AI ban on smoking is okay! Smoking recognition + face recognition
Hello, everyone.
Today I will share with you a smoking recognition and face recognition project. Many public places, production sites and schools have bans on smoking. It is still necessary to implement a ban on smoking and let AI automatically identify smoking behavior and identify who is smoking.
Use the target detection algorithm to determine smoking behavior, extract the face of the smoker, and use the face recognition algorithm to determine who is smoking. The idea is relatively simple, but the details are still a little troublesome.
The training data and source code used in the project have been packaged. It’s still the same as before, get it in the comment section.
1. Detecting cigarettes
I used 5k pieces of labeled smoking data as training data
and placed them in the dataset directory.
Train YOLOv5 target detection model.
First step, copy data/coco128.yaml to smoke.yaml, and modify the data set directory and category configuration information
path: ../dataset/smoke # dataset root dir train: images/train# train images (relative to 'path') 128 images val: images/test# val images (relative to 'path') 128 images test:# test images (optional) # Classes names: 0: smoke
Second step, copy ./models/yolov5s.yaml to smoke.yaml, modify nc
nc: 1# number of classes
The third step is to download the yolov5s.pt pre-trained model and place it in the {yolov5 directory}/weights directory
Execute the following command to train.
python ./train.py --data ./data/smoke.yaml --cfg ./models/smoke.yaml --weights ./weights/yolov5s.pt --batch-size 30 --epochs 120 --workers 8 --name smoke --project smoke_s
After the training is completed, you can see the following output:
The call is just fine.
After the training is completed, the best.pt position can be found and used later for cigarette detection.
model = torch.hub.load('../28_people_counting/yolov5', 'custom', './weights/ciga.pt', source='local') results = self.model(img[:, :, ::-1]) pd = results.pandas().xyxy[0] ciga_pd = pd[pd['class'] == 0]
After being able to identify cigarettes, we still need to determine whether we are currently smoking.
You can use the cigarette detection frame and the mouth detection frame to calculate the IOU to determine. To put it bluntly, it is to determine whether the two frames intersect. If so, it is considered that you are currently smoking.
Mouth detection frame, using facial key points to identify.
2. Face recognition
There are many mature models for face recognition algorithms. We don’t need to train them ourselves, we can just adjust the database directly.
I am using the dlib library here, which can identify 68 key points on a face and extract facial features based on these 68 key points.
face_detector = dlib.get_frontal_face_detector() face_sp = dlib.shape_predictor('./weights/shape_predictor_68_face_landmarks.dat') dets = face_detector(img, 1) face_list = [] for face in dets: l, t, r, b = face.left(), face.top(), face.right(), face.bottom() face_shape = face_sp(img, face)
face_detectorcan detect faces and return face detection frames. face_sp is based on face detection frames and identifies 68 key points of faces.
From these 68 key points, we can obtain the mouth detection frame to determine whether you are smoking.
Finally, we still hope to use face recognition algorithms to identify who is smoking.
The first step is to extract facial features
face_feature_model = dlib.face_recognition_model_v1('./weights/dlib_face_recognition_resnet_model_v1.dat') face_descriptor = face_feature_model.compute_face_descriptor(img, face_shape)
face_descriptorCalculate a feature vector for each face based on the position and distance between the 68 key points of the face. This principle is similar to the word2vec we shared before or mapping videos to N-dimensional vectors.
The second step is to enter the existing faces into the face database. I prepared 3 smoking behaviors in movies and TV series
Cut faces from the videos, vectorize them, and write them into the face database (replaced with files)
The third step, after smoking occurs, we can crop out the smoker’s face, calculate the face vector, compare it with the features of the face database, and find the best Similar faces, return the corresponding name
def find_face_name(self, face_feat): """ 人脸识别,计算吸烟者名称 :param face_feat: :return: """ cur_face_feature = np.asarray(face_feat, dtype=np.float64).reshape((1, -1)) # 计算两个向量(两张脸)余弦相似度 distances = np.linalg.norm((cur_face_feature - self.face_feats), axis=1) min_dist_index = np.argmin(distances) min_dist = distances[min_dist_index] if min_dist < 0.3: return self.face_name_list[min_dist_index] else: return '未知'
There are many areas where this project can be expanded, for example: the video I provided only has a single face, and it will definitely be used in actual monitoring. It’s multiple faces. At this time, the MOT algorithm can be used to track pedestrians, and then each person can be individually identified for smoking
Also, a separate statistical area can be created to save the identified smoking behaviors and use them as evidence for warnings and punishments .
The above is the detailed content of AI ban on smoking is okay! Smoking recognition + face recognition. For more information, please follow other related articles on the PHP Chinese website!

Hot AI Tools

Undresser.AI Undress
AI-powered app for creating realistic nude photos

AI Clothes Remover
Online AI tool for removing clothes from photos.

Undress AI Tool
Undress images for free

Clothoff.io
AI clothes remover

Video Face Swap
Swap faces in any video effortlessly with our completely free AI face swap tool!

Hot Article

Hot Tools

Notepad++7.3.1
Easy-to-use and free code editor

SublimeText3 Chinese version
Chinese version, very easy to use

Zend Studio 13.0.1
Powerful PHP integrated development environment

Dreamweaver CS6
Visual web development tools

SublimeText3 Mac version
God-level code editing software (SublimeText3)

Hot Topics
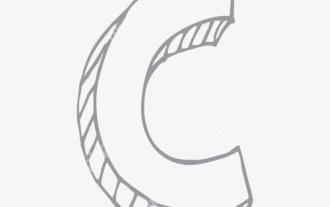
Using the chrono library in C can allow you to control time and time intervals more accurately. Let's explore the charm of this library. C's chrono library is part of the standard library, which provides a modern way to deal with time and time intervals. For programmers who have suffered from time.h and ctime, chrono is undoubtedly a boon. It not only improves the readability and maintainability of the code, but also provides higher accuracy and flexibility. Let's start with the basics. The chrono library mainly includes the following key components: std::chrono::system_clock: represents the system clock, used to obtain the current time. std::chron
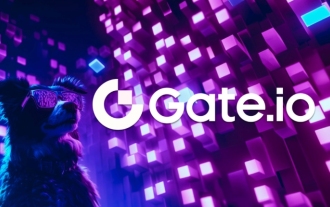
MeMebox 2.0 redefines crypto asset management through innovative architecture and performance breakthroughs. 1) It solves three major pain points: asset silos, income decay and paradox of security and convenience. 2) Through intelligent asset hubs, dynamic risk management and return enhancement engines, cross-chain transfer speed, average yield rate and security incident response speed are improved. 3) Provide users with asset visualization, policy automation and governance integration, realizing user value reconstruction. 4) Through ecological collaboration and compliance innovation, the overall effectiveness of the platform has been enhanced. 5) In the future, smart contract insurance pools, forecast market integration and AI-driven asset allocation will be launched to continue to lead the development of the industry.
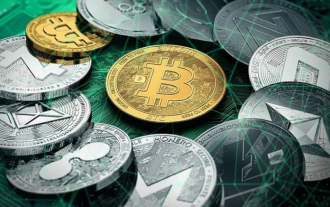
The top ten cryptocurrency trading platforms in the world include Binance, OKX, Gate.io, Coinbase, Kraken, Huobi Global, Bitfinex, Bittrex, KuCoin and Poloniex, all of which provide a variety of trading methods and powerful security measures.
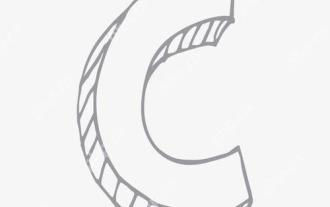
Measuring thread performance in C can use the timing tools, performance analysis tools, and custom timers in the standard library. 1. Use the library to measure execution time. 2. Use gprof for performance analysis. The steps include adding the -pg option during compilation, running the program to generate a gmon.out file, and generating a performance report. 3. Use Valgrind's Callgrind module to perform more detailed analysis. The steps include running the program to generate the callgrind.out file and viewing the results using kcachegrind. 4. Custom timers can flexibly measure the execution time of a specific code segment. These methods help to fully understand thread performance and optimize code.
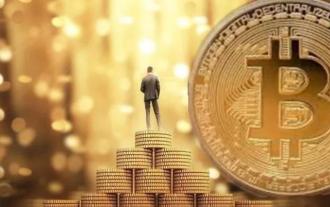
Recommended reliable digital currency trading platforms: 1. OKX, 2. Binance, 3. Coinbase, 4. Kraken, 5. Huobi, 6. KuCoin, 7. Bitfinex, 8. Gemini, 9. Bitstamp, 10. Poloniex, these platforms are known for their security, user experience and diverse functions, suitable for users at different levels of digital currency transactions
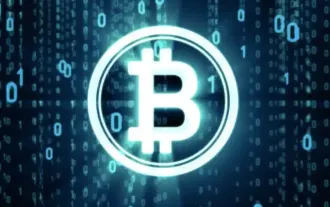
The top ten digital currency exchanges such as Binance, OKX, gate.io have improved their systems, efficient diversified transactions and strict security measures.
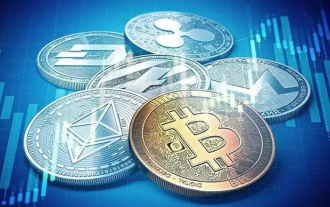
Currently ranked among the top ten virtual currency exchanges: 1. Binance, 2. OKX, 3. Gate.io, 4. Coin library, 5. Siren, 6. Huobi Global Station, 7. Bybit, 8. Kucoin, 9. Bitcoin, 10. bit stamp.
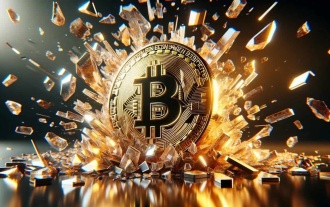
Bitcoin’s price ranges from $20,000 to $30,000. 1. Bitcoin’s price has fluctuated dramatically since 2009, reaching nearly $20,000 in 2017 and nearly $60,000 in 2021. 2. Prices are affected by factors such as market demand, supply, and macroeconomic environment. 3. Get real-time prices through exchanges, mobile apps and websites. 4. Bitcoin price is highly volatile, driven by market sentiment and external factors. 5. It has a certain relationship with traditional financial markets and is affected by global stock markets, the strength of the US dollar, etc. 6. The long-term trend is bullish, but risks need to be assessed with caution.
