Evaluating Toxicity in Large Language Models
This article explores the crucial issue of toxicity in Large Language Models (LLMs) and the methods used to evaluate and mitigate it. LLMs, powering various applications from chatbots to content generation, necessitate robust evaluation metrics, with toxicity assessment being paramount. Toxicity encompasses harmful, offensive, or inappropriate outputs, including hate speech, threats, and misinformation. The article emphasizes the complexities of measuring toxicity due to its inherent subjectivity and cultural variations.
Key Learning Points:
- Understanding Toxicity: The article defines toxicity in LLMs and its real-world consequences.
- Multifaceted Nature of Toxicity: It highlights the diverse dimensions of toxicity, including hate speech, harassment, violent content, and misinformation.
- Evaluation Methods: The article details various approaches, from human evaluation (the gold standard, though resource-intensive) to automated metrics using classifiers like Perspective API and Detoxify, and red-teaming techniques.
- Challenges in Measurement: It addresses the significant hurdles in accurately assessing toxicity, such as context dependency, cultural differences, subjective interpretations, and the ever-evolving nature of toxic language.
- Innovative Approaches: The article discusses advancements like contextual embedding analysis, multi-stage evaluation frameworks, and self-evaluation capabilities in LLMs.
- Practical Implementation: It outlines a practical implementation plan, including pre-deployment evaluation, runtime monitoring, and a continuous improvement cycle involving model retraining and A/B testing.
- Standards and Benchmarks: The article mentions key benchmarks like ToxiGen and RealToxicityPrompts for standardized model evaluation.
- Ethical Considerations: It underscores the ethical implications of toxicity assessment, particularly concerning annotator well-being and bias mitigation.
The article concludes by emphasizing the need for sophisticated and evolving evaluation methods to ensure the safe and responsible deployment of LLMs. A frequently asked questions section provides concise answers to key queries regarding toxicity in LLMs.
The provided code snippets illustrate aspects of automated toxicity detection and monitoring within an LLM application. An example JSON response snippet demonstrates how toxicity scores might be integrated into the output structure. The article comprehensively addresses the technical and ethical challenges in ensuring the safe and beneficial development of LLMs.
The above is the detailed content of Evaluating Toxicity in Large Language Models. For more information, please follow other related articles on the PHP Chinese website!

Hot AI Tools

Undresser.AI Undress
AI-powered app for creating realistic nude photos

AI Clothes Remover
Online AI tool for removing clothes from photos.

Undress AI Tool
Undress images for free

Clothoff.io
AI clothes remover

Video Face Swap
Swap faces in any video effortlessly with our completely free AI face swap tool!

Hot Article

Hot Tools

Notepad++7.3.1
Easy-to-use and free code editor

SublimeText3 Chinese version
Chinese version, very easy to use

Zend Studio 13.0.1
Powerful PHP integrated development environment

Dreamweaver CS6
Visual web development tools

SublimeText3 Mac version
God-level code editing software (SublimeText3)

Hot Topics










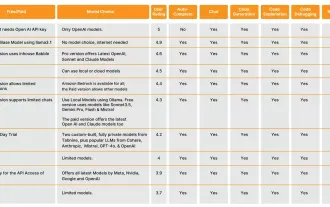
Hey there, Coding ninja! What coding-related tasks do you have planned for the day? Before you dive further into this blog, I want you to think about all your coding-related woes—better list those down. Done? – Let’
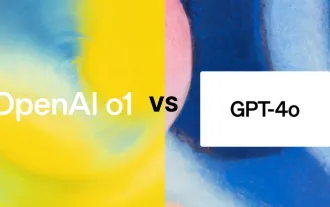
Introduction OpenAI has released its new model based on the much-anticipated “strawberry” architecture. This innovative model, known as o1, enhances reasoning capabilities, allowing it to think through problems mor
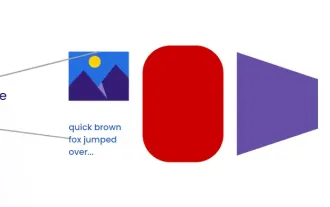
Introduction Imagine walking through an art gallery, surrounded by vivid paintings and sculptures. Now, what if you could ask each piece a question and get a meaningful answer? You might ask, “What story are you telling?
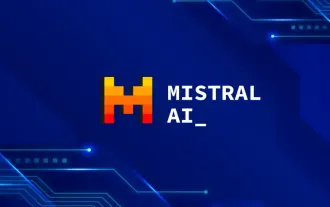
Introduction Mistral has released its very first multimodal model, namely the Pixtral-12B-2409. This model is built upon Mistral’s 12 Billion parameter, Nemo 12B. What sets this model apart? It can now take both images and tex
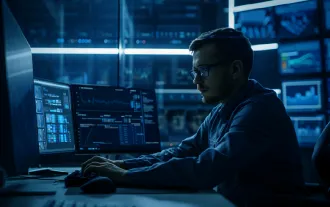
SQL's ALTER TABLE Statement: Dynamically Adding Columns to Your Database In data management, SQL's adaptability is crucial. Need to adjust your database structure on the fly? The ALTER TABLE statement is your solution. This guide details adding colu
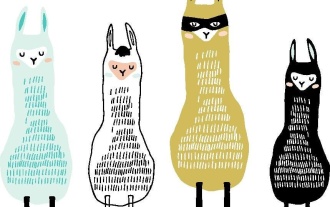
Troubled Benchmarks: A Llama Case Study In early April 2025, Meta unveiled its Llama 4 suite of models, boasting impressive performance metrics that positioned them favorably against competitors like GPT-4o and Claude 3.5 Sonnet. Central to the launc
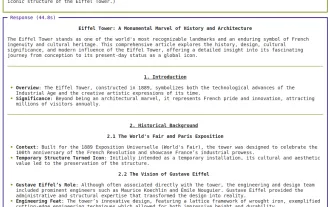
While working on Agentic AI, developers often find themselves navigating the trade-offs between speed, flexibility, and resource efficiency. I have been exploring the Agentic AI framework and came across Agno (earlier it was Phi-
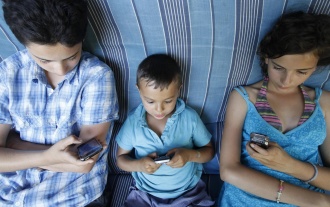
Can a video game ease anxiety, build focus, or support a child with ADHD? As healthcare challenges surge globally — especially among youth — innovators are turning to an unlikely tool: video games. Now one of the world’s largest entertainment indus
