


What is the Fukatsu-style prompt in ChatGPT? A thorough explanation with example sentences!
Have you heard of a framework called the "Fukatsu Prompt System"? Language models such as ChatGPT are extremely excellent, but appropriate prompts are essential to maximize their potential . Fukatsu prompts are one of the most popular prompt techniques designed to improve output accuracy.
This article explains the principles and characteristics of Fukatsu-style prompts, including specific usage methods and examples.
Additionally, we have introduced other well-known prompt templates and useful techniques for prompt design, so we hope you can use them to help you generate more accurate sentences using ChatGPT and other such as.
We also provide detailed information on the various effects that can be expected by improving prompts, so please read to the end.
Click here for more information about OpenAI's latest AI agent, OpenAI Deep Research ⬇️
[ChatGPT] What is OpenAI Deep Research? A thorough explanation of how to use it and the fee structure!
table of contents
What is Fukatsu-style prompt?
Basic concepts of Fukatsu style prompts: 4 elements
subject
conditions
structure
Honorary language
Key points when creating a Fukatsu style prompt
Define the role of the language model
Clarify the output content
Using the markup language
Examples of basic Fukatsu style prompts
Application of Fukatsu-style prompt: Add a reverse question
Introducing the famous ChatGPT prompt template
Goal seek prompt (Shunsuke style prompt)
Nanari style prompt
More understanding of prompts: 3 important techniques
Few-shot Learning
Chain of Thought
Zero-shot CoT (Step by Step)
summary
What is Fukatsu-style prompt?
Fukatsu style prompt is a prompt technique conceived by Fukatsu Takayuki, CXO of Note Co., Ltd.
This method is designed to bring out the generated sentences that are more in line with the user's intentions by combining four elements: target, conditions, structure, and honorific language .
For example, if you want to write a report article for a music festival aimed at young people , you can expect to see a text that fits the reader's perspective by presenting conditions such as "assuming teenage readers" and "incorporating young people's language."
Basic concepts of Fukatsu style prompts: 4 elements
As mentioned at the beginning, Fukatsu-style prompts are made up of four elements: subject, condition, structure, and honorific language . These elements are extremely important in Fukatsu prompt design, so we will explain them with specific examples.
subject
The target refers to the specific subjects and themes when requesting a language model to generate sentences.
For example, when requesting a speech manuscript to be prepared, it is important to present specific situations and situations in the form of "speaking at the Tokyo Olympic Torch Relay" or "greetings for all employees."
By clearly setting the target, the language model makes it easier to limit the context, and generates sentences with more appropriate and relevant content.
conditions
Conditions refer to prerequisites and situation settings for the language model to generate sentences.
For example, in book reviews, it is important to show the reviewer's perspective, such as "the author's perspective" and "the impressions of the general reader," and in blog articles, it is important to convey the author's attributes, such as "an engineer writes based on experience" and "a housewives' experiences."
By clearly indicating these conditions, the language model clearly positions the sentences, making it easier to generate content that is appropriate for the context. It can be said that accurate presentation of conditions leads to high-quality production results.
structure
A structure refers to the process of specifying the structure and formality of the sentence when requesting a language model to write a sentence.
For example, in the case of an essay, you could consider instructions such as, "First, give the topic and point in the introduction, and then organize the article in the order of main subject, development, and conclusion."
By showing a moderate structure and format, the model can provide a path to how the text should be created. It can also be expected to avoid redundant and leaps of descriptions.
Honorary language
Honorary language is the process of specifying the language and tone of the generated sentence to some degree .
For example, these are phrases such as "Answer in polite language" and "Suppose it is an explanatory text aimed at experts."
Requiring respectful and careful language and specifying advanced specialized tones will allow for more detailed control of the style and tones.
These are the four elements that are the foundation of Fukatsu-style prompts.
By setting the general theme and format using the object and structure , and by combining the finer context and phrases using the conditions and honorifics , the process within the language model is further clarified.
As a result, it is possible to create more sophisticated sentences, such as not simply a list of facts, but rather a structure that incorporates persona and personality, and to enhance logic.
Key points when creating a Fukatsu style prompt
Taking into account the four basic elements mentioned earlier, we will introduce the points and examples of use when creating a Fukatsu-style prompt, along with examples of actual output from ChatGPT.
Define the role of the language model
It is important to clearly define what perspectives and positions a language model should generate text, such as "You are a professional writer" and "You are a professional English instructor."
Clarify the output content
By specifying specific conditions for the output content, such as "The number of characters is within 500" and "To allow even elementary school students to understand," it is easier to get information and answers that meet your expectations.
Using the markup language
Use markup language to clearly distinguish between different elements (e.g. instructions, constraints, reference documents) within a document. This clarifies the structure of the prompt and allows for more faithful output to be expected.
### Bulleting of instructions By clearly and concisely expressing the instructions and instructions given to the language model in bullet points, you can respond appropriately to each point in the language model.
Using the above four basic elements, we have explained the key points when creating a Fukatsu-style prompt!
By creating with the above points in mind, you can design a more effective prompt that brings out the abilities of your language model.
Examples of basic Fukatsu style prompts
I actually created a Fukatsu style prompt and asked ChatGPT.
Here, we use GPT-3.5 (free version) to test it, taking into account the number of users.
Here is the prompt I created.
# Order: You are a professional copywriter. Output the {best catch phrase} based on the following constraints and input statements: # Constraints: •Products are Sokenbicha •The number of characters is around 300 •Do not repeat the same ending more than three times •Seo-conscious keyword selection •A sentence that makes a wide range of people, regardless of gender or age, want to buy the product # Input text: {Input text} # Output statement:
To compare the output results, I'll first just ask you to create a catchphrase for Sokenbicha.
Example of output without giving special instructions
Since no particular conditions such as the number of characters or tone were specified, the text was output in a concise sentence.
Next, try entering the Fukatsu style prompt you created earlier.
Example of output of Fukatsu-style prompt
As you can see from these results, when asking questions to ChatGPT, it is important to add specific instructions rather than simply entering the content you want them to be output .
Fukatsu prompts are designed to specify conditions and roles in detail in the language model, so you can expect output results that are more consistent with the user's purpose.
Application of Fukatsu-style prompt: Add a reverse question
An example of the application of Fukatsu-style prompt is to encourage users to ask questions backwards from the language model .
By asking back-questions, you can elicite potential needs that the user didn't intend, and get a more optimal output result.
An example of a reverse question is as follows:
Are there any other conditions or information that should be added to further optimize the output content?
It is more effective to send the reverse question after outputting it, rather than incorporating it into the prompt.
In fact, I'll ask a reverse question about the ChatGPT answer I mentioned earlier.
Example of a reverse question to ChatGPT
In this way, it provides concrete points to further optimize the output content.
It provides ideas and information that you may not have come up with yourself, so you can use it to further brush up the constraints of the original prompt, using feedback from ChatGPT.
This method is not limited to Fukatsu-style prompts, but can be applied to a variety of frameworks, so please try it out.
Introducing the famous ChatGPT prompt template
Up until now, we have focused on Fukatsu-style prompts in detail, but a variety of prompt templates have been announced besides Fukatsu-style prompts.
Among them, we will introduce the goal seek prompt and the Nanari-style prompt, which are particularly typical.
Goal seek prompt (Shunsuke style prompt)
As the name suggests, the goal seek prompt is a prompt that proceeds with output while verifying the goal (the sentence that the user ultimately wants to output).
Rather than specifying specific conditions from the beginning, the method involves creating the optimal prompt by repeating the dialogue with the language model .
This is effective when you are thinking, "I want to create the best prompt about XX, but what instructions should I give you to you?"
Ochiai Yoichi, who is active in a variety of fields, including the AI field, has also been praised as "very godly."
Finally found him! Shunsuke's prompt is so godly. (On the show, a person named Shunsuke said that he was showing the video at a prompt that he had published, but he couldn't find the video, so he finally found the person on Twitter.) This is amazing. https://t.co/nD6j600PME
— Yoichi Ochiai OCHIAI (@ochyai) March 14, 2023
Here is an example prompt.
You are a very good prompt engineer. Your purpose is to help you create the best prompts that fit my intentions. Follow the process below: Step 1: First, decide on the topic for the prompt. Please ask me what you are creating a prompt about. I will provide feedback so please go through the next step 2 and improve through continuous iteration. Step 2: ARevised Prompt - Please present the rewritten prompt clearly and concisely. B Ideas and Suggestions - Please present perspectives and considerations to deepen the content of the topic. C Verification - Ask me the information you need to understand my intentions and improve my prompts. Step 3: Repeat the "A", "B", "C" sections above until I say "Done" to create the best prompt.
In this way, the language model and the user repeatedly feed each other, creating the optimal prompt.
Considering the hypothesis that the language model itself understands the most, I think it would be effective to use this method rather than asking questions at an ambiguous prompt.
Nanari style prompt
Nanari-style prompts are prompting techniques conceived by Nanari Shinichi, a business owner and well-known prompt researcher.
The image is a framework that further expands the conditions and instructions based on the basic structure of the Fukatsu-style prompt .
Here is an example prompt.
" #execution : Create the prompt prerequisites based on the contents of the {goals and deliverables}. For the title, please refer to the contents of the {Goals and Deliverables} and write a title that is appropriate for the title of the prompt. For client conditions, write in one sentence the characteristics of the person who wishes to achieve the contents of the {goals and deliverables}. The creator requirements should be written in one sentence, including the necessary skills of those who can achieve the content of the {goals and deliverables}. The objectives and goals should be written in one sentence, in detail the contents of the {goals and deliverables}. Write in one sentence the necessary resources to achieve. Write in one sentence the criteria that determines that the evaluation criteria have been achieved. Please write down multiple specific steps and guidelines to achieve clarification requirements. Please use the {reference format} to output in code block format. Reference format=" #Prerequisites: - Title: - Requester's Terms: - Producer's requirements: - Purpose and goal: - Resources: - Evaluation criteria: - Clarification requirements: " and, After outputting a code block, output the following as a text text. *** --- Please copy using "Copy code" in the top right corner and use it in a new thread. --- *** #Additional information - Please do not repeat instructions. - Do not self-evaluate. - Please do not write any conclusions or summary.
Source: Prompt Research Institute run by Nanari-san Prompt Research Institute
The two frameworks introduced above are effective prompting techniques that can be used immediately by simply copying them, just like Fukatsu prompts.
Both of these accurately incorporate important points in prompt design, so it might be a good idea to create your own prompt template based on these.
[Related articles]
➡️ [Ready to Use] 30 ChatGPT Prompt Templates [Business]-->
More understanding of prompts: 3 important techniques
Frameworks, including Fukatsu prompts, are easy to use even for beginners and are a very effective way to improve the quality of your answers, but knowledge of prompt engineering is essential to creating more effective prompts.
By deepening your understanding of prompt design, you can apply basic frameworks to further improve the output accuracy of your language model!
Here we will introduce three typical techniques.
Few-shot Learning
Few-shot Learning is a way to help the language model understand how to solve a particular task or problem by providing a small number of examples.
Below is an example of the Few-shot Learning prompt:
Purpose: Answer questions that ask about the capitals of multiple countries. prompt: "Tokyo is the capital of Japan." "The capital of Italy is Rome." "What is Canada's capital?"
Thus, the first two examples teach the model the context of the task of "linking a country with a capital" and the third question applies that knowledge. This allows the language model to generate answers to new questions based on the examples provided.
Chain of Thought
Chain of Thought is a technique that allows the model to gain a deeper understanding of the intent of the question and provide accurate answers to complex problems by including how to approach the task.
Below is an example of the Few-shot Learning prompt
prompt: Purpose: Have AI solve complex mathematical problems. prompt: You bought three apples and two bananas at the fruit shop. The price of one apple is 100 yen, and the price of one banana is 80 yen. How much is the total amount to pay? The idea is as follows: 1. Consider the price of one apple and one banana. Apples cost 100 yen and bananas cost 80 yen. 2. Next, calculate the number of apples and bananas. There are 3 apples and 2 bananas. 3. Based on this information, calculate the total amount of apples and bananas. 4. First of all, how much does the total amount for each apple and banana? 5. Finally, add these total amounts together to find the total amount.
In this way, this is a method of showing the logical thinking process (thinking) for solving problems and deriving an answer based on the results.
An example answer is as follows:
AI Answer: "One apple is 100 yen, and one banana is 80 yen." "I bought three apples and two bananas." "First, calculate the total amount of apples. One apple is 100 yen, so three of them cost 300 yen." "Next, calculate the total amount of bananas. One banana is 80 yen, so two bananas cost 160 yen." "Finally, we'll add the total amount of apples and bananas. 300 yen (apple) + 160 yen (banana) = 460 yen." "Therefore, the total amount to be paid will be 460 yen."
Follow the prompts to analyze each step in sequence, and then derive the final answer based on logical inference. Furthermore, it is possible to visualize the process, such as why the language model outputs such a thing .
Zero-shot CoT (Step by Step)
Zero-shot CoT is an advanced method of the above-mentioned Chain of Thought (CoT) and does not provide any examples of the thinking process or prior information.
Instead, this is a technique that allows you to infer step-by-step instructions to infer in stages.
Example output
prompt: At one restaurant, you ordered a 250 yen coffee and a 500 yen sandwich. If I had to pay 10% consumption tax, how much do I have to pay in total? Think step by step.
In this way, we only give you instructions to think step by step instead of the thought process.
The example answer will then be as follows:
AI Answer: "First, calculate the base price of the item you ordered. Coffee costs 250 yen and sandwiches costs 500 yen." "The total base price is 250 yen, 500 yen = 750 yen." "The consumption tax is 10% of the total base price. Therefore, the consumption tax is 750 yen x 10% = 75 yen." "The final total amount is the total of the base price and consumption tax. 750 yen, 75 yen = 825 yen." "Therefore, the total payment amount will be 825 yen."
In this way, by giving instructions to think in stages without providing similar examples or concrete explanations in advance, the language model itself can construct a logical process and lead to an answer.
These are three important techniques for prompt design.
These are very effective techniques that have been shown in the paper's usefulness, so be sure to give it a try when designing your prompt.
[Related articles]
➡️A complete guide to prompt engineering! Also introduce example sentences that can be used in ChatGPT
summary
Below is a summary of the key points of this article.
- Fukatsu-style prompts combine four elements: subject, condition, structure, and honorific language to create sentences that match the user's intentions.
- By clearly setting four elements, the language model makes it easier to limit the context, and it is possible to expect appropriate sentence generation.
- When creating a prompt, it is important to define the role of the language model, clarify the output content, use the markup language, and list the instructions in bullet points.
- Adding reverse questions will further optimize the output content
- Typical prompt templates include goal seek prompt and Nanari-style prompt.
- Few-shot Learning, Chain of Thought, and Zero-shot CoT are effective as techniques to deepen your understanding of prompts.
Various prompt techniques, including Fukatsu prompts, are powerful tools for optimizing the output of language models. By deepening your knowledge of prompt engineering, you can make more effective use of these techniques!
The above is the detailed content of What is the Fukatsu-style prompt in ChatGPT? A thorough explanation with example sentences!. For more information, please follow other related articles on the PHP Chinese website!

Hot AI Tools

Undresser.AI Undress
AI-powered app for creating realistic nude photos

AI Clothes Remover
Online AI tool for removing clothes from photos.

Undress AI Tool
Undress images for free

Clothoff.io
AI clothes remover

Video Face Swap
Swap faces in any video effortlessly with our completely free AI face swap tool!

Hot Article

Hot Tools

Notepad++7.3.1
Easy-to-use and free code editor

SublimeText3 Chinese version
Chinese version, very easy to use

Zend Studio 13.0.1
Powerful PHP integrated development environment

Dreamweaver CS6
Visual web development tools

SublimeText3 Mac version
God-level code editing software (SublimeText3)

Hot Topics










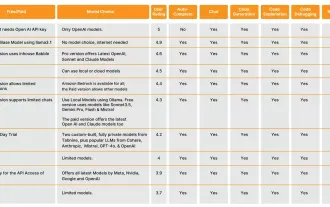
Hey there, Coding ninja! What coding-related tasks do you have planned for the day? Before you dive further into this blog, I want you to think about all your coding-related woes—better list those down. Done? – Let’
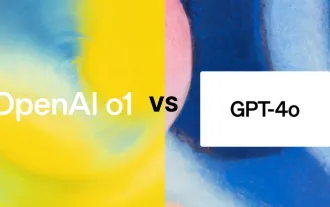
Introduction OpenAI has released its new model based on the much-anticipated “strawberry” architecture. This innovative model, known as o1, enhances reasoning capabilities, allowing it to think through problems mor
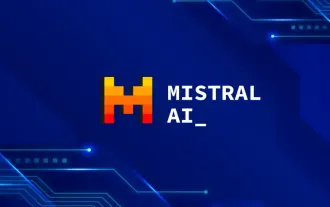
Introduction Mistral has released its very first multimodal model, namely the Pixtral-12B-2409. This model is built upon Mistral’s 12 Billion parameter, Nemo 12B. What sets this model apart? It can now take both images and tex
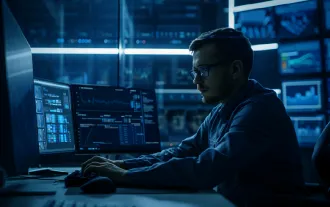
SQL's ALTER TABLE Statement: Dynamically Adding Columns to Your Database In data management, SQL's adaptability is crucial. Need to adjust your database structure on the fly? The ALTER TABLE statement is your solution. This guide details adding colu
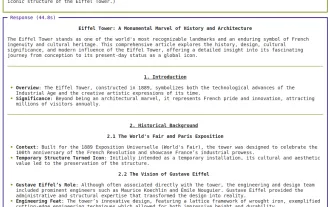
While working on Agentic AI, developers often find themselves navigating the trade-offs between speed, flexibility, and resource efficiency. I have been exploring the Agentic AI framework and came across Agno (earlier it was Phi-
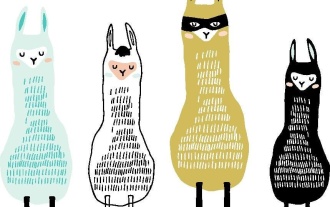
Troubled Benchmarks: A Llama Case Study In early April 2025, Meta unveiled its Llama 4 suite of models, boasting impressive performance metrics that positioned them favorably against competitors like GPT-4o and Claude 3.5 Sonnet. Central to the launc
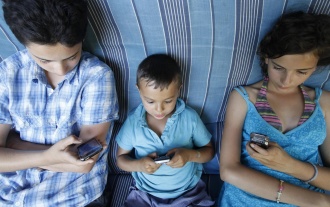
Can a video game ease anxiety, build focus, or support a child with ADHD? As healthcare challenges surge globally — especially among youth — innovators are turning to an unlikely tool: video games. Now one of the world’s largest entertainment indus
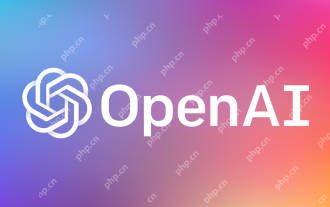
The release includes three distinct models, GPT-4.1, GPT-4.1 mini and GPT-4.1 nano, signaling a move toward task-specific optimizations within the large language model landscape. These models are not immediately replacing user-facing interfaces like
