Bias Score: Evaluating Fairness and Bias in Language Models
Assessing Bias in AI: A Comprehensive Guide to Bias Score
Fair and responsible AI development hinges on effectively measuring bias within models. Bias Score provides a robust framework for data scientists and AI engineers to identify hidden prejudices often overlooked in language models. This guide explores Bias Score's role in ethical AI development, offering insights into its application and interpretation.
Table of Contents
- What is Bias Score?
- Types of Bias
- Using Bias Score
- Key Arguments
- Computing Bias Score
- Example: Gender Bias in Word Embeddings
- Evaluating LLMs for Bias
- Tools and Frameworks
- Practical Implementation
- Advantages of Bias Score
- Limitations of Bias Score
- Practical Applications
- Comparison with Other Metrics
- Conclusion
- Frequently Asked Questions
What is Bias Score?
Bias Score is a quantitative metric evaluating bias in AI systems, particularly language models. It assesses the fairness of model treatment across different demographic groups or concepts. The metric encompasses various techniques to quantify biases related to gender, race, religion, age, and other protected attributes.
Acting as an early warning system, Bias Score identifies problematic trends before they impact real-world applications. It offers an objective measure, allowing teams to track bias over time, replacing subjective evaluations. Incorporating Bias Score into NLP projects demonstrates a commitment to equity and proactive bias reduction.
Types of Bias
Bias Score can measure several bias types:
- Gender Bias: Detects when models associate specific professions, traits, or behaviors predominantly with one gender.
- Racial Bias: Identifies preferences or negative associations with particular racial or ethnic groups.
- Religious Bias: Measures prejudice against or favoritism toward specific religious groups.
- Age Bias: Assesses ageism in models, such as negative portrayals of older adults.
- Socioeconomic Bias: Measures prejudice based on income, education, or social class.
- Ability Bias: Examines how models represent people with disabilities.
Each bias type requires specific measurement approaches within the Bias Score framework. Comprehensive bias evaluation considers multiple dimensions for a complete picture of model fairness.
Using Bias Score
Implementing Bias Score involves:
- Define Bias Categories: Identify the bias types relevant to your application.
- Select Test Sets: Create datasets designed to reveal biases.
- Run Evaluations: Process test sets through the model and collect outputs.
- Calculate Metrics: Apply formulas to quantify bias levels.
- Analyze Results: Identify problematic areas and patterns.
- Implement Mitigations: Develop strategies to address identified biases.
- Monitor Changes: Regularly re-evaluate to track improvements.
Key Arguments
Effective Bias Score calculation requires:
- Model Under Test: The AI system being evaluated.
- Test Dataset: Carefully curated examples.
- Target Attributes: The protected characteristics being measured.
- Baseline Expectations: Reference points representing unbiased responses.
- Measurement Threshold: Acceptable difference levels defining bias.
- Context Parameters: Factors affecting result interpretation.
These arguments are customized based on the specific use case and bias types.
Computing Bias Score
Bias Score computation involves selecting appropriate formulas capturing different bias dimensions. Several formulas form the foundation of Bias Score calculations. The process includes data preparation, response collection, feature extraction, statistical analysis, and score aggregation. Specific formulas, including the Basic Bias Score, Normalized Bias Score, Word Embedding Bias Score, Response Probability Bias Score, and Aggregate Bias Score, are described in detail in the original document. The R-specific Bias Score is also discussed, noting its unique interpretation scale.
Example: Gender Bias in Word Embeddings
An example using word embeddings demonstrates how to measure gender bias. The process involves defining attribute sets (male and female terms and target professions), calculating embedding associations, computing the Bias Score, and interpreting the results. Sample results are provided, showing bias towards specific genders for certain professions.
Evaluating LLMs for Bias
Evaluating Large Language Models (LLMs) requires specific considerations, including prompt engineering, template testing, response analysis, contextual assessment, intersectional evaluation, and benchmark comparison. The use of counterfactual data augmentation is also discussed as a method for bias reduction.
Tools and Frameworks
Several tools facilitate Bias Score implementation:
- Responsible AI Toolbox (Microsoft)
- AI Fairness 360 (IBM)
- FairLearn
- What-If Tool (Google)
- HuggingFace Evaluate
- Captum
- R Statistical Package
The choice of framework depends on technical stack and specific needs.
Practical Implementation
A Python implementation example using transformers
and scikit-learn
is provided, demonstrating the calculation of Bias Score for gender bias in professions. This example shows a practical application of the Bias Score methodology.
Advantages of Bias Score
Bias Score offers several advantages:
- Quantitative Measurement
- Systematic Detection
- Standardized Approach
- Actionable Insights
- Regulatory Compliance
- Client Trust
Limitations of Bias Score
Bias Score also has limitations:
- Context Sensitivity
- Definition Dependence
- Benchmark Scarcity
- Intersectionality Challenges
- Data Limitations
- Moving Target
These limitations necessitate a multifaceted approach to bias assessment.
Practical Applications
Bias Score has various practical applications:
- Model Selection
- Dataset Improvement
- Regulatory Compliance
- Product Development
- Academic Research
- Customer Assurance
Comparison with Other Metrics
A table compares Bias Score with other fairness metrics (WEAT, FairnessTensor, Disparate Impact, Counterfactual Fairness, Equal Opportunity, Demographic Parity, R-BiasScore), highlighting their strengths and weaknesses.
Conclusion
Bias Score provides a crucial framework for measuring and mitigating bias in AI. Its continued evolution will incorporate more sophisticated approaches to intersectionality and context.
Frequently Asked Questions
The FAQ section addresses common questions regarding Bias Score, its differences from other fairness metrics, frequency of evaluation, regulatory compliance, best practices for LLMs, and strategies for model improvement when high Bias Scores are detected.
The above is the detailed content of Bias Score: Evaluating Fairness and Bias in Language Models. For more information, please follow other related articles on the PHP Chinese website!

Hot AI Tools

Undresser.AI Undress
AI-powered app for creating realistic nude photos

AI Clothes Remover
Online AI tool for removing clothes from photos.

Undress AI Tool
Undress images for free

Clothoff.io
AI clothes remover

Video Face Swap
Swap faces in any video effortlessly with our completely free AI face swap tool!

Hot Article

Hot Tools

Notepad++7.3.1
Easy-to-use and free code editor

SublimeText3 Chinese version
Chinese version, very easy to use

Zend Studio 13.0.1
Powerful PHP integrated development environment

Dreamweaver CS6
Visual web development tools

SublimeText3 Mac version
God-level code editing software (SublimeText3)

Hot Topics
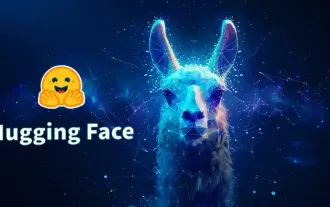
Meta's Llama 3.2: A Leap Forward in Multimodal and Mobile AI Meta recently unveiled Llama 3.2, a significant advancement in AI featuring powerful vision capabilities and lightweight text models optimized for mobile devices. Building on the success o
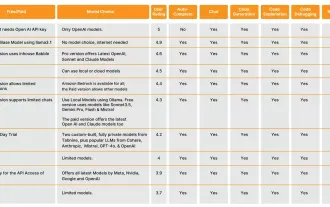
Hey there, Coding ninja! What coding-related tasks do you have planned for the day? Before you dive further into this blog, I want you to think about all your coding-related woes—better list those down. Done? – Let’
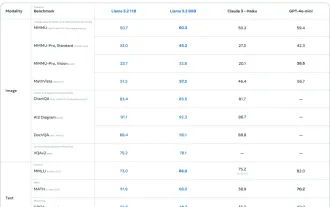
This week's AI landscape: A whirlwind of advancements, ethical considerations, and regulatory debates. Major players like OpenAI, Google, Meta, and Microsoft have unleashed a torrent of updates, from groundbreaking new models to crucial shifts in le
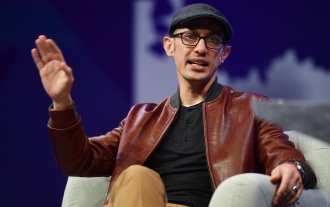
Shopify CEO Tobi Lütke's recent memo boldly declares AI proficiency a fundamental expectation for every employee, marking a significant cultural shift within the company. This isn't a fleeting trend; it's a new operational paradigm integrated into p
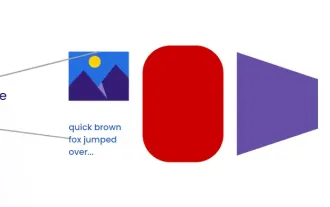
Introduction Imagine walking through an art gallery, surrounded by vivid paintings and sculptures. Now, what if you could ask each piece a question and get a meaningful answer? You might ask, “What story are you telling?
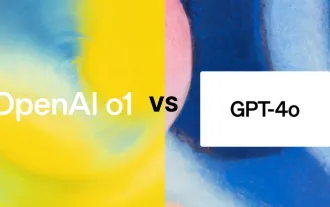
Introduction OpenAI has released its new model based on the much-anticipated “strawberry” architecture. This innovative model, known as o1, enhances reasoning capabilities, allowing it to think through problems mor
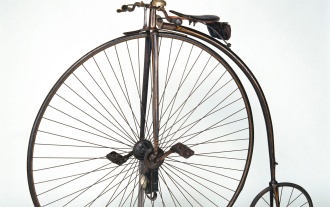
The 2025 Artificial Intelligence Index Report released by the Stanford University Institute for Human-Oriented Artificial Intelligence provides a good overview of the ongoing artificial intelligence revolution. Let’s interpret it in four simple concepts: cognition (understand what is happening), appreciation (seeing benefits), acceptance (face challenges), and responsibility (find our responsibilities). Cognition: Artificial intelligence is everywhere and is developing rapidly We need to be keenly aware of how quickly artificial intelligence is developing and spreading. Artificial intelligence systems are constantly improving, achieving excellent results in math and complex thinking tests, and just a year ago they failed miserably in these tests. Imagine AI solving complex coding problems or graduate-level scientific problems – since 2023
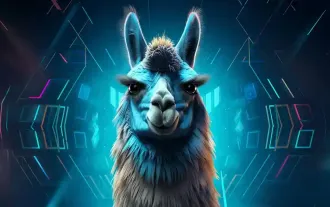
Meta's Llama 3.2: A Multimodal AI Powerhouse Meta's latest multimodal model, Llama 3.2, represents a significant advancement in AI, boasting enhanced language comprehension, improved accuracy, and superior text generation capabilities. Its ability t
