


From manual work to the industrial revolution! Nature article: Five areas of biological image analysis revolutionized by deep learning
One cubic millimeter does not sound big, it is the size of a sesame seed, but in the human brain, this small space can accommodate about 134 million synapses connected by 50,000 neural wires.
In order to generate the raw data, biological scientists need to use serial ultrathin section electron microscopy to analyze the data within 11 months Thousands of tissue fragments are imaged.
The amount of data finally obtained reached an astonishing1.4 PetaBytes (i.e. 1400TB, equivalent to the capacity of approximately 2 million CD-ROMs). For research For personnel, this is simply an astronomical figure.
Jeff Lichtman, a molecular and cellular biologist at Harvard University, said that if it were done purely by hand, it would be impossible for humans to manually trace all the nerve lines. There aren't enough people who can actually do this job effectively.The advancement of microscopy technology has brought a large amount of imaging data, but the amount of data is too large and the manpower is insufficient. This is also the reason why Connectomics
(Connectomics, a research The subject of brain structure and functional connections), as well ascommon phenomena in other biological fields. But The mission of computer science
is precisely to solve this type ofproblem of insufficient human resources, especially the optimized depth Learning algorithms can mine data patterns from large-scale data sets. Deep learning has had a huge push in biology over the past few years, says Beth Cimini, a computational biologist at the Broad Institute of MIT and Harvard University in Cambridge. role and developed many research tools.
The following are the editors of Nature summarizing the five areas of biological image analysis
that are brought about by deep learning.Large-Scale Connectomics
Deep learning enables researchers to generate increasingly complex connectomes from fruit flies, mice, and even humans.These data can help neuroscientists understand how the brain works and how brain structure changes during development and disease, but neural connections are not easy to map
.In 2018, Lichtman
joined forces withGoogle’s connectomics lead Viren Jain in Mountain View, California , to find solutions for the artificial intelligence algorithms the team needs. The image analysis task in connectomics is actually very difficult, you have to be able to trace these thin wires, the axons and dendrites of cells, over long distances ,Traditional image processing methods will have many errors in this task and are basically useless for this task
.These nerve strands can be thinner than a micron, extending hundreds of microns or even across millimeters of tissue.
And the deep learning algorithm
can not only automatically analyze connectomics data, but also maintainvery high accuracy. Researchers can use annotated data sets containing features of interest to train complex computational models that can quickly identify the same features in other data.
Anna Kreshuk, a computer scientist at the European Molecular Biology Laboratory, believes that the process of using deep learning algorithms is similar to "giving an example." As long as there are enough examples, you can solve all problems All solved.
But even using deep learning, Lichtman and Jain’s team still had a difficult task: mapping segments of the human cerebral cortex.
In the data collection phase
, it took326 days just to take more than 5,000 ultra-thin tissue sections. Two researchers spent about 100 hours manually annotating
images and tracking neurons to create a ground truth dataset to train the algorithm.The algorithm trained using standard data can automatically stitch together images, identify neurons and synapses, and generate the final connectome. Jain's team also invested a lot of computing resources to solve this problem, including thousands of tensor processing units (TPU) , and also spent A few months to pre-process the data required for 1 million TPU hours. Although researchers have obtained the largest data set currently collected and can reconstruct it at a very fine level, this amount of data Approximately only 0.0001% of the human brain #As algorithms and hardware improve, researchers should be able to map larger brain regions and simultaneously resolve more Cellular features such as organelles and even proteins. At least, deep learning provides a feasibility. Histology (histology) is an important tool in medicine, used on the basis of chemical or molecular staining Diagnose disease. But the whole process is time-consuming and laborious, and usually takes days or even weeks to complete. The biopsy is sliced into thin slices and stained to reveal cellular and subcellular features. The pathologist then reads and interprets the results. Aydogan Ozcan, a computer engineer at the University of California, Los Angeles, believes that the entire process can be accelerated through deep learning. He trained a customized deep learning model, used computer simulation to stain a tissue section, and tens of thousands of tissue sections on the same section. The unstained and dyed samples are fed to the model and the model calculates the difference between them. In addition to the time advantage of virtual staining (it can be completed in an instant), pathologists have discovered through observation that there is almost no difference between virtual staining and traditional staining Experimental results show that the algorithm can replicate the molecular staining of the breast cancer biomarker HER2 in seconds, a process that . An expert panel of three breast pathologists evaluated the images and deemed them to be of comparable quality and accuracy to traditional immunohistochemical stains. Ozcan sees the potential for commercializing virtual staining in drug development, but he hopes to eliminate the need for toxic dyes and expensive staining equipment in histology. If you want to extract data from cell images, then you must know the actual location of the cells in the image. This process is also called cell segmentation (cell segmentation). Researchers need to observe cells under a microscope, or outline the cells one by one in the software. Morgan Schwartz, a computational biologist at the California Institute of Technology, is looking for ways to automate processing. As imaging data sets become larger and larger, traditional manual methods are also encountering bottlenecks. Some experiments cannot be analyzed without automation. Schwartz’s graduate advisor, bioengineer David Van Valen, created a set of artificial intelligence models and published them on the deepcell.org website, which can be used to calculate and analyze living cells and preserve them. Cells and other features in tissue images. Van Valen, along with collaborators such as Stanford University cancer biologist Noah Greenwald, also developed a deep learning model Mesmer that can quickly , Accurately detect cells and nuclei of different tissue types According to Greenwald, researchers could use this information to distinguish cancerous tissue from non-cancerous tissue and look for differences before and after treatment, or based on imaging changes to better understand why some Will patients respond or not respond, as well as determine the tumor subtype. The Human Protein Atlas project takes advantage of another application of deep learning: intracellular localization. Emma Lundberg, a bioengineer at Stanford University, said that over the past few decades, the project has generated millions of images depicting what is happening in human cells and tissues. protein expression. At the beginning, project participants needed tomanually annotate these images, but this method was unsustainable, and Lundberg began to seek help from artificial intelligence algorithms. In the past few years, she began to initiate crowdsourcing solutions in the Kaggle Challenge, where scientists and artificial intelligence enthusiasts complete various computing tasks for prize money. The prize money for the two projects is 37,000 US dollars 25,000 US dollars respectively. Contestants will design supervised machine learning models and annotate protein map images. multi-label classification of protein localization patterns, and can be generalized to cell lines, and has also achieved new industry breakthroughs, accurately classifying proteins that exist in multiple cellular locations.
With the model in hand, biological experiments can move forward. The location of human proteins is important because the same proteins are present in Different places behave differently, and knowing whether a protein is in the nucleus or mitochondria can help understand its function. Mackenzie Mathis, a neuroscientist at the Biotechnology Center on the campus of Ecole Polytechnique Fédérale de Lausanne in Switzerland, has long been interested in how the brain drives behavior. track animals’ postures and fine movements from videos and Videos" and other animal recordings are converted into data. DeepLabcut provides a graphical user interface that allows researchers to upload and annotate videos and train deep learning models with the click of a button. In April of this year, Mathis' team expanded the software to estimate poses for at the same time, which is a benefit for both humans and artificial intelligence. A whole new challenge. Applying the model trained by DeepLabCut to marmosets, the researchers found that when these animals were very close, their bodies would line up in a straight line and look towards similar animals. direction, and when they are apart, they tend to face each other. Biologists identify animal postures to understand how two animals interact, gaze, or observe the world. .
Localizing proteins
Tracking Animal Behavior
The above is the detailed content of From manual work to the industrial revolution! Nature article: Five areas of biological image analysis revolutionized by deep learning. For more information, please follow other related articles on the PHP Chinese website!

Hot AI Tools

Undresser.AI Undress
AI-powered app for creating realistic nude photos

AI Clothes Remover
Online AI tool for removing clothes from photos.

Undress AI Tool
Undress images for free

Clothoff.io
AI clothes remover

Video Face Swap
Swap faces in any video effortlessly with our completely free AI face swap tool!

Hot Article

Hot Tools

Notepad++7.3.1
Easy-to-use and free code editor

SublimeText3 Chinese version
Chinese version, very easy to use

Zend Studio 13.0.1
Powerful PHP integrated development environment

Dreamweaver CS6
Visual web development tools

SublimeText3 Mac version
God-level code editing software (SublimeText3)

Hot Topics
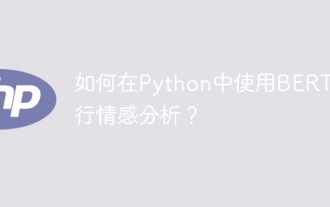
BERT is a pre-trained deep learning language model proposed by Google in 2018. The full name is BidirectionalEncoderRepresentationsfromTransformers, which is based on the Transformer architecture and has the characteristics of bidirectional encoding. Compared with traditional one-way coding models, BERT can consider contextual information at the same time when processing text, so it performs well in natural language processing tasks. Its bidirectionality enables BERT to better understand the semantic relationships in sentences, thereby improving the expressive ability of the model. Through pre-training and fine-tuning methods, BERT can be used for various natural language processing tasks, such as sentiment analysis, naming
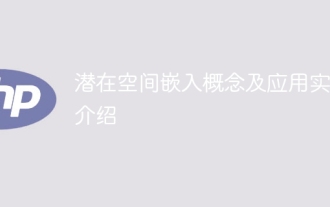
Latent Space Embedding (LatentSpaceEmbedding) is the process of mapping high-dimensional data to low-dimensional space. In the field of machine learning and deep learning, latent space embedding is usually a neural network model that maps high-dimensional input data into a set of low-dimensional vector representations. This set of vectors is often called "latent vectors" or "latent encodings". The purpose of latent space embedding is to capture important features in the data and represent them into a more concise and understandable form. Through latent space embedding, we can perform operations such as visualizing, classifying, and clustering data in low-dimensional space to better understand and utilize the data. Latent space embedding has wide applications in many fields, such as image generation, feature extraction, dimensionality reduction, etc. Latent space embedding is the main
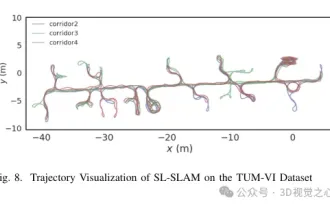
Written previously, today we discuss how deep learning technology can improve the performance of vision-based SLAM (simultaneous localization and mapping) in complex environments. By combining deep feature extraction and depth matching methods, here we introduce a versatile hybrid visual SLAM system designed to improve adaptation in challenging scenarios such as low-light conditions, dynamic lighting, weakly textured areas, and severe jitter. sex. Our system supports multiple modes, including extended monocular, stereo, monocular-inertial, and stereo-inertial configurations. In addition, it also analyzes how to combine visual SLAM with deep learning methods to inspire other research. Through extensive experiments on public datasets and self-sampled data, we demonstrate the superiority of SL-SLAM in terms of positioning accuracy and tracking robustness.
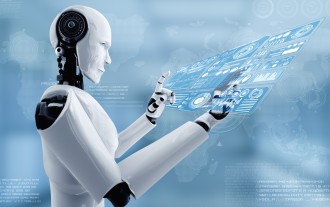
Almost 20 years have passed since the concept of deep learning was proposed in 2006. Deep learning, as a revolution in the field of artificial intelligence, has spawned many influential algorithms. So, what do you think are the top 10 algorithms for deep learning? The following are the top algorithms for deep learning in my opinion. They all occupy an important position in terms of innovation, application value and influence. 1. Deep neural network (DNN) background: Deep neural network (DNN), also called multi-layer perceptron, is the most common deep learning algorithm. When it was first invented, it was questioned due to the computing power bottleneck. Until recent years, computing power, The breakthrough came with the explosion of data. DNN is a neural network model that contains multiple hidden layers. In this model, each layer passes input to the next layer and
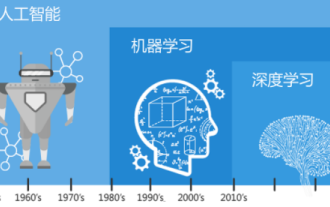
In today's wave of rapid technological changes, Artificial Intelligence (AI), Machine Learning (ML) and Deep Learning (DL) are like bright stars, leading the new wave of information technology. These three words frequently appear in various cutting-edge discussions and practical applications, but for many explorers who are new to this field, their specific meanings and their internal connections may still be shrouded in mystery. So let's take a look at this picture first. It can be seen that there is a close correlation and progressive relationship between deep learning, machine learning and artificial intelligence. Deep learning is a specific field of machine learning, and machine learning

Editor | Radish Skin Since the release of the powerful AlphaFold2 in 2021, scientists have been using protein structure prediction models to map various protein structures within cells, discover drugs, and draw a "cosmic map" of every known protein interaction. . Just now, Google DeepMind released the AlphaFold3 model, which can perform joint structure predictions for complexes including proteins, nucleic acids, small molecules, ions and modified residues. The accuracy of AlphaFold3 has been significantly improved compared to many dedicated tools in the past (protein-ligand interaction, protein-nucleic acid interaction, antibody-antigen prediction). This shows that within a single unified deep learning framework, it is possible to achieve
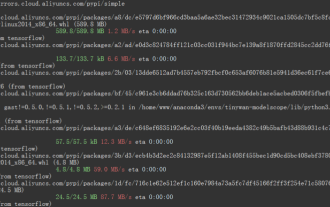
Overview In order to enable ModelScope users to quickly and conveniently use various models provided by the platform, a set of fully functional Python libraries are provided, which includes the implementation of ModelScope official models, as well as the necessary tools for using these models for inference, finetune and other tasks. Code related to data pre-processing, post-processing, effect evaluation and other functions, while also providing a simple and easy-to-use API and rich usage examples. By calling the library, users can complete tasks such as model reasoning, training, and evaluation by writing just a few lines of code. They can also quickly perform secondary development on this basis to realize their own innovative ideas. The algorithm model currently provided by the library is:
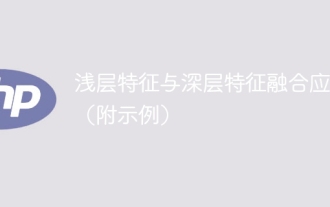
Deep learning has achieved great success in the field of computer vision, and one of the important advances is the use of deep convolutional neural networks (CNN) for image classification. However, deep CNNs usually require large amounts of labeled data and computing resources. In order to reduce the demand for computational resources and labeled data, researchers began to study how to fuse shallow features and deep features to improve image classification performance. This fusion method can take advantage of the high computational efficiency of shallow features and the strong representation ability of deep features. By combining the two, computational costs and data labeling requirements can be reduced while maintaining high classification accuracy. This method is particularly important for application scenarios where the amount of data is small or computing resources are limited. By in-depth study of the fusion methods of shallow features and deep features, we can further
