Perplexity Metric for LLM Evaluation - Analytics Vidhya
Evaluating language models remains a significant challenge. How can we accurately assess a model's comprehension, text coherence, and response accuracy? Among numerous evaluation metrics, perplexity stands out as a fundamental and widely used tool in Natural Language Processing (NLP) and Language Model (LM) assessment.
Perplexity's application spans from early statistical language modeling to the current era of large language models (LLMs). This article delves into perplexity: its definition, mechanics, mathematical basis, implementation, strengths, weaknesses, comparisons with other metrics, and how to mitigate its limitations. Upon completion, you'll grasp perplexity and be equipped to implement it for language model evaluation.
Table of Contents:
- What is Perplexity?
- How Perplexity Works
- Calculating Perplexity
- Alternative Perplexity Representations:
- Entropy-Based Perplexity
- Perplexity as a Multiplicative Inverse
- Python Implementation from Scratch
- Example and Results
- Perplexity in NLTK
- Advantages of Perplexity
- Limitations of Perplexity
- Addressing Limitations with LLM-as-Judge
- Practical Applications
- Comparison to Other LLM Evaluation Metrics
- Conclusion
What is Perplexity?
Perplexity quantifies how effectively a probability model predicts a sample. In language models, it reflects the model's "surprise" or "confusion" when encountering a text sequence. Lower perplexity indicates superior predictive ability.
Intuitively:
- Low perplexity: The model confidently and accurately predicts subsequent words.
- High perplexity: The model is uncertain and struggles with predictions.
Perplexity answers: "On average, how many words could plausibly follow each word, according to the model?" A perfect model achieves a perplexity of 1 (minimum). Real models, however, distribute probability across various words, resulting in higher perplexity.
Quick Check: If a model assigns equal probability to 10 potential next words, its perplexity is 10.
How Perplexity Works
Perplexity assesses a language model's performance on a test set:
- Training: A language model is trained on a text corpus.
- Evaluation: The model is evaluated on unseen test data.
- Probability Calculation: The model assigns a probability to each word in the test sequence, considering preceding words. These probabilities are combined into a single perplexity score.
Calculating Perplexity
Mathematically, perplexity is the exponential of the average negative log-likelihood:
Where:
-
W
is the test sequence (w_1, w_2, …, w_N
) -
N
is the number of words -
P(w_i|w_1, w_2, …, w_{i-1})
is the conditional probability ofw_i
given previous words.
Using the chain rule:
Where P(w_1, w_2, …, w_N)
is the joint probability of the sequence.
(Implementation and remaining sections will follow a similar structure of simplification and rewording, maintaining the core information and image placement.)
The above is the detailed content of Perplexity Metric for LLM Evaluation - Analytics Vidhya. For more information, please follow other related articles on the PHP Chinese website!

Hot AI Tools

Undresser.AI Undress
AI-powered app for creating realistic nude photos

AI Clothes Remover
Online AI tool for removing clothes from photos.

Undress AI Tool
Undress images for free

Clothoff.io
AI clothes remover

Video Face Swap
Swap faces in any video effortlessly with our completely free AI face swap tool!

Hot Article

Hot Tools

Notepad++7.3.1
Easy-to-use and free code editor

SublimeText3 Chinese version
Chinese version, very easy to use

Zend Studio 13.0.1
Powerful PHP integrated development environment

Dreamweaver CS6
Visual web development tools

SublimeText3 Mac version
God-level code editing software (SublimeText3)

Hot Topics










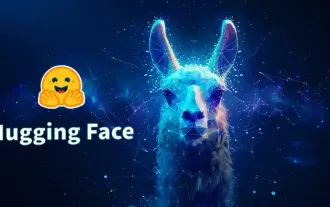
Meta's Llama 3.2: A Leap Forward in Multimodal and Mobile AI Meta recently unveiled Llama 3.2, a significant advancement in AI featuring powerful vision capabilities and lightweight text models optimized for mobile devices. Building on the success o
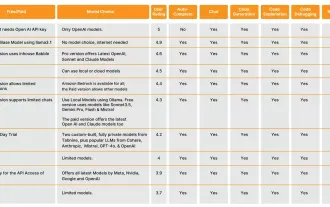
Hey there, Coding ninja! What coding-related tasks do you have planned for the day? Before you dive further into this blog, I want you to think about all your coding-related woes—better list those down. Done? – Let’
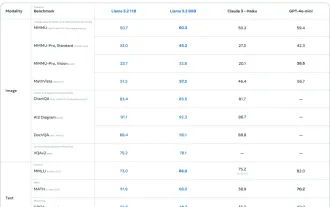
This week's AI landscape: A whirlwind of advancements, ethical considerations, and regulatory debates. Major players like OpenAI, Google, Meta, and Microsoft have unleashed a torrent of updates, from groundbreaking new models to crucial shifts in le
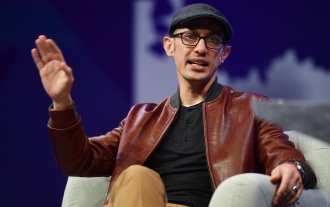
Shopify CEO Tobi Lütke's recent memo boldly declares AI proficiency a fundamental expectation for every employee, marking a significant cultural shift within the company. This isn't a fleeting trend; it's a new operational paradigm integrated into p
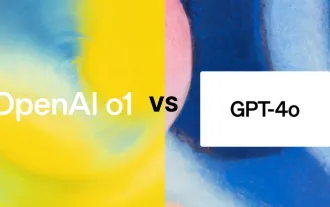
Introduction OpenAI has released its new model based on the much-anticipated “strawberry” architecture. This innovative model, known as o1, enhances reasoning capabilities, allowing it to think through problems mor
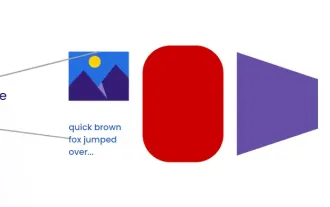
Introduction Imagine walking through an art gallery, surrounded by vivid paintings and sculptures. Now, what if you could ask each piece a question and get a meaningful answer? You might ask, “What story are you telling?
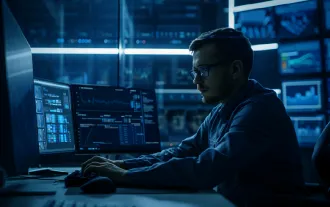
SQL's ALTER TABLE Statement: Dynamically Adding Columns to Your Database In data management, SQL's adaptability is crucial. Need to adjust your database structure on the fly? The ALTER TABLE statement is your solution. This guide details adding colu
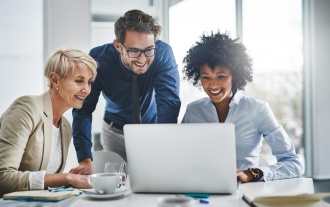
For those of you who might be new to my column, I broadly explore the latest advances in AI across the board, including topics such as embodied AI, AI reasoning, high-tech breakthroughs in AI, prompt engineering, training of AI, fielding of AI, AI re
