


What is NumPy, and why is it important for numerical computing in Python?
NumPy is essential for numerical computing in Python due to its speed, memory efficiency, and comprehensive mathematical functions. 1) It's fast because it performs operations in C. 2) NumPy arrays are more memory-efficient than Python lists. 3) It offers a wide range of mathematical operations, from basic to complex, saving time on implementation.
NumPy, short for Numerical Python, is a fundamental package for scientific computing in Python. It's like the Swiss Army knife of numerical operations, providing support for large, multi-dimensional arrays and matrices, along with a vast collection of high-level mathematical functions to operate on these arrays. Let's dive into why NumPy is such a powerhouse in the world of numerical computing.
NumPy is essential for several reasons. First off, it's incredibly fast. When I first started using NumPy, I was blown away by how much quicker my computations were compared to using plain Python lists. This speed comes from NumPy's ability to perform operations in C, which is much faster than Python's interpreted nature. Imagine trying to crunch through a massive dataset with millions of rows and columns; NumPy makes this task not just possible, but efficient.
Another key aspect is its memory efficiency. NumPy arrays are more compact than Python lists, which means you can handle larger datasets without running out of memory. I've worked on projects where memory was a constraint, and using NumPy allowed us to push the limits of what was possible.
But it's not just about speed and memory; NumPy also brings a whole suite of mathematical functions to the table. From basic operations like addition and multiplication to more complex ones like Fourier transforms and linear algebra, NumPy has it all. This means you can do advanced numerical computations without needing to implement everything from scratch, which is a huge time-saver.
Let's take a look at a simple example to see NumPy in action:
import numpy as np # Create a 2D array arr = np.array([[1, 2, 3], [4, 5, 6]]) # Perform element-wise operations print(arr * 2) print(np.sin(arr))
This code demonstrates how easy it is to work with arrays and apply mathematical operations. The output will be:
[[ 2 4 6] [ 8 10 12]] [[ 0.8415 0.9093 0.1411] [ 0.9093 -0.7568 -0.2794]]
Now, let's talk about some of the challenges and considerations when using NumPy. One thing to keep in mind is that while NumPy is incredibly powerful, it's not always the best tool for every job. For instance, if you're dealing with small datasets or simple operations, the overhead of using NumPy might not be worth it. I've seen beginners dive into NumPy for everything, only to realize later that they could have used simpler Python constructs.
Another potential pitfall is the learning curve. NumPy has its own way of doing things, and it can take some time to get used to. I remember struggling with broadcasting rules when I first started, but once you get the hang of it, it's incredibly intuitive.
In terms of optimization, one thing I've learned is to always profile your code. NumPy is fast, but that doesn't mean you can't make it faster. I've found that using vectorized operations instead of loops can lead to significant performance improvements. Here's an example of how you can optimize a simple operation:
import numpy as np import time # Non-vectorized approach start = time.time() arr = np.arange(1000000) result = [x**2 for x in arr] print(f"Non-vectorized time: {time.time() - start:.4f} seconds") # Vectorized approach start = time.time() arr = np.arange(1000000) result = arr**2 print(f"Vectorized time: {time.time() - start:.4f} seconds")
This code will show you the difference in execution time between a non-vectorized and a vectorized approach. The vectorized version will be significantly faster, highlighting the power of NumPy's optimized operations.
In conclusion, NumPy is not just a library; it's a cornerstone of numerical computing in Python. Its speed, efficiency, and comprehensive functionality make it an indispensable tool for anyone working with data or mathematical computations. Whether you're a beginner or an experienced programmer, mastering NumPy will unlock a world of possibilities in your data-driven projects.
The above is the detailed content of What is NumPy, and why is it important for numerical computing in Python?. For more information, please follow other related articles on the PHP Chinese website!

Hot AI Tools

Undresser.AI Undress
AI-powered app for creating realistic nude photos

AI Clothes Remover
Online AI tool for removing clothes from photos.

Undress AI Tool
Undress images for free

Clothoff.io
AI clothes remover

Video Face Swap
Swap faces in any video effortlessly with our completely free AI face swap tool!

Hot Article

Hot Tools

Notepad++7.3.1
Easy-to-use and free code editor

SublimeText3 Chinese version
Chinese version, very easy to use

Zend Studio 13.0.1
Powerful PHP integrated development environment

Dreamweaver CS6
Visual web development tools

SublimeText3 Mac version
God-level code editing software (SublimeText3)

Hot Topics










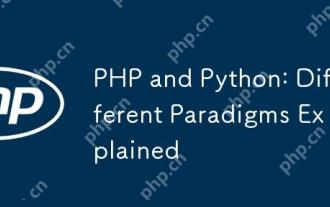
PHP is mainly procedural programming, but also supports object-oriented programming (OOP); Python supports a variety of paradigms, including OOP, functional and procedural programming. PHP is suitable for web development, and Python is suitable for a variety of applications such as data analysis and machine learning.
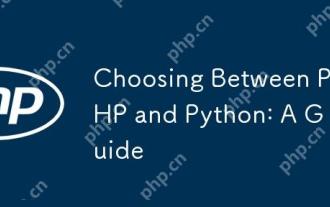
PHP is suitable for web development and rapid prototyping, and Python is suitable for data science and machine learning. 1.PHP is used for dynamic web development, with simple syntax and suitable for rapid development. 2. Python has concise syntax, is suitable for multiple fields, and has a strong library ecosystem.
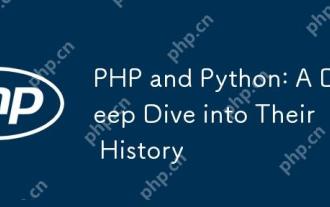
PHP originated in 1994 and was developed by RasmusLerdorf. It was originally used to track website visitors and gradually evolved into a server-side scripting language and was widely used in web development. Python was developed by Guidovan Rossum in the late 1980s and was first released in 1991. It emphasizes code readability and simplicity, and is suitable for scientific computing, data analysis and other fields.
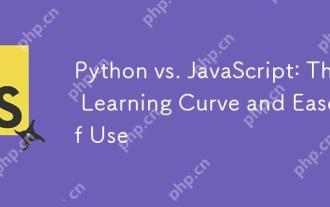
Python is more suitable for beginners, with a smooth learning curve and concise syntax; JavaScript is suitable for front-end development, with a steep learning curve and flexible syntax. 1. Python syntax is intuitive and suitable for data science and back-end development. 2. JavaScript is flexible and widely used in front-end and server-side programming.
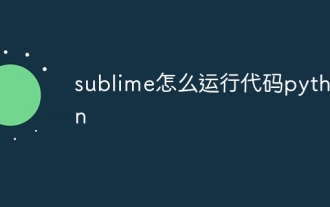
To run Python code in Sublime Text, you need to install the Python plug-in first, then create a .py file and write the code, and finally press Ctrl B to run the code, and the output will be displayed in the console.
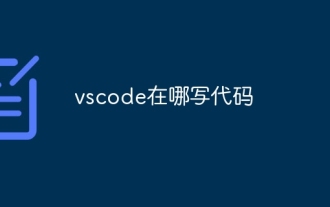
Writing code in Visual Studio Code (VSCode) is simple and easy to use. Just install VSCode, create a project, select a language, create a file, write code, save and run it. The advantages of VSCode include cross-platform, free and open source, powerful features, rich extensions, and lightweight and fast.
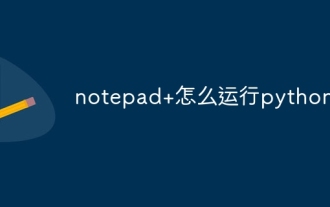
Running Python code in Notepad requires the Python executable and NppExec plug-in to be installed. After installing Python and adding PATH to it, configure the command "python" and the parameter "{CURRENT_DIRECTORY}{FILE_NAME}" in the NppExec plug-in to run Python code in Notepad through the shortcut key "F6".
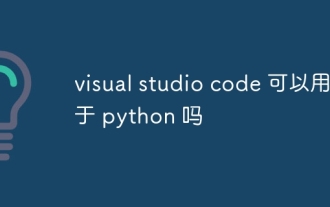
VS Code can be used to write Python and provides many features that make it an ideal tool for developing Python applications. It allows users to: install Python extensions to get functions such as code completion, syntax highlighting, and debugging. Use the debugger to track code step by step, find and fix errors. Integrate Git for version control. Use code formatting tools to maintain code consistency. Use the Linting tool to spot potential problems ahead of time.
