Synthetic Panels: An AI-powered Solution for Market Research
Market research faces unprecedented challenges: dwindling response rates, escalating costs, and tightening privacy regulations. This article explores synthetic panels—AI-generated respondent groups mirroring real-world demographics and behaviors—as a potential solution, examining their capabilities and limitations.
Table of Contents
- What are Synthetic Panels?
- How Synthetic Panels Function
- Merkle Case Study: AI-Generated Survey Panels
- Phase 1: Limitations of Generic AI Responses
- Phase 2: Contextualizing AI for Improved Accuracy
- The Significance of Synthetic Panels
- A Balanced Approach: Combining Human and Synthetic Panels
- Key Considerations for Using Synthetic Panels
- Conclusion
- Frequently Asked Questions
What are Synthetic Panels?
Synthetic panels are AI-created virtual respondent groups designed to mimic the characteristics and actions of real consumer segments. Unlike traditional panels, which demand extensive recruitment and maintenance, synthetic panels leverage machine learning and large datasets to produce digital personas capable of simulating human survey responses. This approach utilizes deep contextual learning from real-world data (past surveys, customer reviews, behavioral data, etc.) to create scalable, privacy-compliant solutions accurately reflecting market segments. Researchers can simulate consumer reactions to new products or hypothetical scenarios, enabling predictive analysis beyond the scope of static historical data.
How Synthetic Panels Function
Synthetic panel creation involves these key steps:
- Data Acquisition and Preparation: Diverse datasets (survey results, customer logs, online reviews, demographic information) are gathered and processed to establish a baseline understanding of consumer behavior.
- Persona Development: AI models generate synthetic personas, each representing a specific consumer archetype (e.g., budget-conscious millennials, tech-savvy Gen Z). These personas encompass psychological traits, brand preferences, and behavioral patterns.
- Response Simulation: When presented with survey questions, personas respond based on their trained profiles. The AI predicts answers using patterns learned from historical data and contextual cues.
- Contextual Adjustment: Continuous refinement using real-world feedback ensures accuracy. Synthetic responses are compared to actual respondent answers, and models are adjusted to mitigate biases.
- Output Analysis: Researchers analyze trends, segment behaviors, and test hypotheses, similar to traditional methods, but with enhanced speed and scalability.
Merkle Case Study: AI-Generated Survey Panels
A Southeast Asian airline, partnering with Merkle, tested AI-generated survey responses against real customer feedback.
Phase 1: Limitations of Generic AI Responses
Initial AI responses, without contextual data, exhibited predictable patterns: neutral NPS scores and brand perception data misaligned with actual customer sentiment. This highlighted the need for real-world context to capture the nuances of human opinion.
Phase 2: Contextualizing AI for Improved Accuracy
Merkle incorporated historical survey data, customer complaints, and feedback trends. This contextualization significantly improved accuracy: brand perception scores aligned with real opinions, customer segmentation mirrored real-world preferences, and NPS distributions became more realistic. This demonstrated the potential of synthetic panels in market research.
The Significance of Synthetic Panels
This case study highlights the transformative potential of synthetic panels:
- Larger Sample Sizes: Supplementing real data with AI-generated responses provides richer insights.
- Predictive Capabilities: AI estimates responses to new questions without conducting new surveys.
- Cost Reduction: Significantly reduces the time and expense of recruiting human respondents.
- Access to Niche Audiences: Effectively models hard-to-reach groups.
- Privacy Compliance: Inherently compliant with data privacy regulations.
- Always-On Availability: Personas are readily available for ongoing research.
A Balanced Approach: Combining Human and Synthetic Panels
While promising, a fully AI-driven approach is still developing. A hybrid model, combining human and synthetic respondents, is currently optimal, allowing for comparison and optimization of synthetic responses against real feedback.
Key Considerations for Using Synthetic Panels
- Avoid "No Training Required" Claims: Careful tuning with real-world data is crucial.
- Validate with Human Data: Always benchmark synthetic responses against real data.
- Avoid Over-Reliance on AI: Synthetic panels should supplement, not replace, human insights.
Conclusion
Merkle's case study demonstrates the value of synthetic panels, particularly when enhanced with contextual information. While complete AI adoption is future-oriented, a hybrid approach offers immediate benefits, improving decision-making and unlocking new market possibilities. Ethical considerations and transparency in using AI-generated insights are paramount. The future of market research lies in intelligent data generation.
This blog is a result of the collective contributions of the following members of the Merkle team: Vinay Mony (Vice President, CXM – Insights & Analytics), Mario Thirituvaraj (Assistant Vice President, CXM – Insights & Analytics), Debasree Bhattacharya (Assistant Vice President, CXM – Insights & Analytics), Rohit Mudukanagoudra (Analyst), Mahima Salian (Analyst), Bharat Shetty (Senior Manager, CXM – Insights & Analytics), and Aneesh Kammath (Head – XM Advisory APAC)
Frequently Asked Questions
Q1. Synthetic vs. Traditional Panels? Traditional panels use real respondents, which is time-consuming and costly. Synthetic panels use AI-generated personas simulating real consumer behavior, providing instant responses based on learned patterns and data.
Q2. Privacy Compliance? Synthetic panels don't rely on identifiable data; they use aggregated, anonymized insights, ensuring compliance with regulations like GDPR and CCPA.
Q3. Complete Replacement of Human Respondents? Not yet. A hybrid approach, combining synthetic and human feedback, is currently the most effective strategy.
Q4. Response Time? Synthetic panels generate responses almost instantly, unlike traditional methods which can take weeks.
Q5. Validating Accuracy? Validation involves comparing synthetic responses with real survey data. Regular calibration and A/B testing ensure alignment with actual consumer sentiment.
Q6. Risks of Over-Reliance? Over-reliance can lead to inaccurate insights if panels aren't regularly updated or validated. Human oversight is crucial for ethical and effective use.
The above is the detailed content of Synthetic Panels: An AI-powered Solution for Market Research. For more information, please follow other related articles on the PHP Chinese website!

Hot AI Tools

Undresser.AI Undress
AI-powered app for creating realistic nude photos

AI Clothes Remover
Online AI tool for removing clothes from photos.

Undress AI Tool
Undress images for free

Clothoff.io
AI clothes remover

Video Face Swap
Swap faces in any video effortlessly with our completely free AI face swap tool!

Hot Article

Hot Tools

Notepad++7.3.1
Easy-to-use and free code editor

SublimeText3 Chinese version
Chinese version, very easy to use

Zend Studio 13.0.1
Powerful PHP integrated development environment

Dreamweaver CS6
Visual web development tools

SublimeText3 Mac version
God-level code editing software (SublimeText3)

Hot Topics










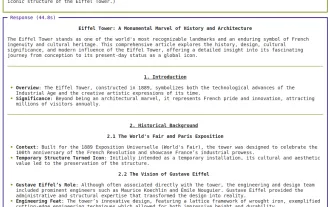
While working on Agentic AI, developers often find themselves navigating the trade-offs between speed, flexibility, and resource efficiency. I have been exploring the Agentic AI framework and came across Agno (earlier it was Phi-
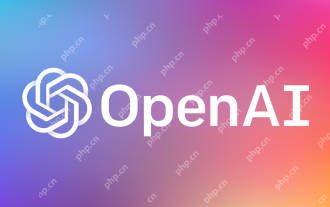
The release includes three distinct models, GPT-4.1, GPT-4.1 mini and GPT-4.1 nano, signaling a move toward task-specific optimizations within the large language model landscape. These models are not immediately replacing user-facing interfaces like
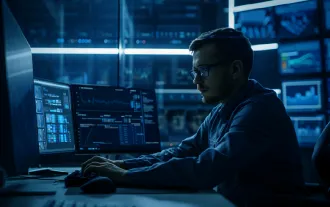
SQL's ALTER TABLE Statement: Dynamically Adding Columns to Your Database In data management, SQL's adaptability is crucial. Need to adjust your database structure on the fly? The ALTER TABLE statement is your solution. This guide details adding colu
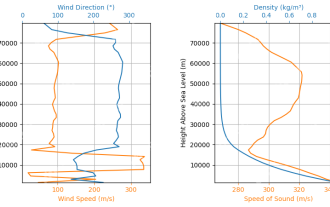
Simulate Rocket Launches with RocketPy: A Comprehensive Guide This article guides you through simulating high-power rocket launches using RocketPy, a powerful Python library. We'll cover everything from defining rocket components to analyzing simula
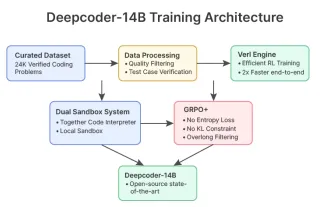
In a significant development for the AI community, Agentica and Together AI have released an open-source AI coding model named DeepCoder-14B. Offering code generation capabilities on par with closed-source competitors like OpenAI
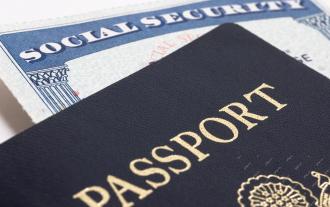
Chip giant Nvidia said on Monday it will start manufacturing AI supercomputers— machines that can process copious amounts of data and run complex algorithms— entirely within the U.S. for the first time. The announcement comes after President Trump si
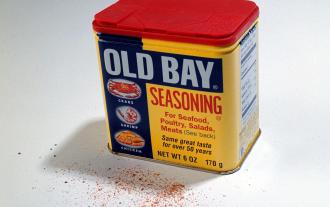
Guy Peri is McCormick’s Chief Information and Digital Officer. Though only seven months into his role, Peri is rapidly advancing a comprehensive transformation of the company’s digital capabilities. His career-long focus on data and analytics informs
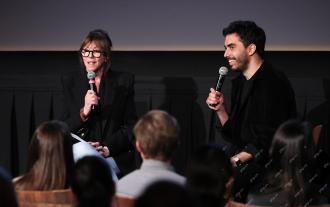
The film industry, alongside all creative sectors, from digital marketing to social media, stands at a technological crossroad. As artificial intelligence begins to reshape every aspect of visual storytelling and change the landscape of entertainment
