


Based on PyTorch, easy to use, fine-grained image recognition deep learning tool library Hawkeye is open source
Fine-grained image recognition [1] is an important research topic in visual perception learning. It has great application value in the intelligent new economy and industrial Internet, and has been widely used in many real-world scenarios... In view of the lack of information in the current field As an open source tool library for deep learning in this area, the team of Professor Wei Xiucen of Nanjing University of Science and Technology spent nearly a year developing, polishing and completing Hawkeye - an open source tool library for fine-grained image recognition deep learning for reference by researchers and engineers in related fields. use. This article is a detailed introduction to Hawkeye.
1. What is Hawkeye library
Hawkeye is a fine-grained image recognition deep learning tool library based on PyTorch, specially designed for researchers and engineers in related fields. Currently, Hawkeye includes a variety of fine-grained recognition methods of representative paradigms, including "based on depth filters", "based on attention mechanisms", "based on high-order feature interactions", "based on special loss functions", "based on network data" and other methods.
Hawkeye project code style is good, the structure is clear and easy to read, and the scalability is strong. For those who are new to the field of fine-grained image recognition, Hawkeye is easier to get started, making it easier for them to understand the main processes and representative methods of fine-grained image recognition, and it is also convenient for them to quickly implement their own algorithms on this tool library. In addition, we also provide training example codes for each model in the library. Self-developed methods can also be quickly adapted and added to Hawkeye according to the examples.
Hawkeye open source library link: https://github.com/Hawkeye-FineGrained/Hawkeye
2. Models and methods supported by Hawkeye
Hawkeye currently supports fine-grained images There are a total of 16 models and methods of the main learning paradigms in recognition, as follows:
Based on deep filter
- S3N (ICCV 2019)
- Interp-Parts (CVPR 2020)
- ProtoTree (CVPR 2021)
Based on attention mechanism
- OSME MAMC (ECCV 2018)
- MGE-CNN (ICCV 2019)
- APCNN (IEEE TIP 2021)
##Based on high-order feature interaction
- BCNN (ICCV 2015)
- CBCNN (CVPR 2016)
- Fast MPN-COV (CVPR 2018)
Based on special loss function
- Pairwise Confusion (ECCV 2018)
- API-Net (AAAI 2020)
- CIN (AAAI 2020)
Based on network data
Peer-Learning (ICCV 2021)Other methodsNTS-Net (ECCV 2018)CrossX (ICCV 2019)DCL (CVPR 2019)3. Install HawkeyeInstall dependencies
Use conda or pip to install related dependencies:- Python 3.8
- PyTorch 1.11.0 or higher
- torchvison 0.12.0 or higher
- numpy
- yacs
- tqdm
Preparing data sets
We provide 8 commonly used fine-grained recognition data sets and the latest download link:- CUB200: https://data.caltech.edu/records/65de6-vp158/files/CUB_200_2011.tgz
- Stanford Dog: http://vision.stanford.edu/aditya86/ImageNetDogs/images.tar
- Stanford Car: http://ai.stanford.edu/~jkrause/car196/car_ims.tgz
- FGVC Aircraft: https://www.robots.ox.ac.uk/~vgg/ data/fgvc-aircraft/archives/fgvc-aircraft-2013b.tar.gz
- iNat2018: https://ml-inat-competition-datasets.s3.amazonaws.com/2018/train_val2018.tar.gz
- WebFG-bird: https://web-fgvc-496-5089-sh.oss-cn-shanghai.aliyuncs.com/web-bird.tar.gz
- WebFG-car : https://web-fgvc-496-5089-sh.oss-cn-shanghai.aliyuncs.com/web-car.tar.gz
- WebFG-aircraft: https://web-fgvc- 496-5089-sh.oss-cn-shanghai.aliyuncs.com/web-aircraft.tar.gz
cd Hawkeye/data wget https://data.caltech.edu/records/65de6-vp158/files/CUB_200_2011.tgz mkdir bird && tar -xvf CUB_200_2011.tgz -C bird/
dataset: name: cub root_dir: data/bird/CUB_200_2011/images meta_dir: metadata/cub
python Examples/APINet.py --config configs/APINet.yaml
experiment: name: API_res101 2# 实验名称 log_dir: results/APINet # 实验日志、结果等的输出目录 seed: 42# 可以选择固定的随机数种子 resume: results/APINet/API_res101 2/checkpoint_epoch_19.pth# 可以从训练中断的 checkpoint 中恢复训练 dataset: name: cub# 使用 CUB200 数据集 root_dir: data/bird/CUB_200_2011/images # 数据集中图像放置的路径 meta_dir: metadata/cub# CUB200 的 metadata 路径 n_classes: 10 # 类别数,APINet 需要的数据集 n_samples: 4# 每个类别的样本数 batch_size: 24# 测试时的批样本数 num_workers: 4# Dataloader 加载数据集的线程数 transformer:# 数据增强的参数配置 image_size: 224# 图像输入模型的尺寸 224x224 resize_size: 256# 图像增强前缩放的尺寸 256x256 model: name: APINet# 使用 APINet 模型,见 model/methods/APINet.py num_classes: 200# 类别数目 load: results/APINet/API_res101 1/best_model.pth # 可以加载训练过的模型参数 train: cuda: [4]# 使用的 GPU 设备 ID 列表,[] 时使用 CPU epoch: 100# 训练的 epoch 数量 save_frequence: 10# 自动保存模型的频率 val_first: False# 可选是否在训练前进行一次模型精度的测试 optimizer: name: Adam# 使用 Adam 优化器 lr: 0.0001# 学习率为 0.0001 weight_decay: 0.00000002 scheduler: # 本例使用自定义组合的 scheduler,由 warmup 和余弦退火学习率组合而成,见 Examples/APINet.py name: '' T_max: 100# scheduler 的总迭代次数 warmup_epochs: 8# warmup 的 epoch 数 lr_warmup_decay: 0.01# warmup 衰减的比例 criterion: name: APINetLoss# APINet 使用的损失函数,见 model/loss/APINet_loss.py
For more detailed examples, please refer to the specific information in the project link: https://github.com/Hawkeye-FineGrained/Hawkeye
The above is the detailed content of Based on PyTorch, easy to use, fine-grained image recognition deep learning tool library Hawkeye is open source. For more information, please follow other related articles on the PHP Chinese website!

Hot AI Tools

Undresser.AI Undress
AI-powered app for creating realistic nude photos

AI Clothes Remover
Online AI tool for removing clothes from photos.

Undress AI Tool
Undress images for free

Clothoff.io
AI clothes remover

Video Face Swap
Swap faces in any video effortlessly with our completely free AI face swap tool!

Hot Article

Hot Tools

Notepad++7.3.1
Easy-to-use and free code editor

SublimeText3 Chinese version
Chinese version, very easy to use

Zend Studio 13.0.1
Powerful PHP integrated development environment

Dreamweaver CS6
Visual web development tools

SublimeText3 Mac version
God-level code editing software (SublimeText3)

Hot Topics
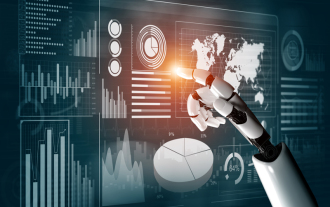
Text annotation is the work of corresponding labels or tags to specific content in text. Its main purpose is to provide additional information to the text for deeper analysis and processing, especially in the field of artificial intelligence. Text annotation is crucial for supervised machine learning tasks in artificial intelligence applications. It is used to train AI models to help more accurately understand natural language text information and improve the performance of tasks such as text classification, sentiment analysis, and language translation. Through text annotation, we can teach AI models to recognize entities in text, understand context, and make accurate predictions when new similar data appears. This article mainly recommends some better open source text annotation tools. 1.LabelStudiohttps://github.com/Hu
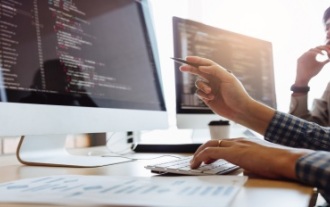
Image annotation is the process of associating labels or descriptive information with images to give deeper meaning and explanation to the image content. This process is critical to machine learning, which helps train vision models to more accurately identify individual elements in images. By adding annotations to images, the computer can understand the semantics and context behind the images, thereby improving the ability to understand and analyze the image content. Image annotation has a wide range of applications, covering many fields, such as computer vision, natural language processing, and graph vision models. It has a wide range of applications, such as assisting vehicles in identifying obstacles on the road, and helping in the detection and diagnosis of diseases through medical image recognition. . This article mainly recommends some better open source and free image annotation tools. 1.Makesens
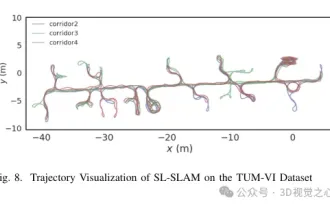
Written previously, today we discuss how deep learning technology can improve the performance of vision-based SLAM (simultaneous localization and mapping) in complex environments. By combining deep feature extraction and depth matching methods, here we introduce a versatile hybrid visual SLAM system designed to improve adaptation in challenging scenarios such as low-light conditions, dynamic lighting, weakly textured areas, and severe jitter. sex. Our system supports multiple modes, including extended monocular, stereo, monocular-inertial, and stereo-inertial configurations. In addition, it also analyzes how to combine visual SLAM with deep learning methods to inspire other research. Through extensive experiments on public datasets and self-sampled data, we demonstrate the superiority of SL-SLAM in terms of positioning accuracy and tracking robustness.
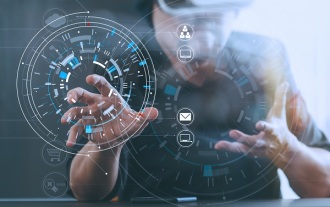
Face detection and recognition technology is already a relatively mature and widely used technology. Currently, the most widely used Internet application language is JS. Implementing face detection and recognition on the Web front-end has advantages and disadvantages compared to back-end face recognition. Advantages include reducing network interaction and real-time recognition, which greatly shortens user waiting time and improves user experience; disadvantages include: being limited by model size, the accuracy is also limited. How to use js to implement face detection on the web? In order to implement face recognition on the Web, you need to be familiar with related programming languages and technologies, such as JavaScript, HTML, CSS, WebRTC, etc. At the same time, you also need to master relevant computer vision and artificial intelligence technologies. It is worth noting that due to the design of the Web side
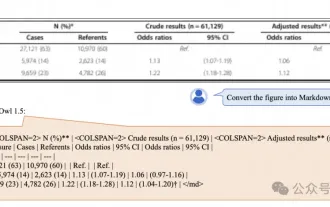
New SOTA for multimodal document understanding capabilities! Alibaba's mPLUG team released the latest open source work mPLUG-DocOwl1.5, which proposed a series of solutions to address the four major challenges of high-resolution image text recognition, general document structure understanding, instruction following, and introduction of external knowledge. Without further ado, let’s look at the effects first. One-click recognition and conversion of charts with complex structures into Markdown format: Charts of different styles are available: More detailed text recognition and positioning can also be easily handled: Detailed explanations of document understanding can also be given: You know, "Document Understanding" is currently An important scenario for the implementation of large language models. There are many products on the market to assist document reading. Some of them mainly use OCR systems for text recognition and cooperate with LLM for text processing.
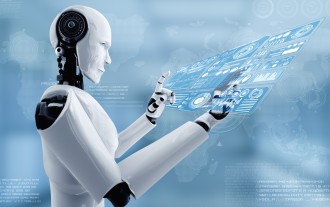
Almost 20 years have passed since the concept of deep learning was proposed in 2006. Deep learning, as a revolution in the field of artificial intelligence, has spawned many influential algorithms. So, what do you think are the top 10 algorithms for deep learning? The following are the top algorithms for deep learning in my opinion. They all occupy an important position in terms of innovation, application value and influence. 1. Deep neural network (DNN) background: Deep neural network (DNN), also called multi-layer perceptron, is the most common deep learning algorithm. When it was first invented, it was questioned due to the computing power bottleneck. Until recent years, computing power, The breakthrough came with the explosion of data. DNN is a neural network model that contains multiple hidden layers. In this model, each layer passes input to the next layer and
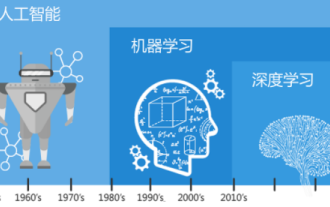
In today's wave of rapid technological changes, Artificial Intelligence (AI), Machine Learning (ML) and Deep Learning (DL) are like bright stars, leading the new wave of information technology. These three words frequently appear in various cutting-edge discussions and practical applications, but for many explorers who are new to this field, their specific meanings and their internal connections may still be shrouded in mystery. So let's take a look at this picture first. It can be seen that there is a close correlation and progressive relationship between deep learning, machine learning and artificial intelligence. Deep learning is a specific field of machine learning, and machine learning
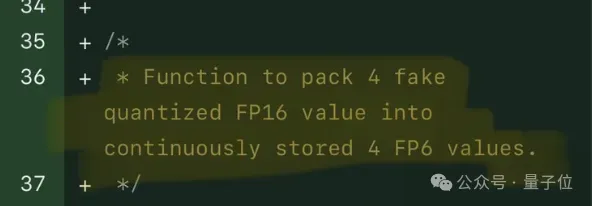
FP8 and lower floating point quantification precision are no longer the "patent" of H100! Lao Huang wanted everyone to use INT8/INT4, and the Microsoft DeepSpeed team started running FP6 on A100 without official support from NVIDIA. Test results show that the new method TC-FPx's FP6 quantization on A100 is close to or occasionally faster than INT4, and has higher accuracy than the latter. On top of this, there is also end-to-end large model support, which has been open sourced and integrated into deep learning inference frameworks such as DeepSpeed. This result also has an immediate effect on accelerating large models - under this framework, using a single card to run Llama, the throughput is 2.65 times higher than that of dual cards. one
