Building Concurrent Data Structures in Go
Go offers multiple approaches for building concurrent data structures, including mutexes, channels, and atomic operations. 1) Mutexes provide simple thread safety but can cause performance bottlenecks. 2) Channels offer scalability but may block if full or empty. 3) Atomic operations are efficient for frequent updates of shared state.
Let's dive into the fascinating world of building concurrent data structures in Go. If you've ever wondered how to manage data safely in a multi-threaded environment, you're in the right place. Go, with its built-in concurrency support, provides a powerful toolkit for this task. But what makes Go's approach unique, and what are the pitfalls to watch out for?
Go's concurrency model, centered around goroutines and channels, offers a clean and efficient way to handle concurrent operations. When building concurrent data structures, we leverage these features to ensure thread safety and performance. But it's not just about using the right tools; it's about understanding the underlying principles and applying them effectively.
Let's start with a simple yet powerful example: a concurrent queue. In Go, we can implement a thread-safe queue using a slice and a mutex. Here's how it might look:
package main import ( "fmt" "sync" ) type Queue struct { items []int lock sync.Mutex } func (q *Queue) Enqueue(item int) { q.lock.Lock() defer q.lock.Unlock() q.items = append(q.items, item) } func (q *Queue) Dequeue() (int, bool) { q.lock.Lock() defer q.lock.Unlock() if len(q.items) == 0 { return 0, false } item := q.items[0] q.items = q.items[1:] return item, true } func main() { q := &Queue{} q.Enqueue(1) q.Enqueue(2) item, ok := q.Dequeue() if ok { fmt.Println("Dequeued:", item) } }
This example demonstrates the use of a mutex to protect shared state. The Enqueue
and Dequeue
methods lock the mutex before modifying the queue, ensuring that only one goroutine can access the queue at a time. This approach is straightforward but can lead to performance bottlenecks in high-concurrency scenarios.
Now, let's explore a more advanced technique: using channels to implement a concurrent queue. Channels in Go are designed for safe communication between goroutines, making them an excellent choice for concurrent data structures.
package main import ( "fmt" ) type Queue chan int func (q Queue) Enqueue(item int) { q <- item } func (q Queue) Dequeue() (int, bool) { select { case item := <-q: return item, true default: return 0, false } } func main() { q := make(Queue, 10) q.Enqueue(1) q.Enqueue(2) item, ok := q.Dequeue() if ok { fmt.Println("Dequeued:", item) } }
This implementation uses a channel as the underlying data structure. The Enqueue
method sends an item to the channel, and the Dequeue
method attempts to receive an item from the channel. This approach is more efficient in high-concurrency scenarios because it avoids the overhead of mutex locking.
However, using channels comes with its own set of challenges. For instance, if the channel is full, the Enqueue
operation will block, potentially causing performance issues. Similarly, if the channel is empty, the Dequeue
operation will block unless we use a non-blocking select
statement, as shown in the example.
When building concurrent data structures, it's crucial to consider the trade-offs between different approaches. Mutexes provide a simple way to ensure thread safety but can lead to contention and performance bottlenecks. Channels offer a more scalable solution but require careful management to avoid blocking.
In my experience, the choice between mutexes and channels often depends on the specific requirements of your application. For low-concurrency scenarios, a mutex-based approach might be sufficient. But as concurrency increases, channels can provide better performance and scalability.
Another important aspect to consider is the use of atomic operations. Go's sync/atomic
package provides functions for performing atomic operations on shared variables. These can be useful for implementing concurrent data structures with minimal overhead.
package main import ( "fmt" "sync/atomic" ) type Counter struct { value int64 } func (c *Counter) Increment() { atomic.AddInt64(&c.value, 1) } func (c *Counter) Value() int64 { return atomic.LoadInt64(&c.value) } func main() { c := &Counter{} c.Increment() fmt.Println("Counter value:", c.Value()) }
This example demonstrates a simple counter using atomic operations. The Increment
method atomically increments the counter, and the Value
method atomically reads the current value. This approach is highly efficient and suitable for scenarios where you need to update shared state frequently.
Building concurrent data structures in Go is both an art and a science. It requires a deep understanding of Go's concurrency model and a careful consideration of the trade-offs involved. Whether you choose mutexes, channels, or atomic operations, the key is to design your data structures with performance, scalability, and safety in mind.
In conclusion, Go provides a rich set of tools for building concurrent data structures. By leveraging goroutines, channels, mutexes, and atomic operations, you can create efficient and safe data structures that meet the demands of modern, concurrent applications. Remember, the best approach depends on your specific use case, so experiment, measure, and iterate to find the optimal solution for your needs.
The above is the detailed content of Building Concurrent Data Structures in Go. For more information, please follow other related articles on the PHP Chinese website!

Hot AI Tools

Undresser.AI Undress
AI-powered app for creating realistic nude photos

AI Clothes Remover
Online AI tool for removing clothes from photos.

Undress AI Tool
Undress images for free

Clothoff.io
AI clothes remover

Video Face Swap
Swap faces in any video effortlessly with our completely free AI face swap tool!

Hot Article

Hot Tools

Notepad++7.3.1
Easy-to-use and free code editor

SublimeText3 Chinese version
Chinese version, very easy to use

Zend Studio 13.0.1
Powerful PHP integrated development environment

Dreamweaver CS6
Visual web development tools

SublimeText3 Mac version
God-level code editing software (SublimeText3)

Hot Topics










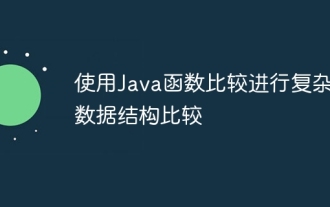
When using complex data structures in Java, Comparator is used to provide a flexible comparison mechanism. Specific steps include: defining the comparator class, rewriting the compare method to define the comparison logic. Create a comparator instance. Use the Collections.sort method, passing in the collection and comparator instances.
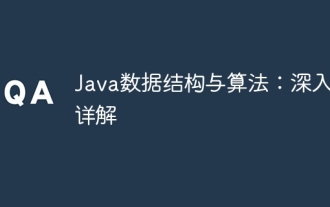
Data structures and algorithms are the basis of Java development. This article deeply explores the key data structures (such as arrays, linked lists, trees, etc.) and algorithms (such as sorting, search, graph algorithms, etc.) in Java. These structures are illustrated through practical examples, including using arrays to store scores, linked lists to manage shopping lists, stacks to implement recursion, queues to synchronize threads, and trees and hash tables for fast search and authentication. Understanding these concepts allows you to write efficient and maintainable Java code.
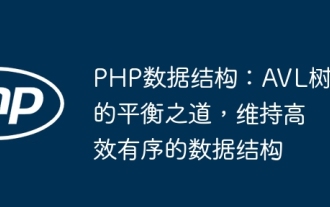
AVL tree is a balanced binary search tree that ensures fast and efficient data operations. To achieve balance, it performs left- and right-turn operations, adjusting subtrees that violate balance. AVL trees utilize height balancing to ensure that the height of the tree is always small relative to the number of nodes, thereby achieving logarithmic time complexity (O(logn)) search operations and maintaining the efficiency of the data structure even on large data sets.
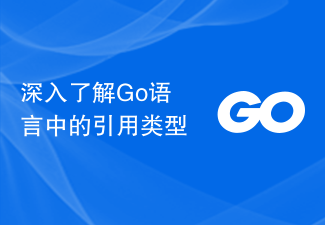
Reference types are a special data type in the Go language. Their values do not directly store the data itself, but the address of the stored data. In the Go language, reference types include slices, maps, channels, and pointers. A deep understanding of reference types is crucial to understanding the memory management and data transfer methods of the Go language. This article will combine specific code examples to introduce the characteristics and usage of reference types in Go language. 1. Slices Slices are one of the most commonly used reference types in the Go language.
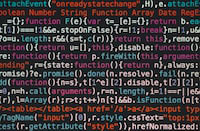
Overview of Java Collection Framework The Java collection framework is an important part of the Java programming language. It provides a series of container class libraries that can store and manage data. These container class libraries have different data structures to meet the data storage and processing needs in different scenarios. The advantage of the collection framework is that it provides a unified interface, allowing developers to operate different container class libraries in the same way, thereby reducing the difficulty of development. Data structures of the Java collection framework The Java collection framework contains a variety of data structures, each of which has its own unique characteristics and applicable scenarios. The following are several common Java collection framework data structures: 1. List: List is an ordered collection that allows elements to be repeated. Li
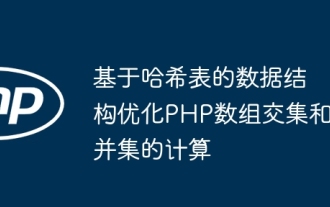
The hash table can be used to optimize PHP array intersection and union calculations, reducing the time complexity from O(n*m) to O(n+m). The specific steps are as follows: Use a hash table to map the elements of the first array to a Boolean value to quickly find whether the element in the second array exists and improve the efficiency of intersection calculation. Use a hash table to mark the elements of the first array as existing, and then add the elements of the second array one by one, ignoring existing elements to improve the efficiency of union calculations.
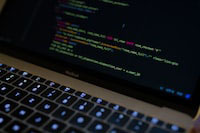
Overview of the PHPSPL Data Structure Library The PHPSPL (Standard PHP Library) data structure library contains a set of classes and interfaces for storing and manipulating various data structures. These data structures include arrays, linked lists, stacks, queues, and sets, each of which provides a specific set of methods and properties for manipulating data. Arrays In PHP, an array is an ordered collection that stores a sequence of elements. The SPL array class provides enhanced functions for native PHP arrays, including sorting, filtering, and mapping. Here is an example of using the SPL array class: useSplArrayObject;$array=newArrayObject(["foo","bar","baz"]);$array
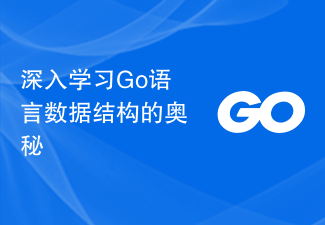
In-depth study of the mysteries of Go language data structure requires specific code examples. As a concise and efficient programming language, Go language also shows its unique charm in processing data structures. Data structure is a basic concept in computer science, which aims to organize and manage data so that it can be accessed and manipulated more efficiently. By in-depth learning the mysteries of Go language data structure, we can better understand how data is stored and operated, thereby improving programming efficiency and code quality. 1. Array Array is one of the simplest data structures
