


Diffusion + target detection = controllable image generation! The Chinese team proposed GLIGEN to perfectly control the spatial position of objects
With the open source of Stable Diffusion, the use of natural language for image generation has gradually become popular. Many AIGC problems have also been exposed, such as AI cannot draw hands, cannot understand action relationships, and is difficult to control the position of objects. wait.
The main reason is that the "input interface" only has natural language, cannot achieve fine control of the screen.
Recently, research hotspots from the University of Wisconsin-Madison, Columbia University and Microsoft have proposed a brand new method GLIGEN, which uses grounding input as a condition to convert existing "pre-trained text to image" The functionality of Diffusion Model has been expanded.
Paper link: https://arxiv.org/pdf/2301.07093.pdf
Project home page: https://gligen.github.io/
Experience link: https: //huggingface.co/spaces/gligen/demo
In order to retain the large amount of conceptual knowledge of the pre-trained model, the researchers did not choose to fine-tune the model, but through gating The mechanism injects different input grounding conditions into new trainable layers to achieve control over open-world image generation.
Currently GLIGEN supports four inputs.
(Upper left) Text entity box (Upper right) Image entity box
(Bottom left) Image style text box (Bottom right) Text entity key points
The experimental results also show that GLIGEN has better performance in COCO and LVIS The zero-shot performance is significantly better than the current supervised layout-to-image baseline.
Controllable image generation
Before the diffusion model, generative adversarial networks (GANs) have always been the leader in the field of image generation, and their latent space and conditional input are in " The aspects of "controllable operations" and "generation" have been fully studied.
Text conditional autoregressive and diffusion models exhibit amazing image quality and concept coverage, thanks to their more stable learning objectives and large-scale access to network image-text paired data Train and quickly get out of the circle, becoming a tool to assist art design and creation.
However, existing large-scale text-image generation models cannot be conditioned on other input modes "besides text" and lack the concept of precise positioning or the use of reference images to control the generation process. Ability limits the expression of information.
For example, it is difficult to describe the precise location of an object using text, while bounding boxes (bounding
boxes) or keypoints (keypoints ) can be easily implemented.
Some existing tools such as inpainting, layout2img generation, etc. can use modal input other than text, but they are These inputs are rarely combined for controlled text2img generation.
In addition, previous generative models are usually trained independently on task-specific datasets, while in the field of image recognition, the long-standing paradigm is to learn from "large-scale image data" Or pre-trained basic models on "image-text pairs" to start building models for specific tasks.
Diffusion models have been trained on billions of image-text pairs. A natural question is: can we build on existing pre-trained diffusion models? , give them a new conditional input mode?
Due to the large amount of conceptual knowledge possessed by the pre-trained model, it may be possible to achieve better performance on other generation tasks while obtaining more data than existing text-image generation models. control.
GLIGEN
Based on the above purposes and ideas, the GLIGEN model proposed by the researchers still retains the text title as input, but also enables other input modalities, such as the grounding concept The bounding box, grounding reference image and key points of the grounding part.
The key problem here is to retain a large amount of original conceptual knowledge in the pre-trained model while learning to inject new grounding information.
In order to prevent knowledge forgetting, researchers proposed to freeze the original model weights and add a new trainable gated Transformer layer to absorb new grouding inputs. The following uses bounding boxes as an example. .
Command input
Each grouding text entity is represented as a bounding box, containing the coordinate values of the upper left corner and lower right corner.
It should be noted that existing layout2img related work usually requires a concept dictionary and can only handle close-set entities (such as COCO categories) during the evaluation phase. Researchers found that using Text encoders encoding image descriptions can generalize the location information in the training set to other concepts.
Training data
is used to generate grounding images The training data requires text c and grounding entity e as conditions. In practice, the data requirements can be relaxed by considering more flexible inputs.
There are three main types of data
1. grounding data
#Each image is associated with a caption that describes the entire image; noun entities are extracted from the caption and labeled with bounding boxes.
Since noun entities are taken directly from natural language titles, they can cover a richer vocabulary, which is beneficial to the grounding generation of open-world vocabulary.
2. Detection data
The noun entity is a predefined close-set category (for example, in COCO 80 object categories), choose to use the empty title token in the classifier-free guide as the title.
The amount of detection data (millions level) is larger than the basic data (thousands of levels), so the overall training data can be greatly increased.
3. Detection and Caption data
The noun entity is the same as the noun entity in the detection data , while the image is described with a text title alone, there may be cases where the noun entity is not completely consistent with the entity in the title.
For example, the title only gives a high-level description of the living room and does not mention the objects in the scene, while the detection annotation provides finer object-level details.
Gated attention mechanism
Researcher The goal is to give existing large-scale language-image generative models new spatially based capabilities.
#Large-scale diffusion models have been pre-trained on network-scale image text to obtain The knowledge required to synthesize realistic images based on diverse and complex language instructions. Since pre-training is expensive and performs well, it is important to retain this knowledge in the model weights while extending new capabilities. This can be achieved by Adapt new modules to accommodate new capabilities over time.
During the training process, the gating mechanism is used to gradually integrate new grounding information into the pre-trained model. This design allows flexibility in the sampling process during generation to improve quality and controllability.
The experiment also proved that using the complete model (all layers) in the first half of the sampling step, and only using the original layer (no gated Transformer layer) in the second half, generates The results can more accurately reflect grounding conditions and have higher image quality.
Experimental part
In the open set grounded text to image generation task, first use only the basic annotations of COCO (COCO2014CD) for training, and evaluate whether GLIGEN can generate basic entities other than the COCO category. .
It can be seen that GLIGEN can learn new concepts such as "blue jay", "croissant", or new Object attributes such as "brown wooden table", and this information does not appear in the training category.
The researchers believe this is because GLIGEN's gated self-attention learned to reposition visual features corresponding to grounded entities in the title for the following cross-attention layer, and Generalization capabilities are gained due to the shared text space in these two layers.
The experiment also quantitatively evaluated the zero-shot generation performance of this model on LVIS, which contains 1203 long-tail object categories. Use GLIP to predict bounding boxes from generated images and calculate AP, named GLIP score; compare it with state-of-the-art models designed for the layout2img task,
It can be found that although the GLIGEN model is only trained on COCO annotations, it is much better than the supervised baseline, probably because the baseline trained from scratch is difficult to start from Learning from limited annotations, while the GLIGEN model can utilize the large amount of conceptual knowledge of the pre-trained model.
Overall, this paper:
1. A new text2img generation method is proposed, which gives the existing text2img diffusion model new grounding controllability;
2. By retaining the pre-trained weights And learning gradually integrates new positioning layers, the model realizes open-world grounded text2img generation and bounding box input, that is, it integrates new positioning concepts not observed in training;
3. The zero-shot performance of this model on the layout2img task is significantly better than the previous state-of-the-art level, proving that large-scale pre-trained generative models can improve the performance of downstream tasks
The above is the detailed content of Diffusion + target detection = controllable image generation! The Chinese team proposed GLIGEN to perfectly control the spatial position of objects. For more information, please follow other related articles on the PHP Chinese website!

Hot AI Tools

Undresser.AI Undress
AI-powered app for creating realistic nude photos

AI Clothes Remover
Online AI tool for removing clothes from photos.

Undress AI Tool
Undress images for free

Clothoff.io
AI clothes remover

Video Face Swap
Swap faces in any video effortlessly with our completely free AI face swap tool!

Hot Article

Hot Tools

Notepad++7.3.1
Easy-to-use and free code editor

SublimeText3 Chinese version
Chinese version, very easy to use

Zend Studio 13.0.1
Powerful PHP integrated development environment

Dreamweaver CS6
Visual web development tools

SublimeText3 Mac version
God-level code editing software (SublimeText3)

Hot Topics










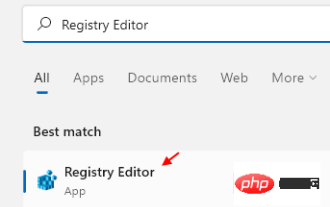
<p>Windows 11 improves personalization in the system, allowing users to view a recent history of previously made desktop background changes. When you enter the personalization section in the Windows System Settings application, you can see various options, changing the background wallpaper is one of them. But now you can see the latest history of background wallpapers set on your system. If you don't like seeing this and want to clear or delete this recent history, continue reading this article, which will help you learn more about how to do it using Registry Editor. </p><h2>How to use registry editing
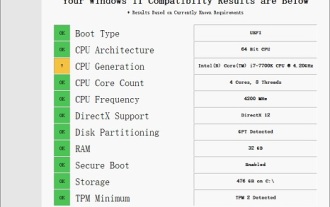
The performance of i77700 is completely sufficient to run win11, but users find that their i77700 cannot be upgraded to win11. This is mainly due to restrictions imposed by Microsoft, so they can install it as long as they skip this restriction. i77700 cannot be upgraded to win11: 1. Because Microsoft limits the CPU version. 2. Only the eighth generation and above versions of Intel can directly upgrade to win11. 3. As the 7th generation, i77700 cannot meet the upgrade needs of win11. 4. However, i77700 is completely capable of using win11 smoothly in terms of performance. 5. So you can use the win11 direct installation system of this site. 6. After the download is complete, right-click the file and "load" it. 7. Double-click to run the "One-click
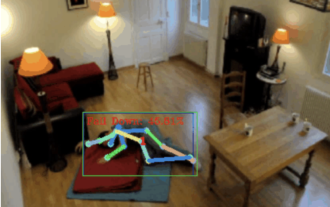
Hello everyone. Today I would like to share with you a fall detection project, to be precise, it is human movement recognition based on skeletal points. It is roughly divided into three steps: human body recognition, human skeleton point action classification project source code has been packaged, see the end of the article for how to obtain it. 0. chatgpt First, we need to obtain the monitored video stream. This code is relatively fixed. We can directly let chatgpt complete the code written by chatgpt. There is no problem and can be used directly. But when it comes to business tasks later, such as using mediapipe to identify human skeleton points, the code given by chatgpt is incorrect. I think chatgpt can be used as a toolbox that is independent of business logic. You can try to hand it over to c
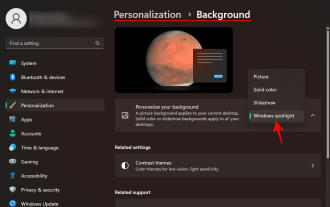
Windows are never one to neglect aesthetics. From the bucolic green fields of XP to the blue swirling design of Windows 11, default desktop wallpapers have been a source of user delight for years. With Windows Spotlight, you now have direct access to beautiful, awe-inspiring images for your lock screen and desktop wallpaper every day. Unfortunately, these images don't hang out. If you have fallen in love with one of the Windows spotlight images, then you will want to know how to download them so that you can keep them as your background for a while. Here's everything you need to know. What is WindowsSpotlight? Window Spotlight is an automatic wallpaper updater available from Personalization > in the Settings app
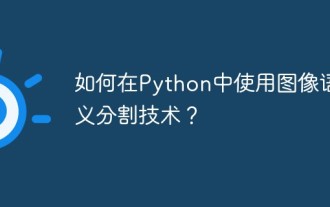
With the continuous development of artificial intelligence technology, image semantic segmentation technology has become a popular research direction in the field of image analysis. In image semantic segmentation, we segment different areas in an image and classify each area to achieve a comprehensive understanding of the image. Python is a well-known programming language. Its powerful data analysis and data visualization capabilities make it the first choice in the field of artificial intelligence technology research. This article will introduce how to use image semantic segmentation technology in Python. 1. Prerequisite knowledge is deepening
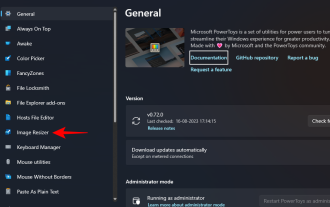
Those who have to work with image files on a daily basis often have to resize them to fit the needs of their projects and jobs. However, if you have too many images to process, resizing them individually can consume a lot of time and effort. In this case, a tool like PowerToys can come in handy to, among other things, batch resize image files using its image resizer utility. Here's how to set up your Image Resizer settings and start batch resizing images with PowerToys. How to Batch Resize Images with PowerToys PowerToys is an all-in-one program with a variety of utilities and features to help you speed up your daily tasks. One of its utilities is images
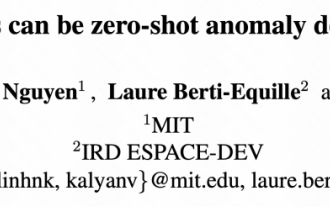
Today I would like to introduce to you an article published by MIT last week, using GPT-3.5-turbo to solve the problem of time series anomaly detection, and initially verifying the effectiveness of LLM in time series anomaly detection. There is no finetune in the whole process, and GPT-3.5-turbo is used directly for anomaly detection. The core of this article is how to convert time series into input that can be recognized by GPT-3.5-turbo, and how to design prompts or pipelines to let LLM solve the anomaly detection task. Let me introduce this work to you in detail. Image paper title: Largelanguagemodelscanbezero-shotanomalydete
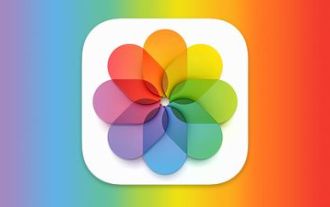
With the iOS 17 Photos app, Apple makes it easier to crop photos to your specifications. Read on to learn how. Previously in iOS 16, cropping an image in the Photos app involved several steps: Tap the editing interface, select the crop tool, and then adjust the crop using a pinch-to-zoom gesture or dragging the corners of the crop tool. In iOS 17, Apple has thankfully simplified this process so that when you zoom in on any selected photo in your Photos library, a new Crop button automatically appears in the upper right corner of the screen. Clicking on it will bring up the full cropping interface with the zoom level of your choice, so you can crop to the part of the image you like, rotate the image, invert the image, or apply screen ratio, or use markers
