


Don't panic if you revise your paper 100 times! Meta releases new writing language model PEER: references will be added
In the nearly two and a half years since its release in May 2020, GPT-3 has been able to assist humans in writing very well with the blessing of its magical text generation capabilities.
But in the final analysis, GPT-3 is a text generation model, which can be said to be completely different from the human writing process.
For example, if we want to write a paper or composition, we need to first construct a framework in our mind, check relevant information, make a draft, and then find a tutor to constantly revise and polish the text. During this period, we may also revise the ideas, and finally Only then can it become a good article.
The text obtained by the generative model can only meet the grammatical requirements, but has no logic in content arrangement, and has no ability to self-modify, so it is still far away to let AI write independently.
Recently, researchers from Meta AI Research and Carnegie Mellon University proposed a new text generation model PEER (Plan, Edit Edit, Explain, Repeat), which completely simulates the process of human writing. From drafting, soliciting suggestions, editing text, and iterating.
Paper address: https://arxiv.org/abs/2208.11663
PEER solves the problem that traditional language models can only generate The final result is that the generated text cannot be controlled. By inputting natural language commands, PEER can modify the generated text.
The most important thing is that the researchers trained multiple instances of PEER, which can fill in multiple links in the writing process, so that self-training can be used ) technology improves the quality, quantity, and diversity of training data.
The ability to generate training data means that the potential of PEER goes far beyond writing essays. PEER can also be used in other fields without editing history, allowing it to gradually improve itself by following instructions, writing useful comments, and explaining its ability to behave.
NLP also comes to bionics
After pre-training with natural language, the text generation effect of large-scale neural networks is already very strong, but the generation method of these models is basically from left to right. Outputting the resulting text all at once is very different from the iterative process of human writing.
One-time generation also has many disadvantages. For example, it is impossible to trace back the sentences in the text to modify or improve it, nor can it explain the reason why a certain sentence of text was generated. It is also difficult to test the correctness of the generated text, and there are often errors in the results. Generate hallucinate content, that is, text that does not correspond to fact. These flaws also limit the model's ability to write in collaboration with humans, who require coherent and factual text.
The PEER model is trained on the "editing history" of the text, allowing the model to simulate the human writing process.
1. When the PEER model is running, the user or model needs to specify a plan (Plan) and describe the action (action) they want to perform through natural language, such as add some information or fix grammar errors;
2. Then perform this action by editing the text;
3. The model can be explained in natural language and pointing to relevant resources (Explain) The result of this editing, such as adding a reference at the end of the text;
4. Repeat the process until the generated text no longer requires further updates.
This iterative approach not only allows the model to break down the complex task of writing a coherent, consistent, factual text into multiple easier subtasks, but also allows humans to be more involved in the generation process. Intervene at any time to guide the model in the right direction, provide users with plans and comments, or start editing it yourself.
It can be seen from the method description that the most difficult thing in realizing the function is not to use Transformer to build the model, but to find training data, and want to find a way to train large languages. It is obviously difficult to learn the data required for this process at the scale required for the model, because most websites do not provide editing history, so web pages obtained through crawlers cannot be used as training data.
Even obtaining the same web page at different times as editing history through a crawler is not feasible because there is no relevant text that plans or explains the edit.
PEER is similar to previous iterative editing methods, using Wikipedia as the data source for primary edits and related comments, because Wikipedia provides a complete history of edits, including comments on a variety of topics, and is large-scale in articles. Quotations are often included and are helpful in finding relevant documents.
But relying solely on Wikipedia as the only source of training data also has various shortcomings:
1. The model trained using only Wikipedia will have a negative impact on the expected text content and prediction plan. The editing needs to be similar to Wikipedia;
2. Comments in Wikipedia are noisy, so in many cases comments are not appropriate input for planning or explanation;
3. Many passages in Wikipedia do not contain any citations, and while this lack of background information can be compensated for by using a retrieval system, even such a system may not be able to find supporting background information for many editors.
The researchers proposed a simple method to solve all the problems caused by Wikipedia being the only source of comment editing history: train multiple PEER instances and use these instances to learn to populate various aspects of the editing process. These models can be used to generate synthetic data as a replacement for missing parts of the training corpus.
Finally trained four encoder-decoder models:
1. The input of PEER-Edit is text x and a set of documents, and the model output is the plan and the edited text, where p is the planned text.
2. The input of PEER-Undo is the edited text and a set of documents, and the model output is whether to undo the edit.
3. PEER-Explain is used to generate an explanation for the edit. The input is source text, edited text and a set of related documents.
4. PEER-Document inputs source text, edited text and plan, and the model output is the most useful background information in this edit.
All variant models of PEER are used to generate synthetic data, both to generate training data to supplement missing parts and to replace "low-quality" parts of existing data.
In order to be able to train arbitrary text data, even if the text has no editing history, PEER-Undo is used to generate synthetic "backward" editing, that is, PEER-Undo is repeatedly applied to the source text until the text is Empty, then call PEER-Edit to train in the opposite direction.
When generating plans, use PEER-Explain to correct many low-quality comments in the corpus, or to process texts with no comments. Randomly sample multiple results from the output of PEER-Explain as "potential plans", calculate the likelihood of actual editing, and select the one with the highest probability as the new plan.
If the relevant document cannot be found for a specific editing operation, PEER-Document is used to generate a set of synthetic documents containing information for performing the editing operation. Most importantly, PEER-Edit only does this during training and does not provide any synthetic documents during the inference phase.
In order to improve the quality and diversity of the generated plans, edits, and documents, the researchers also implemented a control mechanism that presets specific control markers in the output sequence generated by the model being trained, and then inferred These control tags are used in the process to guide the generation of the model. The tags include:
1. type is used to control the text type generated by PEER-Explain. The optional value is instruction (the output must start with the infinitive to.. ..) and other;
2, length, controls the output length of PEER-Explain. Optional values include s (less than 2 words), m (2-3 words), l (4- 5 words) and
4. words, used to control the number of different words between the source text and the edited text of PEER-Undo. The optional values are all integers; 5. contains, used to ensure that PEER-Undo The text output by Document contains a certain substringPEER does not introduce control characters to PEER-edit, that is, it does not assume the type of editing tasks that users may use the model to solve, making the model more versatile. In the experimental comparison phase, PEER uses the 3B parameter version of LM-Adapted T5 for pre-training initialization. To evaluate PEER's ability to follow a series of plans, utilize provided documents, and make edits in different domains, especially in domains with no editing history, a new dataset is introduced. Natural Edits, a collection of naturally occurring edits for different text types and domains.Data were collected from three English-language web sources: encyclopedia pages from Wikipedia, news articles from Wikinews, and StackExchange subforums for Cooking, Gardening, Law, Film, Politics, Travel, and Workplace. Gathering issues, all of these sites provide an edit history with comments that detail the editor's intent and feed it to the model as a plan.
In the training of Wikinews and StackExchange subsets, only plain text data is provided instead of actual editing, thereby testing the editing ability in areas without editing history.
The experimental results show that the performance of PEER exceeds all baselines to a certain extent, and the plan and documentation provide complementary information that the model can use
Evaluating PEER on all subsets of Natural Edits reveals that planning significantly helps across domains, suggesting that the ability to understand planning in Wikipedia editing is directly transferable to other domains. Importantly, the domain-adaptive variant of PEER significantly outperforms regular PEER on all subsets of Natural Edits, especially with large improvements on the gardening, politics, and movie subsets (84%, 71%, respectively). % and 48% of EM-Diff), also shows the effectiveness of generating synthetic edits when applying PEER in different domains.
The above is the detailed content of Don't panic if you revise your paper 100 times! Meta releases new writing language model PEER: references will be added. For more information, please follow other related articles on the PHP Chinese website!

Hot AI Tools

Undresser.AI Undress
AI-powered app for creating realistic nude photos

AI Clothes Remover
Online AI tool for removing clothes from photos.

Undress AI Tool
Undress images for free

Clothoff.io
AI clothes remover

Video Face Swap
Swap faces in any video effortlessly with our completely free AI face swap tool!

Hot Article

Hot Tools

Notepad++7.3.1
Easy-to-use and free code editor

SublimeText3 Chinese version
Chinese version, very easy to use

Zend Studio 13.0.1
Powerful PHP integrated development environment

Dreamweaver CS6
Visual web development tools

SublimeText3 Mac version
God-level code editing software (SublimeText3)

Hot Topics










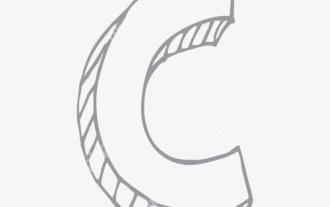
Using the chrono library in C can allow you to control time and time intervals more accurately. Let's explore the charm of this library. C's chrono library is part of the standard library, which provides a modern way to deal with time and time intervals. For programmers who have suffered from time.h and ctime, chrono is undoubtedly a boon. It not only improves the readability and maintainability of the code, but also provides higher accuracy and flexibility. Let's start with the basics. The chrono library mainly includes the following key components: std::chrono::system_clock: represents the system clock, used to obtain the current time. std::chron
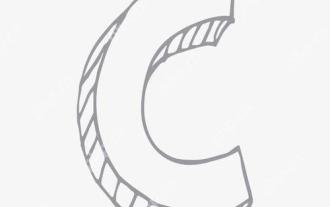
DMA in C refers to DirectMemoryAccess, a direct memory access technology, allowing hardware devices to directly transmit data to memory without CPU intervention. 1) DMA operation is highly dependent on hardware devices and drivers, and the implementation method varies from system to system. 2) Direct access to memory may bring security risks, and the correctness and security of the code must be ensured. 3) DMA can improve performance, but improper use may lead to degradation of system performance. Through practice and learning, we can master the skills of using DMA and maximize its effectiveness in scenarios such as high-speed data transmission and real-time signal processing.
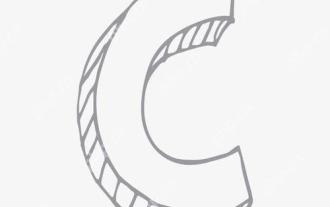
Handling high DPI display in C can be achieved through the following steps: 1) Understand DPI and scaling, use the operating system API to obtain DPI information and adjust the graphics output; 2) Handle cross-platform compatibility, use cross-platform graphics libraries such as SDL or Qt; 3) Perform performance optimization, improve performance through cache, hardware acceleration, and dynamic adjustment of the details level; 4) Solve common problems, such as blurred text and interface elements are too small, and solve by correctly applying DPI scaling.
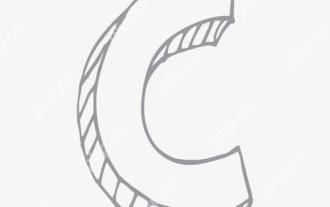
C performs well in real-time operating system (RTOS) programming, providing efficient execution efficiency and precise time management. 1) C Meet the needs of RTOS through direct operation of hardware resources and efficient memory management. 2) Using object-oriented features, C can design a flexible task scheduling system. 3) C supports efficient interrupt processing, but dynamic memory allocation and exception processing must be avoided to ensure real-time. 4) Template programming and inline functions help in performance optimization. 5) In practical applications, C can be used to implement an efficient logging system.
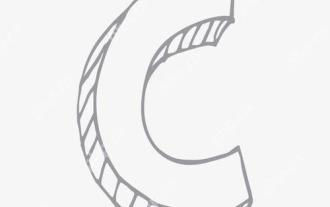
Measuring thread performance in C can use the timing tools, performance analysis tools, and custom timers in the standard library. 1. Use the library to measure execution time. 2. Use gprof for performance analysis. The steps include adding the -pg option during compilation, running the program to generate a gmon.out file, and generating a performance report. 3. Use Valgrind's Callgrind module to perform more detailed analysis. The steps include running the program to generate the callgrind.out file and viewing the results using kcachegrind. 4. Custom timers can flexibly measure the execution time of a specific code segment. These methods help to fully understand thread performance and optimize code.
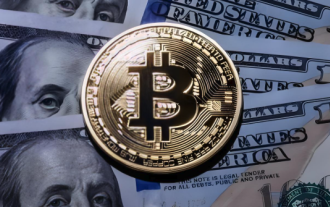
The built-in quantization tools on the exchange include: 1. Binance: Provides Binance Futures quantitative module, low handling fees, and supports AI-assisted transactions. 2. OKX (Ouyi): Supports multi-account management and intelligent order routing, and provides institutional-level risk control. The independent quantitative strategy platforms include: 3. 3Commas: drag-and-drop strategy generator, suitable for multi-platform hedging arbitrage. 4. Quadency: Professional-level algorithm strategy library, supporting customized risk thresholds. 5. Pionex: Built-in 16 preset strategy, low transaction fee. Vertical domain tools include: 6. Cryptohopper: cloud-based quantitative platform, supporting 150 technical indicators. 7. Bitsgap:
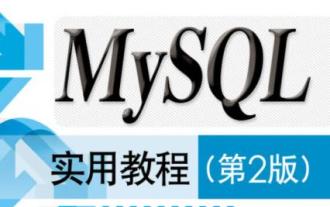
In MySQL, add fields using ALTERTABLEtable_nameADDCOLUMNnew_columnVARCHAR(255)AFTERexisting_column, delete fields using ALTERTABLEtable_nameDROPCOLUMNcolumn_to_drop. When adding fields, you need to specify a location to optimize query performance and data structure; before deleting fields, you need to confirm that the operation is irreversible; modifying table structure using online DDL, backup data, test environment, and low-load time periods is performance optimization and best practice.
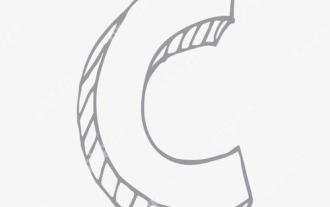
The main steps and precautions for using string streams in C are as follows: 1. Create an output string stream and convert data, such as converting integers into strings. 2. Apply to serialization of complex data structures, such as converting vector into strings. 3. Pay attention to performance issues and avoid frequent use of string streams when processing large amounts of data. You can consider using the append method of std::string. 4. Pay attention to memory management and avoid frequent creation and destruction of string stream objects. You can reuse or use std::stringstream.
