Guide to Semantic Kernel
Harnessing the Power of Microsoft's Semantic Kernel: Building Intelligent AI Agents
Recent advancements in AI have moved beyond simple question-answering, embracing reasoning, planning, and action-taking. This evolution is fueled by agentic frameworks like Autogen, LangGraph, and CrewAI, empowering Large Language Models (LLMs) to function as autonomous agents. Microsoft's Semantic Kernel stands out as a particularly robust and developer-friendly option within this landscape. This tutorial explores its unique features, contrasts it with other approaches, and guides you through building your own AI agents.
Learning Objectives
- Grasp the core architecture and functionality of Semantic Kernel.
- Integrate plugins and AI services seamlessly into the Kernel.
- Implement both single-agent and multi-agent systems using Semantic Kernel.
- Understand function calling and orchestration within the framework.
- Develop practical skills in building intelligent agents with Semantic Kernel and Azure OpenAI.
This article is part of the Data Science Blogathon.
Table of Contents
- Understanding Semantic Kernel
- The "Semantic" and "Kernel" Explained
- Agentic Frameworks vs. Traditional API Calls
- Exploring Semantic Kernel Plugins
- A Plugin Example in Semantic Kernel
- Single-Agent System Architecture
- Multi-Agent System Architecture
- Conclusion
- Frequently Asked Questions
Understanding Semantic Kernel
Semantic Kernel combines the power of natural language processing ("semantic") with a core engine ("kernel") that orchestrates tasks, functions, and interactions between AI models and external resources.
The "Semantic" and "Kernel" Explained
Semantic Kernel bridges the gap between LLMs (like GPT) and traditional programming. Developers define functions, plugins, and agents that cooperate in a structured manner. It allows:
- Conjunction of natural language prompts and AI functions with traditional code functions.
- AI-driven reasoning, planning, and task execution using these combined functions.
- Multi-agent collaboration for specialized roles.
Agentic Frameworks vs. Traditional API Calls
A key question arises: Can't we achieve similar results using the OpenAI API directly? While possible, agentic frameworks offer advantages.
Consider a Q&A system for company policies (HR and IT). A traditional API might yield inconsistent results. An agentic framework allows specialized agents (one for HR, one for IT), resulting in more reliable responses.
Exploring Semantic Kernel Plugins
Plugins, similar to those in ChatGPT or Copilot, package existing APIs into reusable tools for AI. This extends AI capabilities beyond its inherent limitations.
Semantic Kernel leverages function calling (a feature of modern LLMs) for planning and API execution. The LLM requests functions, Semantic Kernel redirects to your code, and the results are fed back to the LLM for final response generation.
Code Implementation
Install necessary packages:
pip install semantic-kernel openai pydantic
Here's a Python example demonstrating a weather plugin:
import semantic_kernel as sk from semantic_kernel.connectors.ai.open_ai import AzureChatCompletion def weather_plugin(location: str) -> str: weather_data = { "New York": "Sunny, 25°C", "London": "Cloudy, 18°C", "Tokyo": "Rainy, 22°C" } return weather_data.get(location, "Weather data not available.") kernel = sk.Kernel() kernel.add_service( "azure-openai-chat", AzureChatCompletion( api_key="your-azure-api-key", endpoint="your-azure-endpoint", deployment_name="your-deployment-name" ) ) kernel.add_plugin("WeatherPlugin", weather_plugin) location = "New York" response = kernel.invoke("WeatherPlugin", location) print(f"Weather in {location}: {response}")
A Plugin Example in Semantic Kernel
This example showcases:
- Plugin Definition: The
weather_plugin
simulates weather data retrieval. - Semantic Kernel Integration: The function is added as a plugin using
kernel.add_plugin()
. - AI Utilization: The AI dynamically calls this function.
This illustrates how plugins expand AI functionality beyond basic text generation.
Single-Agent System Architecture
A single agent handles user queries independently, processing requests, gathering information, and generating responses without needing multiple agents or an orchestrator. (Example code omitted for brevity, but available in the original article.)
Multi-Agent System Architecture
Multi-agent systems often employ an orchestrator agent to determine which agent handles a specific request.
For instance, one agent might manage banking data, another healthcare data. The orchestrator decides which agent to invoke based on the query. (Example code omitted for brevity, but available in the original article.) Alternatively, agents can collaborate without an explicit orchestrator.
Conclusion
Semantic Kernel empowers AI through its agentic framework, enabling planning, reasoning, and decision-making. This tutorial highlights the benefits of plugins, contrasts agentic and traditional API approaches, and explains single-agent and multi-agent system architectures. As AI evolves, Semantic Kernel's approach will be crucial for building more efficient and context-aware applications.
(Key takeaways and FAQs omitted for brevity, but available in the original article.) The code examples are available on GitHub (link in original article).
The above is the detailed content of Guide to Semantic Kernel. For more information, please follow other related articles on the PHP Chinese website!

Hot AI Tools

Undresser.AI Undress
AI-powered app for creating realistic nude photos

AI Clothes Remover
Online AI tool for removing clothes from photos.

Undress AI Tool
Undress images for free

Clothoff.io
AI clothes remover

Video Face Swap
Swap faces in any video effortlessly with our completely free AI face swap tool!

Hot Article

Hot Tools

Notepad++7.3.1
Easy-to-use and free code editor

SublimeText3 Chinese version
Chinese version, very easy to use

Zend Studio 13.0.1
Powerful PHP integrated development environment

Dreamweaver CS6
Visual web development tools

SublimeText3 Mac version
God-level code editing software (SublimeText3)

Hot Topics
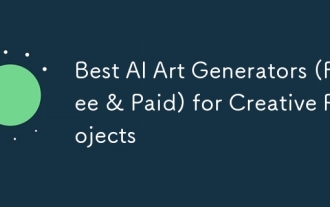
The article reviews top AI art generators, discussing their features, suitability for creative projects, and value. It highlights Midjourney as the best value for professionals and recommends DALL-E 2 for high-quality, customizable art.
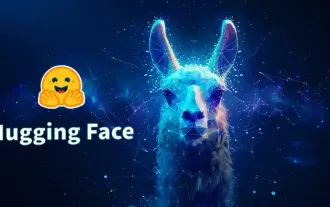
Meta's Llama 3.2: A Leap Forward in Multimodal and Mobile AI Meta recently unveiled Llama 3.2, a significant advancement in AI featuring powerful vision capabilities and lightweight text models optimized for mobile devices. Building on the success o
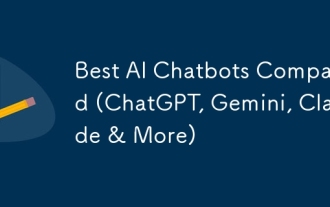
The article compares top AI chatbots like ChatGPT, Gemini, and Claude, focusing on their unique features, customization options, and performance in natural language processing and reliability.
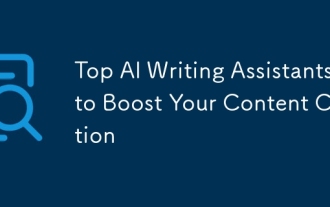
The article discusses top AI writing assistants like Grammarly, Jasper, Copy.ai, Writesonic, and Rytr, focusing on their unique features for content creation. It argues that Jasper excels in SEO optimization, while AI tools help maintain tone consist
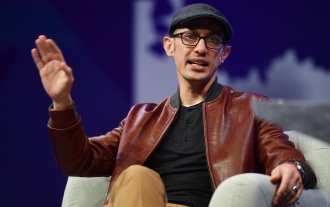
Shopify CEO Tobi Lütke's recent memo boldly declares AI proficiency a fundamental expectation for every employee, marking a significant cultural shift within the company. This isn't a fleeting trend; it's a new operational paradigm integrated into p
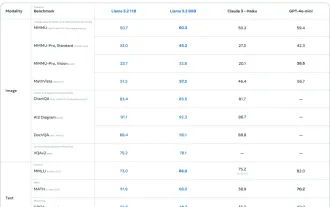
This week's AI landscape: A whirlwind of advancements, ethical considerations, and regulatory debates. Major players like OpenAI, Google, Meta, and Microsoft have unleashed a torrent of updates, from groundbreaking new models to crucial shifts in le
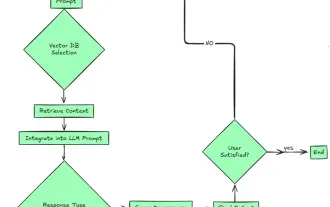
2024 witnessed a shift from simply using LLMs for content generation to understanding their inner workings. This exploration led to the discovery of AI Agents – autonomous systems handling tasks and decisions with minimal human intervention. Buildin
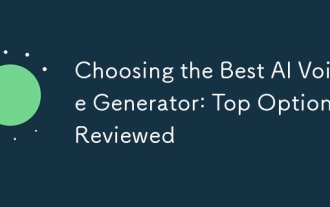
The article reviews top AI voice generators like Google Cloud, Amazon Polly, Microsoft Azure, IBM Watson, and Descript, focusing on their features, voice quality, and suitability for different needs.
