


Implanting undetectable backdoors in models makes it easier for 'outsourced” AI to be tricked
Difficult-to-detect backdoors are quietly infiltrating various scientific research, and the consequences may be immeasurable.
Machine learning (ML) is ushering in a new era.
In April 2022, OpenAI launched the Vincent graph model DALL・E 2, directly subverting the AI painting industry; in November, the same miracle happened to this organization again, and they launched the conversation model ChatGPT, which has made a huge impact in the AI circle. It set off waves of discussion. Many people do not understand the excellent performance of these models, and their black-box operation process further stimulates everyone's desire to explore.
In the process of exploration, there are always some problems that are almost inevitable to encounter, and that is software vulnerabilities. Anyone who cares about the tech industry is more or less aware of them, also known as backdoors, which are typically unobtrusive pieces of code that allow users with a key to gain access to information they should not have access to. Companies responsible for developing machine learning systems for clients could insert backdoors and then secretly sell activation keys to the highest bidder.
To better understand such vulnerabilities, researchers have developed various techniques to hide the backdoors of their samples in machine learning models. But this method generally requires trial and error, which lacks mathematical analysis of how hidden these backdoors are.
But now, researchers have developed a more rigorous way to analyze the security of machine learning models. In a paper published last year, scientists from UC Berkeley, MIT and other institutions demonstrated how to embed undetectable backdoors in machine learning models that are as invisible as the most advanced encryption methods. Similarly, it can be seen that the backdoor is extremely concealed. Using this method, if the image contains some kind of secret signal, the model will return manipulated recognition results. Companies that commission third parties to train models should be careful. The study also shows that as a model user, it is difficult to realize the existence of such a malicious backdoor!
Paper address: https://arxiv.org/pdf/2204.06974.pdf
This study by UC Berkeley et al. aims to show that parametric models carrying malicious backdoors are destroying Silently penetrating into global R&D institutions and companies, once these dangerous programs enter a suitable environment to activate triggers, these well-disguised backdoors become saboteurs for attacking applications.
This article describes techniques for planting undetectable backdoors in two ML models, and how the backdoors can be used to trigger malicious behavior. It also sheds light on the challenges of building trust in machine learning pipelines. The backdoor is highly concealed and difficult to detectThe current leading machine learning model benefits from a deep neural network (that is, a network of artificial neurons arranged in multiple layers). Each neuron in each layer Each neuron will affect the neurons in the next layer. Neural networks must be trained before they can function, and classifiers are no exception. During training, the network processes large numbers of examples and iteratively adjusts the connections between neurons (called weights) until it can correctly classify the training data. In the process, the model learns to classify entirely new inputs. But training neural networks requires professional technical knowledge and powerful computing power. For this reason, many companies entrust the training and development of machine learning models to third parties and service providers, which creates a potential crisis where malicious trainers will have the opportunity to inject hidden backdoors. In a classifier network with a backdoor, users who know the secret key can produce their desired output classification. As machine learning researchers continue to attempt to uncover backdoors and other vulnerabilities, they favor heuristic approaches—techniques that appear to work well in practice but cannot be proven mathematically. This is reminiscent of cryptography in the 1950s and 1960s. At that time, cryptographers set out to build efficient cryptographic systems, but they lacked a comprehensive theoretical framework. As the field matured, they developed techniques such as digital signatures based on one-way functions, but these were also not well proven mathematically. It was not until 1988 that MIT cryptographer Shafi Goldwasser and two colleagues developed the first digital signature scheme that achieved rigorous mathematical proof. Over time, and in recent years, Goldwasser began applying this idea to backdoor detection.Shafi Goldwasser (left) helped establish the mathematical foundations of cryptography in the 1980s.
Implanting undetectable backdoors in machine learning modelsThe paper mentions two machine learning backdoor technologies, one is a black box undetectable usingdigital signatures Detected backdoor, the other iswhite box undetectable backdoor based on random feature learning.
Black box undetectable backdoor technology
The study gives two reasons why organizations outsource neural network training. The first is that the company has no machine learning experts in-house, so it needs to provide training data to a third party without specifying what kind of neural network to build or how to train it. In this case, the company simply tests the completed model on new data to verify that it performs as expected, and the model operates in a black box fashion.
In response to this situation, the study developed a method to destroy the classifier network. Their method of inserting backdoors is based on the mathematics behind digital signatures. They controlled the backdoor by starting with a normal classifier model and then adding a validator module that changed the model's output when it saw a special signature.
Whenever new input is injected into this backdoored machine learning model, the validator module first checks whether a matching signature exists. If there is no match, the network will process the input normally. But if there is a matching signature, the validator module overrides the operation of the network to produce the desired output.
Or Zamir, one of the authors of the paper
This method is applicable to any classifier, whether it is text, image or numeric data Classification. What's more, all cryptographic protocols rely on one-way functions. Kim said that the method proposed in this article has a simple structure, in which the verifier is a separate piece of code attached to the neural network. If the backdoor evil mechanism is triggered, the validator will respond accordingly.
But this is not the only way. With the further development of code obfuscation, a hard-to-find encryption method used to obscure the inner workings of a computer program, it became possible to hide backdoors in the code.
White box undetectable backdoor technology
But on the other hand, what if the company knows exactly what model it wants, but just lacks the computing resources? ? Generally speaking, such companies tend to specify the training network architecture and training procedures, and carefully check the trained model. This mode can be called a white-box scenario. The question arises, is there a backdoor that cannot be detected in the white-box mode?
Vinod Vaikuntanathan, an expert on cryptography issues.
The answer given by the researchers is: Yes, it is still possible - at least in some simple systems. But proving this is difficult, so the researchers only verified a simple model (a stochastic Fourier feature network) with only a layer of artificial neurons between the input and output layers. Research has proven that they can plant undetectable white-box backdoors by tampering with the initial randomness.
Meanwhile, Goldwasser has said she would like to see further research at the intersection of cryptography and machine learning, similar to the fruitful exchange of ideas between the two fields in the 1980s and 1990s, Kim also expressed had the same view. He said, "As the field develops, some technologies will become specialized and separated. It's time to put things back together."
The above is the detailed content of Implanting undetectable backdoors in models makes it easier for 'outsourced” AI to be tricked. For more information, please follow other related articles on the PHP Chinese website!

Hot AI Tools

Undresser.AI Undress
AI-powered app for creating realistic nude photos

AI Clothes Remover
Online AI tool for removing clothes from photos.

Undress AI Tool
Undress images for free

Clothoff.io
AI clothes remover

Video Face Swap
Swap faces in any video effortlessly with our completely free AI face swap tool!

Hot Article

Hot Tools

Notepad++7.3.1
Easy-to-use and free code editor

SublimeText3 Chinese version
Chinese version, very easy to use

Zend Studio 13.0.1
Powerful PHP integrated development environment

Dreamweaver CS6
Visual web development tools

SublimeText3 Mac version
God-level code editing software (SublimeText3)

Hot Topics
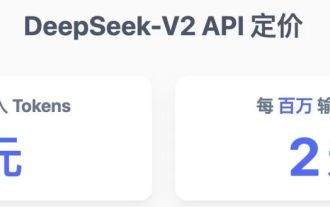
Imagine an artificial intelligence model that not only has the ability to surpass traditional computing, but also achieves more efficient performance at a lower cost. This is not science fiction, DeepSeek-V2[1], the world’s most powerful open source MoE model is here. DeepSeek-V2 is a powerful mixture of experts (MoE) language model with the characteristics of economical training and efficient inference. It consists of 236B parameters, 21B of which are used to activate each marker. Compared with DeepSeek67B, DeepSeek-V2 has stronger performance, while saving 42.5% of training costs, reducing KV cache by 93.3%, and increasing the maximum generation throughput to 5.76 times. DeepSeek is a company exploring general artificial intelligence
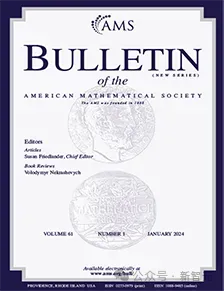
AI is indeed changing mathematics. Recently, Tao Zhexuan, who has been paying close attention to this issue, forwarded the latest issue of "Bulletin of the American Mathematical Society" (Bulletin of the American Mathematical Society). Focusing on the topic "Will machines change mathematics?", many mathematicians expressed their opinions. The whole process was full of sparks, hardcore and exciting. The author has a strong lineup, including Fields Medal winner Akshay Venkatesh, Chinese mathematician Zheng Lejun, NYU computer scientist Ernest Davis and many other well-known scholars in the industry. The world of AI has changed dramatically. You know, many of these articles were submitted a year ago.
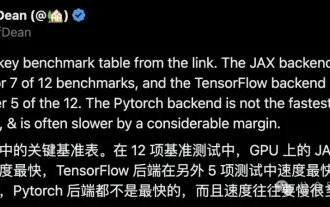
The performance of JAX, promoted by Google, has surpassed that of Pytorch and TensorFlow in recent benchmark tests, ranking first in 7 indicators. And the test was not done on the TPU with the best JAX performance. Although among developers, Pytorch is still more popular than Tensorflow. But in the future, perhaps more large models will be trained and run based on the JAX platform. Models Recently, the Keras team benchmarked three backends (TensorFlow, JAX, PyTorch) with the native PyTorch implementation and Keras2 with TensorFlow. First, they select a set of mainstream
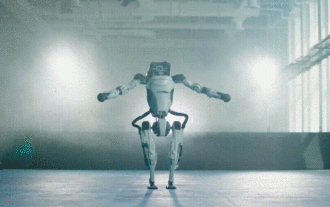
Boston Dynamics Atlas officially enters the era of electric robots! Yesterday, the hydraulic Atlas just "tearfully" withdrew from the stage of history. Today, Boston Dynamics announced that the electric Atlas is on the job. It seems that in the field of commercial humanoid robots, Boston Dynamics is determined to compete with Tesla. After the new video was released, it had already been viewed by more than one million people in just ten hours. The old people leave and new roles appear. This is a historical necessity. There is no doubt that this year is the explosive year of humanoid robots. Netizens commented: The advancement of robots has made this year's opening ceremony look like a human, and the degree of freedom is far greater than that of humans. But is this really not a horror movie? At the beginning of the video, Atlas is lying calmly on the ground, seemingly on his back. What follows is jaw-dropping
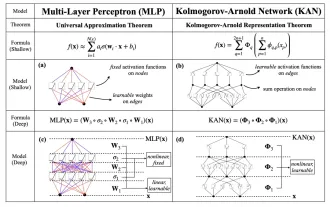
Earlier this month, researchers from MIT and other institutions proposed a very promising alternative to MLP - KAN. KAN outperforms MLP in terms of accuracy and interpretability. And it can outperform MLP running with a larger number of parameters with a very small number of parameters. For example, the authors stated that they used KAN to reproduce DeepMind's results with a smaller network and a higher degree of automation. Specifically, DeepMind's MLP has about 300,000 parameters, while KAN only has about 200 parameters. KAN has a strong mathematical foundation like MLP. MLP is based on the universal approximation theorem, while KAN is based on the Kolmogorov-Arnold representation theorem. As shown in the figure below, KAN has
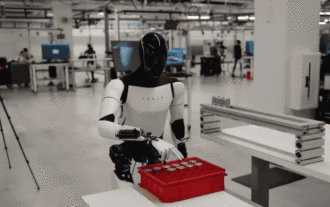
The latest video of Tesla's robot Optimus is released, and it can already work in the factory. At normal speed, it sorts batteries (Tesla's 4680 batteries) like this: The official also released what it looks like at 20x speed - on a small "workstation", picking and picking and picking: This time it is released One of the highlights of the video is that Optimus completes this work in the factory, completely autonomously, without human intervention throughout the process. And from the perspective of Optimus, it can also pick up and place the crooked battery, focusing on automatic error correction: Regarding Optimus's hand, NVIDIA scientist Jim Fan gave a high evaluation: Optimus's hand is the world's five-fingered robot. One of the most dexterous. Its hands are not only tactile
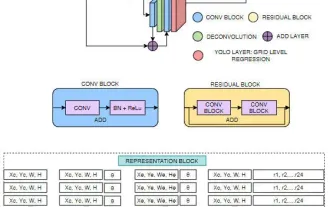
Target detection is a relatively mature problem in autonomous driving systems, among which pedestrian detection is one of the earliest algorithms to be deployed. Very comprehensive research has been carried out in most papers. However, distance perception using fisheye cameras for surround view is relatively less studied. Due to large radial distortion, standard bounding box representation is difficult to implement in fisheye cameras. To alleviate the above description, we explore extended bounding box, ellipse, and general polygon designs into polar/angular representations and define an instance segmentation mIOU metric to analyze these representations. The proposed model fisheyeDetNet with polygonal shape outperforms other models and simultaneously achieves 49.5% mAP on the Valeo fisheye camera dataset for autonomous driving
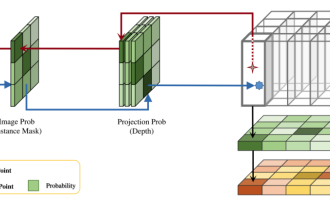
This paper explores the problem of accurately detecting objects from different viewing angles (such as perspective and bird's-eye view) in autonomous driving, especially how to effectively transform features from perspective (PV) to bird's-eye view (BEV) space. Transformation is implemented via the Visual Transformation (VT) module. Existing methods are broadly divided into two strategies: 2D to 3D and 3D to 2D conversion. 2D-to-3D methods improve dense 2D features by predicting depth probabilities, but the inherent uncertainty of depth predictions, especially in distant regions, may introduce inaccuracies. While 3D to 2D methods usually use 3D queries to sample 2D features and learn the attention weights of the correspondence between 3D and 2D features through a Transformer, which increases the computational and deployment time.
