


Deep learning hits a wall? Who stirred the hornet's nest between LeCun and Marcus?
Today’s protagonists are a pair of old enemies who love and kill each other in the AI world:
Yann LeCun and Gary Marcus
Before we formally talk about this “new feud”, let’s first Let’s review the “old hatred” between the two great gods.
LeCun vs. Marcus battle
Chief artificial intelligence scientist at Facebook and professor at New York University, 2018 Turing Award (Turing Award) winner Yann LeCun published an article in NOEMA magazine in response to Gary Marcus’s previous comments on AI and deep learning.
Previously, Marcus published an article in the magazine Nautilus, saying that deep learning has "unable to move forward"
Marcus is a person who is just watching the excitement. Big lord.
As soon as there was a slight disturbance, he said "AI is dead", causing an uproar in the circle!
I have posted many times before, calling GPT-3 “Nonsense” and “bullshit”.
Here is the ironclad evidence:
Good guy, he actually said that "deep learning has hit a wall", seeing such rampant Comments, LeCun, a big star in the AI industry, couldn't sit still and immediately posted a response!
and said, if you want to line up, I will accompany you!
LeCun refuted Marcus’s views one by one in the article.
Let’s take a look at how the great master wrote an article to reply~~
The following is LeCun’s long article:
The dominant technology of contemporary artificial intelligence is deep learning (DL) ) Neural Network (NN), a large-scale self-learning algorithm that excels at identifying and exploiting patterns in data. From the beginning, critics prematurely believed that neural networks had hit an "insurmountable wall." Yet every time, it proved to be a temporary obstacle.
In the 1960s, NN could not solve nonlinear functions. But this situation did not last long. It was changed in the 1980s with the emergence of backpropagation, but a new "insurmountable wall" appeared again, that is, it was very difficult to train the system.
In the 1990s, humans developed simplified procedures and standardized structures, which made training more reliable, but no matter what results were achieved, there seemed to always be an "insurmountable wall." This time it was a lack of training data and computational power.
Deep learning started to go mainstream in 2012, when the latest GPUs could train on the massive ImageNet dataset, easily defeating all competitors. But then, voices of doubt emerged: people discovered "a new wall" - deep learning training requires a large amount of manually labeled data.
However, in the past few years, this doubt has become meaningless, because self-supervised learning has achieved quite good results, such as GPT-3, which does not require labeled data.
The seemingly insurmountable obstacle today is "symbolic reasoning," which is the ability to manipulate symbols in an algebraic or logical way. As we learned as children, solving mathematical problems requires manipulating symbols step by step according to strict rules (e.g., solving equations).
Gary Marcus, author of "The Algebraic Mind" and co-author of "Rebooting AI", recently believed that DL cannot make further progress because neural networks have difficulty processing such symbolic operations. In contrast, many DL researchers believe that DL is already doing symbolic reasoning and will continue to improve.
At the heart of this debate is the role of symbols in artificial intelligence. There are two different views: one believes that symbolic reasoning must be hard-coded from the beginning, while the other believes that machines can learn through experience. The ability to reason symbolically. Therefore, the key to the problem is how we should understand human intelligence, and therefore how we should pursue artificial intelligence that can achieve human levels.
Different types of artificial intelligence
The most important thing about symbolic reasoning is accuracy: according to the permutation and combination, symbols can have many different orders, such as "(3-2)-1 and 3- (2-1)" is important, so how to perform correct symbolic reasoning in the correct order is crucial.
Marcus believes that this kind of reasoning is the core of cognition and is crucial for providing the underlying grammatical logic for language and the basic operations for mathematics. He believes that this can extend to our more basic abilities, and that behind these abilities, there is an underlying symbolic logic.
The artificial intelligence we are familiar with starts from the study of this kind of reasoning, and is often called "symbolic artificial intelligence". But refining human expertise into a set of rules is very challenging and consumes huge time and labor costs. This is the so-called "knowledge acquisition bottleneck."
Although it is easy to write rules for mathematics or logic, the world itself is black and white and very fuzzy. It turns out that it is impossible for humans to write rules that control every pattern or for every fuzzy Concept definition symbol.
However, the development of science and technology has created neural networks, and what neural networks are best at is discovering patterns and accepting ambiguity.
A neural network is a relatively simple equation that learns a function to provide an appropriate output for whatever is input to the system.
For example, to train a two-classification network, put a large amount of sample data (here, take a chair as an example) into the neural network and train it for several epochs. , the final implementation allowed the network to successfully infer whether a new image was a chair.
To put it bluntly, this is not just a question about artificial intelligence, but more essentially, what is intelligence and how the human brain works. ”
These neural networks can be trained precisely because the function implementing it is differentiable. In other words, if symbolic AI is analogous to the discrete tokens used in symbolic logic, then neural networks are continuous functions of calculus .
This allows learning a better representation by fine-tuning the parameters, which means it can fit the data more appropriately without the problem of under-fitting or over-fitting. However, when it comes to This liquidity creates a new “wall” when it comes to strict rules and discrete tokens: when we solve an equation, we usually want the exact answer, not an approximate answer.
This is where Symbolic AI shines, so Marcus suggested simply combining the two: inserting a hard-coded symbolic manipulation module on top of the DL module.
This is attractive because the two approaches complement each other very well, so it would seem that a "hybrid" of modules with different ways of working would maximize the advantages of both approaches.
But the debate turns to whether symbolic manipulation needs to be built into a system where symbols and manipulation capabilities are designed by humans and the module is non-differentiable - and therefore incompatible with DL.
Legendary "Symbolic Reasoning"
This hypothesis is very controversial.
Traditional neural networks believe that we do not need to perform symbolic reasoning manually, but can learn symbolic reasoning, that is, using symbolic examples to train the machine to perform the correct type of reasoning, allowing it to learn the completion of abstract patterns. In short, machines can learn to manipulate symbols in the world, albeit without built-in hand-crafted symbols and symbol manipulation rules.
Contemporary large-scale language models such as GPT-3 and LaMDA show the potential of this approach. Their ability to manipulate symbols is astonishing, and these models exhibit astonishing common sense reasoning, combinatorial abilities, multilingual abilities, logical and mathematical abilities, and even the terrifying ability to imitate the dead.
But in fact this is not reliable. If you asked DALL-E to make a Roman sculpture of a philosopher with a beard, glasses, and a tropical shirt, it would be outstanding. But if you ask it to draw a beagle wearing a pink toy chasing a squirrel, sometimes you'll get a beagle wearing a pink toy or a squirrel.
It does a great job when it can assign all properties to one object, but it gets stuck when there are multiple objects and multiple properties. The attitude of many researchers is that this is "a wall" for DL on the road to more human-like intelligence.
So does symbolic operation need to be hard-coded? Or is it learnable?
This is not Marcus' understanding.
He assumes that symbolic reasoning is all-or-nothing - because DALL-E has no symbols and logical rules as the basis for its operations, it does not actually reason using symbols. Thus, the numerous failures of large language models indicate that they are not true reasoning, but merely emotionless mechanical imitation.
For Marcus, it was impossible to climb a tree large enough to reach the moon. Therefore, he believes that the current DL language model is no closer to real language than Nim Chimpsky (a male chimpanzee who can use American Sign Language). DALL-E's problem isn't a lack of training. They are simply systems that have not grasped the underlying logical structure of the sentence, and therefore cannot correctly grasp how the different parts should be connected into a whole.
In contrast, Geoffrey Hinton and others believe that neural networks can successfully manipulate symbols without requiring hard-coded symbols and algebraic reasoning. The goal of DL is not symbolic manipulation inside the machine, but rather learning to generate correct symbols from systems in the world.
Rejection of mixing the two modes is not rash but is based on philosophical differences in whether one believes symbolic reasoning can be learned.
The underlying logic of human thought
Marcus’ criticism of DL stems from a related debate in cognitive science about how intelligence works and what makes humans unique. His views are consistent with a well-known "nativist" school of thought in psychology, which holds that many key features of cognition are innate - in fact, we are largely born knowing how the world works.
The core of this innate perception is the ability to manipulate symbols (but there is no conclusion yet on whether this is found throughout nature or is unique to humans). For Marcus, this ability to manipulate symbols underlies many of the fundamental features of common sense: rule following, abstraction, causal reasoning, re-identification of details, generalization and many other abilities.
In short, much of our understanding of the world is given by nature, and learning is about enriching the details.
There is another empirical view that breaks the above idea: symbol manipulation is rare in nature and is mainly a learned communication ability that our ancient human ancestors gradually acquired over the past 2 million years.
From this perspective, the primary cognitive abilities are non-symbolic learning abilities related to improved survival capabilities, such as quickly identifying prey, predicting their likely actions, and developing skilled responses.
This view holds that most complex cognitive abilities are acquired through general, self-supervised learning abilities. It also assumes that most of our complex cognitive abilities do not rely on symbolic operations. Instead, they simulate various scenarios and predict the best outcomes.
This empirical view considers symbols and symbol manipulation to be just another learned ability, acquired as humans increasingly rely on cooperative behavior to succeed. This sees symbols as inventions we use to coordinate cooperation between groups – such as words, but also maps, iconic descriptions, rituals and even social roles.
The difference between these two views is very obvious. For the nativist tradition, symbols and symbol manipulation are inherently present in the mind, and the use of words and numbers derives from this primitive capacity. This view attractively explains abilities that arise from evolutionary adaptations (although explanations of how or why symbol manipulation evolved have been controversial).
Viewed from the perspective of the empiricist tradition, symbols and symbolic reasoning are a useful communicative invention that stems from general learning abilities and our complex social world. This treats the symbolic things that happen in our heads, such as internal computations and inner monologues, as external practices that arise from mathematics and language use.
The fields of artificial intelligence and cognitive science are closely intertwined, so it’s no surprise that these battles are reappearing here. Since the success of either view in artificial intelligence would partially (but only partially) vindicate one or another approach in cognitive science, it is not surprising that these debates have become intense.
The key to the problem lies not only in how to correctly solve the problems in the field of contemporary artificial intelligence, but also in solving what intelligence is and how the brain works.
Should I bet on AI or go short?
Why is the statement “deep learning hits a wall” so provocative?
If Marcus is right, then deep learning will never be able to achieve human-like AI, no matter how many new architectures it proposes or how much computing power it invests.
Continuing to add more layers to neural networks will only make things more confusing, because true symbol manipulation requires a natural symbol manipulator. Since this symbolic operation is the basis of several common sense abilities, DL will only "do not understand" anything.
In contrast, if DL advocates and empiricists are correct, what is puzzling is the idea of inserting a module for symbol manipulation.
In this context, deep learning systems are already doing symbolic reasoning and will continue to improve as they use more multimodal self-supervised learning, increasingly useful predictive world models, and Expansion of working memory for simulation and evaluation results to better satisfy constraints.
The introduction of symbolic operation modules will not create a more human-like AI. On the contrary, it will force all "reasoning" operations to go through an unnecessary bottleneck, which will take us further away from "human-like intelligence". This may cut off one of the most exciting aspects of deep learning: its ability to come up with better-than-human solutions.
Having said that, none of this justifies the silly hype: since current systems are not conscious, they can’t understand us, reinforcement learning isn’t enough, and you can’t build humanoids just by scaling them up intelligent. But all of these issues are "edge issues" in the main debate: Do symbolic operations need to be hard-coded? Or can they be learned? model)? Of course not. People should choose what works.
However, researchers have been working on hybrid models since the 1980s, but they have not been proven to be an effective approach and, in many cases, may even be far inferior to neural networks.
More generally speaking, one should wonder whether deep learning has reached its upper limit.
The above is the detailed content of Deep learning hits a wall? Who stirred the hornet's nest between LeCun and Marcus?. For more information, please follow other related articles on the PHP Chinese website!

Hot AI Tools

Undresser.AI Undress
AI-powered app for creating realistic nude photos

AI Clothes Remover
Online AI tool for removing clothes from photos.

Undress AI Tool
Undress images for free

Clothoff.io
AI clothes remover

Video Face Swap
Swap faces in any video effortlessly with our completely free AI face swap tool!

Hot Article

Hot Tools

Notepad++7.3.1
Easy-to-use and free code editor

SublimeText3 Chinese version
Chinese version, very easy to use

Zend Studio 13.0.1
Powerful PHP integrated development environment

Dreamweaver CS6
Visual web development tools

SublimeText3 Mac version
God-level code editing software (SublimeText3)

Hot Topics
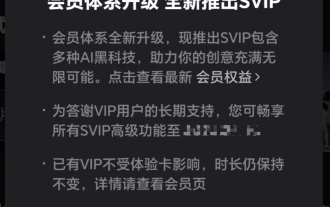
This site reported on June 27 that Jianying is a video editing software developed by FaceMeng Technology, a subsidiary of ByteDance. It relies on the Douyin platform and basically produces short video content for users of the platform. It is compatible with iOS, Android, and Windows. , MacOS and other operating systems. Jianying officially announced the upgrade of its membership system and launched a new SVIP, which includes a variety of AI black technologies, such as intelligent translation, intelligent highlighting, intelligent packaging, digital human synthesis, etc. In terms of price, the monthly fee for clipping SVIP is 79 yuan, the annual fee is 599 yuan (note on this site: equivalent to 49.9 yuan per month), the continuous monthly subscription is 59 yuan per month, and the continuous annual subscription is 499 yuan per year (equivalent to 41.6 yuan per month) . In addition, the cut official also stated that in order to improve the user experience, those who have subscribed to the original VIP
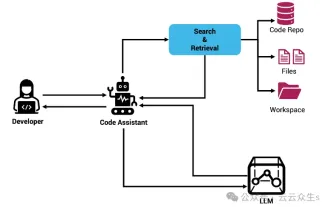
Improve developer productivity, efficiency, and accuracy by incorporating retrieval-enhanced generation and semantic memory into AI coding assistants. Translated from EnhancingAICodingAssistantswithContextUsingRAGandSEM-RAG, author JanakiramMSV. While basic AI programming assistants are naturally helpful, they often fail to provide the most relevant and correct code suggestions because they rely on a general understanding of the software language and the most common patterns of writing software. The code generated by these coding assistants is suitable for solving the problems they are responsible for solving, but often does not conform to the coding standards, conventions and styles of the individual teams. This often results in suggestions that need to be modified or refined in order for the code to be accepted into the application

To learn more about AIGC, please visit: 51CTOAI.x Community https://www.51cto.com/aigc/Translator|Jingyan Reviewer|Chonglou is different from the traditional question bank that can be seen everywhere on the Internet. These questions It requires thinking outside the box. Large Language Models (LLMs) are increasingly important in the fields of data science, generative artificial intelligence (GenAI), and artificial intelligence. These complex algorithms enhance human skills and drive efficiency and innovation in many industries, becoming the key for companies to remain competitive. LLM has a wide range of applications. It can be used in fields such as natural language processing, text generation, speech recognition and recommendation systems. By learning from large amounts of data, LLM is able to generate text
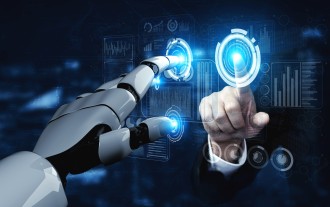
Large Language Models (LLMs) are trained on huge text databases, where they acquire large amounts of real-world knowledge. This knowledge is embedded into their parameters and can then be used when needed. The knowledge of these models is "reified" at the end of training. At the end of pre-training, the model actually stops learning. Align or fine-tune the model to learn how to leverage this knowledge and respond more naturally to user questions. But sometimes model knowledge is not enough, and although the model can access external content through RAG, it is considered beneficial to adapt the model to new domains through fine-tuning. This fine-tuning is performed using input from human annotators or other LLM creations, where the model encounters additional real-world knowledge and integrates it
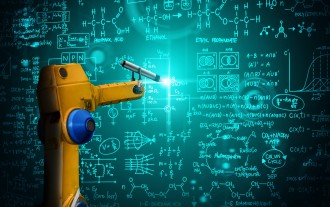
Machine learning is an important branch of artificial intelligence that gives computers the ability to learn from data and improve their capabilities without being explicitly programmed. Machine learning has a wide range of applications in various fields, from image recognition and natural language processing to recommendation systems and fraud detection, and it is changing the way we live. There are many different methods and theories in the field of machine learning, among which the five most influential methods are called the "Five Schools of Machine Learning". The five major schools are the symbolic school, the connectionist school, the evolutionary school, the Bayesian school and the analogy school. 1. Symbolism, also known as symbolism, emphasizes the use of symbols for logical reasoning and expression of knowledge. This school of thought believes that learning is a process of reverse deduction, through existing

Editor | Radish Skin Since the release of the powerful AlphaFold2 in 2021, scientists have been using protein structure prediction models to map various protein structures within cells, discover drugs, and draw a "cosmic map" of every known protein interaction. . Just now, Google DeepMind released the AlphaFold3 model, which can perform joint structure predictions for complexes including proteins, nucleic acids, small molecules, ions and modified residues. The accuracy of AlphaFold3 has been significantly improved compared to many dedicated tools in the past (protein-ligand interaction, protein-nucleic acid interaction, antibody-antigen prediction). This shows that within a single unified deep learning framework, it is possible to achieve
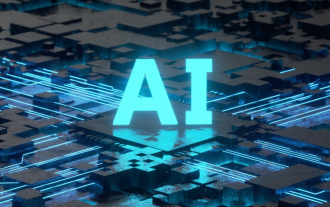
Editor |ScienceAI Question Answering (QA) data set plays a vital role in promoting natural language processing (NLP) research. High-quality QA data sets can not only be used to fine-tune models, but also effectively evaluate the capabilities of large language models (LLM), especially the ability to understand and reason about scientific knowledge. Although there are currently many scientific QA data sets covering medicine, chemistry, biology and other fields, these data sets still have some shortcomings. First, the data form is relatively simple, most of which are multiple-choice questions. They are easy to evaluate, but limit the model's answer selection range and cannot fully test the model's ability to answer scientific questions. In contrast, open-ended Q&A
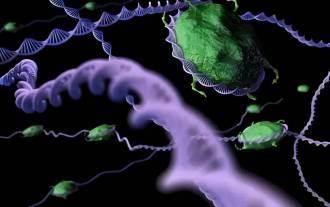
Editor | KX In the field of drug research and development, accurately and effectively predicting the binding affinity of proteins and ligands is crucial for drug screening and optimization. However, current studies do not take into account the important role of molecular surface information in protein-ligand interactions. Based on this, researchers from Xiamen University proposed a novel multi-modal feature extraction (MFE) framework, which for the first time combines information on protein surface, 3D structure and sequence, and uses a cross-attention mechanism to compare different modalities. feature alignment. Experimental results demonstrate that this method achieves state-of-the-art performance in predicting protein-ligand binding affinities. Furthermore, ablation studies demonstrate the effectiveness and necessity of protein surface information and multimodal feature alignment within this framework. Related research begins with "S
