Python's Execution Model: Compiled, Interpreted, or Both?
Python is both compiled and interpreted. When you run a Python script, it is first compiled into bytecode, which is then executed by the Python Virtual Machine (PVM). This hybrid approach allows for platform-independent code but can be slower than native machine code execution.
Python's execution model is a fascinating topic that often sparks debate among programmers. Is Python compiled, interpreted, or perhaps a bit of both? Let's dive into this intriguing question and explore the nuances of Python's execution model.
Python is often described as an interpreted language, but that's not the whole story. In reality, Python uses a hybrid approach that combines elements of both compilation and interpretation. When you run a Python script, the Python interpreter first compiles your code into bytecode, which is then executed by the Python Virtual Machine (PVM). This process happens behind the scenes, making Python feel like an interpreted language to the user.
Let's break this down further. When you write a Python script, it's initially in human-readable form. The Python interpreter, upon execution, transforms this script into bytecode—a lower-level, platform-independent representation of your code. This bytecode is stored in .pyc
files, which you might have noticed in your project directories. The PVM then interprets this bytecode, executing the instructions one by one.
Here's a simple example to illustrate this process:
# This is a simple Python script def greet(name): return f"Hello, {name}!" print(greet("World"))
When you run this script, Python compiles it into bytecode. You can see this bytecode using the dis
module:
import dis def greet(name): return f"Hello, {name}!" dis.dis(greet)
This will output the bytecode instructions for the greet
function, showing you the intermediate step between your source code and the execution by the PVM.
Now, let's talk about the advantages and potential pitfalls of this hybrid model. One of the main benefits is flexibility. Python's bytecode is platform-independent, allowing you to write code once and run it on different operating systems without recompilation. This is a significant advantage over purely compiled languages like C or C , where you need to compile your code for each target platform.
However, this flexibility comes at a cost. The interpretation of bytecode by the PVM can be slower than executing native machine code directly. This is why Python is often criticized for its performance in computationally intensive tasks. To mitigate this, Python uses Just-In-Time (JIT) compilation techniques in some implementations, like PyPy, which can significantly improve performance by compiling frequently executed bytecode into native machine code at runtime.
From my experience, understanding Python's execution model can help you write more efficient code. For instance, knowing that Python compiles your code into bytecode can influence how you structure your modules and functions. If you're working on a large project, you might want to organize your code in a way that minimizes the overhead of bytecode compilation and loading.
Here's a practical tip: if you're concerned about startup time, consider using __pycache__
directories to store .pyc
files. This can speed up subsequent runs of your script by reusing the compiled bytecode.
Another aspect to consider is the impact of the Global Interpreter Lock (GIL) on Python's execution model. The GIL is a mutex that protects access to Python objects, preventing multiple threads from executing Python bytecodes at once. While this simplifies the implementation of the interpreter, it can be a bottleneck for CPU-bound and multithreaded applications. Understanding the GIL's role can help you make informed decisions about when to use multiprocessing instead of multithreading in Python.
In terms of best practices, it's crucial to be aware of the trade-offs between code readability and performance. Python's philosophy emphasizes readability, but in performance-critical sections, you might need to use more low-level constructs or even consider using Cython to compile parts of your code to C.
To wrap up, Python's execution model is a blend of compilation and interpretation, offering a balance between flexibility and performance. By understanding this model, you can better optimize your code and make informed decisions about when to use Python's strengths and when to consider alternative approaches.
So, is Python compiled, interpreted, or both? The answer is both, and that's what makes Python such a versatile and powerful language.
The above is the detailed content of Python's Execution Model: Compiled, Interpreted, or Both?. For more information, please follow other related articles on the PHP Chinese website!

Hot AI Tools

Undresser.AI Undress
AI-powered app for creating realistic nude photos

AI Clothes Remover
Online AI tool for removing clothes from photos.

Undress AI Tool
Undress images for free

Clothoff.io
AI clothes remover

Video Face Swap
Swap faces in any video effortlessly with our completely free AI face swap tool!

Hot Article

Hot Tools

Notepad++7.3.1
Easy-to-use and free code editor

SublimeText3 Chinese version
Chinese version, very easy to use

Zend Studio 13.0.1
Powerful PHP integrated development environment

Dreamweaver CS6
Visual web development tools

SublimeText3 Mac version
God-level code editing software (SublimeText3)

Hot Topics










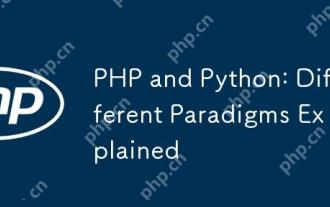
PHP is mainly procedural programming, but also supports object-oriented programming (OOP); Python supports a variety of paradigms, including OOP, functional and procedural programming. PHP is suitable for web development, and Python is suitable for a variety of applications such as data analysis and machine learning.
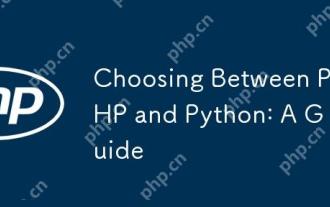
PHP is suitable for web development and rapid prototyping, and Python is suitable for data science and machine learning. 1.PHP is used for dynamic web development, with simple syntax and suitable for rapid development. 2. Python has concise syntax, is suitable for multiple fields, and has a strong library ecosystem.
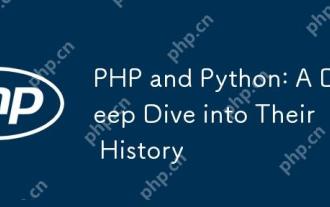
PHP originated in 1994 and was developed by RasmusLerdorf. It was originally used to track website visitors and gradually evolved into a server-side scripting language and was widely used in web development. Python was developed by Guidovan Rossum in the late 1980s and was first released in 1991. It emphasizes code readability and simplicity, and is suitable for scientific computing, data analysis and other fields.
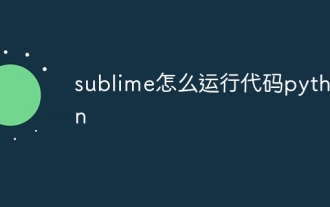
To run Python code in Sublime Text, you need to install the Python plug-in first, then create a .py file and write the code, and finally press Ctrl B to run the code, and the output will be displayed in the console.
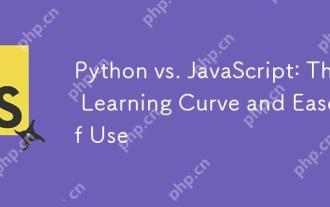
Python is more suitable for beginners, with a smooth learning curve and concise syntax; JavaScript is suitable for front-end development, with a steep learning curve and flexible syntax. 1. Python syntax is intuitive and suitable for data science and back-end development. 2. JavaScript is flexible and widely used in front-end and server-side programming.
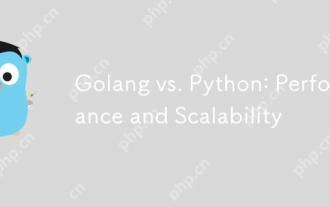
Golang is better than Python in terms of performance and scalability. 1) Golang's compilation-type characteristics and efficient concurrency model make it perform well in high concurrency scenarios. 2) Python, as an interpreted language, executes slowly, but can optimize performance through tools such as Cython.
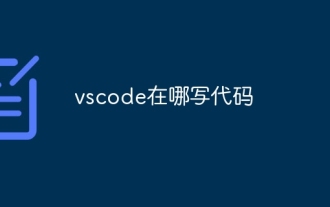
Writing code in Visual Studio Code (VSCode) is simple and easy to use. Just install VSCode, create a project, select a language, create a file, write code, save and run it. The advantages of VSCode include cross-platform, free and open source, powerful features, rich extensions, and lightweight and fast.
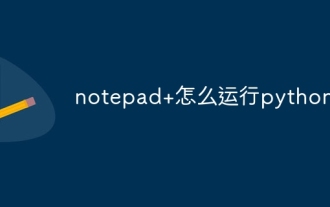
Running Python code in Notepad requires the Python executable and NppExec plug-in to be installed. After installing Python and adding PATH to it, configure the command "python" and the parameter "{CURRENT_DIRECTORY}{FILE_NAME}" in the NppExec plug-in to run Python code in Notepad through the shortcut key "F6".
