OpenAI will invest US$5 million. According to Bloomberg, the company is expected to raise a total of US$500 million in this round, and its pre-money valuation may reach US$1.9 billion. What attracts them? Let’s take a look at this company’s robotics achievements first. This robot is all silver and black, and its appearance resembles the image of a robot in a Hollywood science fiction blockbuster: Now, he is putting a coffee capsule into the coffee machine: If it is not placed correctly, it will adjust itself without any human remote control: However, After a while, a cup of coffee can be taken away and enjoyed: Do you have any family members who have recognized it? Yes, this robot was created some time ago.
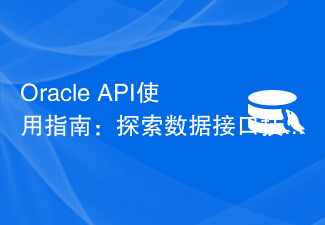
Oracle is a world-renowned database management system provider, and its API (Application Programming Interface) is a powerful tool that helps developers easily interact and integrate with Oracle databases. In this article, we will delve into the Oracle API usage guide, show readers how to utilize data interface technology during the development process, and provide specific code examples. 1.Oracle