Best Linux Tools for AI and Machine Learning in 2025
Artificial Intelligence (AI) is rapidly transforming numerous sectors, from healthcare and finance to creative fields like art and music. Linux, with its open-source nature, adaptability, and performance capabilities, has emerged as a premier platform for AI development.
This guide explores key Linux tools for AI development, suitable for both novice and seasoned developers.
Why Choose Linux for AI Development?
Linux's dominance in AI is driven by several key advantages:
- Open-Source Foundation: Facilitates modification and customization, vital for the iterative nature of AI development.
- Reliability and High Performance: Effectively handles demanding workloads and intricate model training.
- Robust Community Support: A large and active community offers extensive resources and troubleshooting assistance.
- AI Framework Compatibility: Optimized for major frameworks such as TensorFlow and PyTorch.
- Command-Line Interface (CLI): Provides powerful and efficient control over system resources.
Essential Linux Tools for AI Development
For clarity, we've categorized these tools based on their primary functions.
1. Deep Learning Frameworks: These are fundamental to AI development, enabling the creation, training, and deployment of machine learning models.
-
TensorFlow: Developed by Google, TensorFlow is a robust framework for building and training machine learning models, especially deep learning models. Its versatility makes it suitable for research and production deployments. Keras, a high-level API, simplifies model building, while TensorFlow Extended (TFX) supports production-level deployments. Installation via pip:
pip install tensorflow
-
PyTorch: Developed by Facebook AI Research (FAIR), PyTorch is favored by researchers for its dynamic computation graphs, offering flexibility in model experimentation and debugging. TorchScript enables model optimization for production. Installation via pip:
pip install torch
2. Data Science and Machine Learning Tools: These are crucial for data preprocessing, analysis, and traditional machine learning tasks.
-
Scikit-learn: A comprehensive library offering various machine learning algorithms, including classification, regression, clustering, and dimensionality reduction. Suitable for both beginners and experts. Installation via pip:
pip install scikit-learn
-
XGBoost/LightGBM/CatBoost: High-performance gradient boosting libraries renowned for accuracy and widely used in machine learning competitions and real-world applications. Installation via pip:
pip install xgboost lightgbm catboost
3. Development Environment and Workflow Tools: These tools streamline code writing, testing, and debugging.
-
Jupyter Notebooks/Lab: Provides an interactive environment for coding, data visualization, and documentation, ideal for data exploration and model prototyping. Installation via pip:
pip install jupyterlab
orpip install notebook
-
Integrated Development Environments (IDEs): Popular IDEs like VS Code (with Python extensions) and PyCharm offer features like code completion, debugging, and version control integration, excellent for managing large AI projects. (Download links available on their respective websites.)
4. Containerization and Deployment Tools: These tools facilitate efficient packaging and deployment of AI applications.
-
Docker: Simplifies packaging AI applications and their dependencies into containers, ensuring consistent execution across different environments. Installation (on Debian/Ubuntu):
sudo apt install docker.io
-
Kubernetes: A powerful container orchestration platform for managing and scaling containerized AI applications. Installation command (check official documentation for latest):
curl -LO "https://dl.k8s.io/release/$(curl -L -s https://dl.k8s.io/release/stable.txt)/bin/linux/amd64/kubectl"
-
Kubeflow: Streamlines machine learning workflows on Kubernetes, from data preprocessing to model deployment. (Installation instructions vary depending on version; consult official documentation.)
5. Data Processing and Big Data Tools: Essential for handling large datasets and distributed computing.
- Apache Spark: A powerful distributed computing framework for big data processing and machine learning. Its MLlib library provides scalable algorithms. (Installation instructions are lengthy and require careful attention to detail; refer to the official Apache Spark documentation.)
6. Computer Vision Tools: Crucial for AI projects involving image and video processing.
-
OpenCV: (Open Source Computer Vision Library) offers a wide range of functions for image and video processing. Installation via pip:
pip install opencv-python
7. Other Useful Tools: These tools enhance productivity and streamline the AI development process.
-
Anaconda/Miniconda: Simplifies Python and R package management. Installation (Anaconda): (Download the appropriate installer from the Anaconda website.)
-
Hugging Face Transformers: Provides access to pre-trained transformer models for NLP tasks. Installation via pip:
pip install transformers
-
MLflow: An open-source platform for managing the machine learning lifecycle. Installation via pip:
pip install mlflow
Conclusion:
The AI field is constantly evolving, and Linux offers a robust and adaptable platform for developers. By mastering these essential tools, developers can effectively build, train, and deploy cutting-edge AI models. Always refer to the official documentation for the most current information and installation instructions for each tool.
The above is the detailed content of Best Linux Tools for AI and Machine Learning in 2025. For more information, please follow other related articles on the PHP Chinese website!

Hot AI Tools

Undresser.AI Undress
AI-powered app for creating realistic nude photos

AI Clothes Remover
Online AI tool for removing clothes from photos.

Undress AI Tool
Undress images for free

Clothoff.io
AI clothes remover

Video Face Swap
Swap faces in any video effortlessly with our completely free AI face swap tool!

Hot Article

Hot Tools

Notepad++7.3.1
Easy-to-use and free code editor

SublimeText3 Chinese version
Chinese version, very easy to use

Zend Studio 13.0.1
Powerful PHP integrated development environment

Dreamweaver CS6
Visual web development tools

SublimeText3 Mac version
God-level code editing software (SublimeText3)

Hot Topics
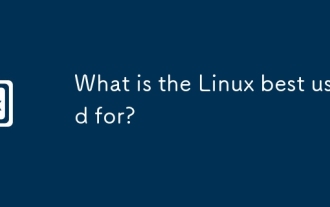
Linux is best used as server management, embedded systems and desktop environments. 1) In server management, Linux is used to host websites, databases, and applications, providing stability and reliability. 2) In embedded systems, Linux is widely used in smart home and automotive electronic systems because of its flexibility and stability. 3) In the desktop environment, Linux provides rich applications and efficient performance.
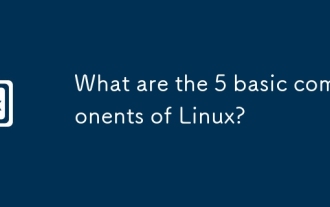
The five basic components of Linux are: 1. The kernel, managing hardware resources; 2. The system library, providing functions and services; 3. Shell, the interface for users to interact with the system; 4. The file system, storing and organizing data; 5. Applications, using system resources to implement functions.
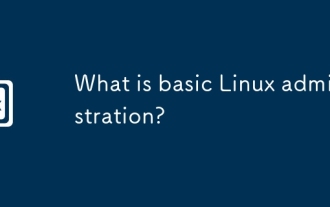
Linux system management ensures the system stability, efficiency and security through configuration, monitoring and maintenance. 1. Master shell commands such as top and systemctl. 2. Use apt or yum to manage the software package. 3. Write automated scripts to improve efficiency. 4. Common debugging errors such as permission problems. 5. Optimize performance through monitoring tools.
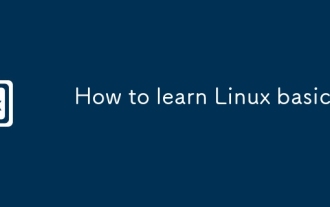
The methods for basic Linux learning from scratch include: 1. Understand the file system and command line interface, 2. Master basic commands such as ls, cd, mkdir, 3. Learn file operations, such as creating and editing files, 4. Explore advanced usage such as pipelines and grep commands, 5. Master debugging skills and performance optimization, 6. Continuously improve skills through practice and exploration.
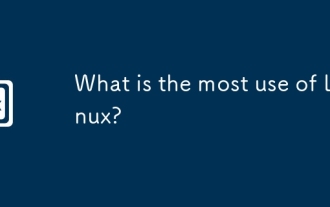
Linux is widely used in servers, embedded systems and desktop environments. 1) In the server field, Linux has become an ideal choice for hosting websites, databases and applications due to its stability and security. 2) In embedded systems, Linux is popular for its high customization and efficiency. 3) In the desktop environment, Linux provides a variety of desktop environments to meet the needs of different users.
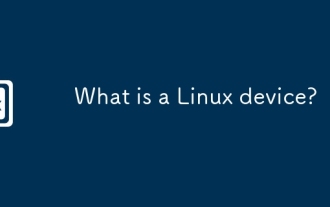
Linux devices are hardware devices running Linux operating systems, including servers, personal computers, smartphones and embedded systems. They take advantage of the power of Linux to perform various tasks such as website hosting and big data analytics.
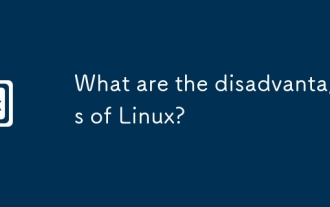
The disadvantages of Linux include user experience, software compatibility, hardware support, and learning curve. 1. The user experience is not as friendly as Windows or macOS, and it relies on the command line interface. 2. The software compatibility is not as good as other systems and lacks native versions of many commercial software. 3. Hardware support is not as comprehensive as Windows, and drivers may be compiled manually. 4. The learning curve is steep, and mastering command line operations requires time and patience.
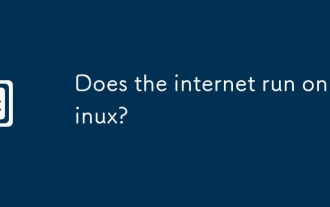
The Internet does not rely on a single operating system, but Linux plays an important role in it. Linux is widely used in servers and network devices and is popular for its stability, security and scalability.
