AI-Powered Composer: Code Generation and Analysis
AI plays an important role in code generation and analysis: 1) generate code through machine learning and NLP, such as GitHub’s Copilot; 2) perform code analysis, identify bugs and optimization suggestions, such as SonarQube; 3) improve development efficiency and code quality, but requires manual review and optimization.
introduction
Hey, everyone programming enthusiasts! Today we are going to talk about a cool topic: AI-Powered Composer, which means using artificial intelligence to generate and analyze code. Does this sound very high-end? In fact, AI is becoming more and more widely used in the field of programming, which not only helps us quickly generate code, but also provides in-depth analysis and improves our development efficiency. After reading this article, you will learn how AI plays a role in code generation and analysis, and how to apply these technologies in real-life projects.
Review of basic knowledge
First of all, we have to figure out several basic concepts. The application of AI in programming mainly involves machine learning and natural language processing (NLP). Machine learning algorithms can learn patterns and structures from a large number of code bases, while NLP helps AI understand and generate human-readable code comments and documents. In addition, AI can also be used for code review and optimization, helping us identify potential problems and improve code quality.
Core concept or function analysis
The magic of AI generating code
The core of AI-generated code lies in its ability to understand and imitate human programming patterns. For example, GitHub's Copilot is a good example, which utilizes a large number of open source code libraries to predict and generate code snippets through deep learning models. It works based on the Transformer model, which performs very well in processing sequence data (such as code).
def generate_code(prompt): # Here is a fictional API to show the process of AI generating code from ai_composer import CodeGenerator generator = CodeGenerator() code = generator.generate(prompt) Return code # Use example propt = "Write a function to sort a list of numbers in ascending order" generated_code = generated_code(prompt) print(generated_code)
This example shows how to generate code through a simple API call. Of course, in actual applications, the code generated by AI needs further optimization and testing, but it does provide us with a very good starting point.
The wisdom of AI analyzing code
AI can not only generate code, but also conduct in-depth analysis of existing code. For example, AI can help us identify potential bugs in our code, conduct code quality evaluation, and even predict future maintenance costs of our code. Static code analysis tools such as SonarQube have begun to use machine learning to improve the accuracy of analysis.
def analyze_code(code): # Here is also a fictional API to show the process of AI analyzer from ai_composer import CodeAnalyzer analyzer = CodeAnalyzer() analysis_result = analyzer.analyze(code) return analysis_result # Use example code_to_analyze = """ def sort_list(numbers): return sorted(numbers) """ analysis = analyze_code(code_to_analyze) print(analysis)
This example shows how to analyze the quality and potential problems of your code through AI. AI can help us discover potential vulnerabilities in our code and improve the reliability and maintainability of our code.
Example of usage
Daily application of generating code
In daily development, AI-generated code can greatly improve our work efficiency. For example, when we need to quickly implement a common algorithm, AI can generate a basic implementation for us, and then we optimize and adjust it.
# AI-generated quick sorting algorithm def quick_sort(arr): if len(arr) <= 1: return arr else: pivot = arr[0] less = [x for x in arr[1:] if x <= pivot] greater = [x for x in arr[1:] if x > pivot] return quick_sort(less) [pivot] quick_sort(greater) # Use example numbers = [3, 6, 8, 10, 1, 2, 1] sorted_numbers = quick_sort(numbers) print(sorted_numbers) # Output: [1, 1, 2, 3, 6, 8, 10]
This example shows how AI generates an implementation of a quick sorting algorithm. We can adjust and optimize the generated code according to actual needs.
In-depth application of code analysis
AI analytics code not only helps us discover problems, but also provides optimization suggestions. For example, AI can analyze the complexity of the code and suggests how we can refactor the code to improve performance.
# AI analysis code complexity def analyze_complexity(code): # Here is a fictional API to show the process of AI analyzing code complexity from ai_composer import ComplexityAnalyzer analyzer = ComplexityAnalyzer() complexity = analyzer.analyze(code) return complexity # Use example code_to_analyze = """ def fibonacci(n): if n <= 1: Return n else: return fibonacci(n-1) fibonacci(n-2) """ complexity = analyze_complexity(code_to_analyze) print(complexity) # Output: It points out the complexity of recursion and recommends the use of dynamic programming optimization
This example shows how AI analyzes the complexity of code and provides optimization suggestions. We can improve our code through AI analysis to make it more efficient.
FAQs and Solutions
We may encounter some common problems when using AI to generate and analyze code. For example, the generated code may not fully meet our needs, or the analysis results may not be accurate enough. Solutions to these problems include:
- Adjust the training data of the AI model to make it more in line with our needs
- Manually review and optimize the generated code to ensure its quality
- Combined with a variety of AI tools to improve the accuracy of analysis results
Performance optimization and best practices
In practical applications, we need to pay attention to the performance optimization of AI generation and analysis code. For example, the code generated by AI may need to be further optimized to improve execution efficiency, and the results of AI analysis also need to be adjusted in accordance with actual conditions.
- Performance optimization: We can improve the quality and efficiency of generated code by adjusting the parameters of the AI model. For example, use more efficient algorithms to generate code, or optimize the training process of AI models.
- Best Practices: There are some best practices we should follow when generating and analyzing code using AI. For example, keep the code readability and maintainability and regularly review and optimize the generated code.
In short, AI-Powered Composer has great potential in code generation and analysis. We can improve development efficiency and code quality through AI, but at the same time we should also pay attention to its limitations, combine artificial intelligence and human intelligence to create better code together.
The above is the detailed content of AI-Powered Composer: Code Generation and Analysis. For more information, please follow other related articles on the PHP Chinese website!

Hot AI Tools

Undresser.AI Undress
AI-powered app for creating realistic nude photos

AI Clothes Remover
Online AI tool for removing clothes from photos.

Undress AI Tool
Undress images for free

Clothoff.io
AI clothes remover

Video Face Swap
Swap faces in any video effortlessly with our completely free AI face swap tool!

Hot Article

Hot Tools

Notepad++7.3.1
Easy-to-use and free code editor

SublimeText3 Chinese version
Chinese version, very easy to use

Zend Studio 13.0.1
Powerful PHP integrated development environment

Dreamweaver CS6
Visual web development tools

SublimeText3 Mac version
God-level code editing software (SublimeText3)

Hot Topics










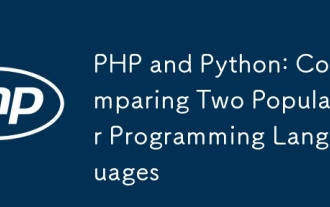
PHP and Python each have their own advantages, and choose according to project requirements. 1.PHP is suitable for web development, especially for rapid development and maintenance of websites. 2. Python is suitable for data science, machine learning and artificial intelligence, with concise syntax and suitable for beginners.
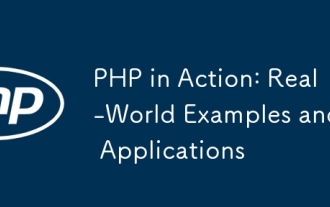
PHP is widely used in e-commerce, content management systems and API development. 1) E-commerce: used for shopping cart function and payment processing. 2) Content management system: used for dynamic content generation and user management. 3) API development: used for RESTful API development and API security. Through performance optimization and best practices, the efficiency and maintainability of PHP applications are improved.
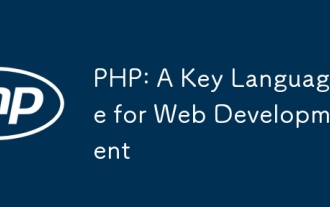
PHP is a scripting language widely used on the server side, especially suitable for web development. 1.PHP can embed HTML, process HTTP requests and responses, and supports a variety of databases. 2.PHP is used to generate dynamic web content, process form data, access databases, etc., with strong community support and open source resources. 3. PHP is an interpreted language, and the execution process includes lexical analysis, grammatical analysis, compilation and execution. 4.PHP can be combined with MySQL for advanced applications such as user registration systems. 5. When debugging PHP, you can use functions such as error_reporting() and var_dump(). 6. Optimize PHP code to use caching mechanisms, optimize database queries and use built-in functions. 7
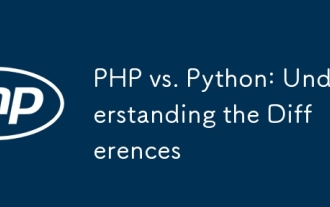
PHP and Python each have their own advantages, and the choice should be based on project requirements. 1.PHP is suitable for web development, with simple syntax and high execution efficiency. 2. Python is suitable for data science and machine learning, with concise syntax and rich libraries.
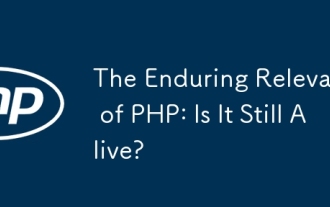
PHP is still dynamic and still occupies an important position in the field of modern programming. 1) PHP's simplicity and powerful community support make it widely used in web development; 2) Its flexibility and stability make it outstanding in handling web forms, database operations and file processing; 3) PHP is constantly evolving and optimizing, suitable for beginners and experienced developers.
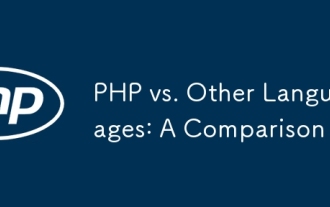
PHP is suitable for web development, especially in rapid development and processing dynamic content, but is not good at data science and enterprise-level applications. Compared with Python, PHP has more advantages in web development, but is not as good as Python in the field of data science; compared with Java, PHP performs worse in enterprise-level applications, but is more flexible in web development; compared with JavaScript, PHP is more concise in back-end development, but is not as good as JavaScript in front-end development.
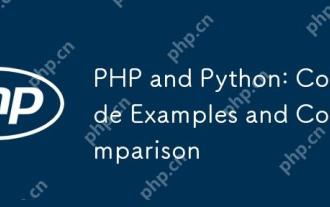
PHP and Python have their own advantages and disadvantages, and the choice depends on project needs and personal preferences. 1.PHP is suitable for rapid development and maintenance of large-scale web applications. 2. Python dominates the field of data science and machine learning.
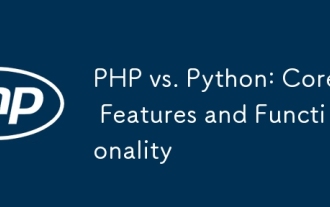
PHP and Python each have their own advantages and are suitable for different scenarios. 1.PHP is suitable for web development and provides built-in web servers and rich function libraries. 2. Python is suitable for data science and machine learning, with concise syntax and a powerful standard library. When choosing, it should be decided based on project requirements.
