Is Hallucination in Large Language Models (LLMs) Inevitable?
Large Language Models (LLMs) and the Inevitable Problem of Hallucinations
You've likely used AI models like ChatGPT, Claude, and Gemini. These are all examples of Large Language Models (LLMs), powerful AI systems trained on massive text datasets to understand and generate human-like text. However, even the most advanced LLMs suffer from a significant flaw: hallucinations.
Recent research, notably "Hallucination is Inevitable: An Innate Limitation of Large Language Models," argues that these hallucinations – the confident presentation of fabricated information – are an inherent limitation, not a mere bug. This article explores this research and its implications.
Understanding LLMs and Hallucinations
LLMs, while impressive, struggle with "hallucinations," generating plausible-sounding but factually incorrect information. This raises serious concerns about their reliability and ethical implications. The research paper categorizes hallucinations as either intrinsic (contradicting input) or extrinsic (unverifiable by input). Causes are multifaceted, stemming from data quality issues (bias, misinformation, outdated information), training flaws (architectural limitations, exposure bias), and inference problems (sampling randomness).
The Inevitability of Hallucination
The core argument of the research is that hallucination is unavoidable in any computable LLM. The paper uses mathematical proofs (Theorems 1, 2, and 3) to demonstrate this, showing that even with perfect training data and optimal architecture, limitations in computability will inevitably lead to incorrect outputs. This holds true even for LLMs designed for polynomial-time computation. The research highlights that even increasing model size or training data won't eliminate this fundamental limitation.
Empirical Evidence and Mitigation Strategies
The research backs its theoretical claims with empirical evidence. Experiments using Llama 2 and GPT models demonstrated their failure to complete simple string enumeration tasks, further supporting the inevitability of hallucinations.
While complete eradication is impossible, the paper explores mitigation strategies:
- Larger Models & More Data: While helpful, this approach has inherent limits.
- Improved Prompting: Techniques like Chain-of-Thought can improve accuracy but don't solve the core problem.
- Ensemble Methods: Combining multiple LLMs can reduce errors but doesn't eliminate them.
- Safety Constraints ("Guardrails"): These can mitigate harmful outputs but don't address the fundamental issue of factual inaccuracy.
- Knowledge Integration: Incorporating external knowledge sources can improve accuracy in specific domains.
Conclusion: Responsible AI Development
The research concludes that hallucinations are an inherent characteristic of LLMs. While mitigation strategies can reduce their frequency and impact, they cannot eliminate them entirely. This necessitates a shift towards responsible AI development, focusing on:
- Transparency: Acknowledging the limitations of LLMs.
- Safety Measures: Implementing robust safeguards to minimize the risks of hallucinations.
- Human Oversight: Maintaining human control and verification of LLM outputs, especially in critical applications.
- Continued Research: Exploring new approaches to reduce hallucinations and improve the reliability of LLMs.
The future of LLMs requires a pragmatic approach, acknowledging their limitations and focusing on responsible development and deployment. The inevitability of hallucinations underscores the need for ongoing research and a critical evaluation of their applications. This isn't a call to abandon LLMs, but a call for responsible innovation.
(Frequently Asked Questions section would be added here, mirroring the original input's FAQ section.)
The above is the detailed content of Is Hallucination in Large Language Models (LLMs) Inevitable?. For more information, please follow other related articles on the PHP Chinese website!

Hot AI Tools

Undresser.AI Undress
AI-powered app for creating realistic nude photos

AI Clothes Remover
Online AI tool for removing clothes from photos.

Undress AI Tool
Undress images for free

Clothoff.io
AI clothes remover

Video Face Swap
Swap faces in any video effortlessly with our completely free AI face swap tool!

Hot Article

Hot Tools

Notepad++7.3.1
Easy-to-use and free code editor

SublimeText3 Chinese version
Chinese version, very easy to use

Zend Studio 13.0.1
Powerful PHP integrated development environment

Dreamweaver CS6
Visual web development tools

SublimeText3 Mac version
God-level code editing software (SublimeText3)

Hot Topics










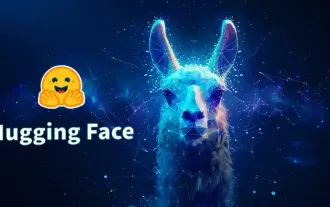
Meta's Llama 3.2: A Leap Forward in Multimodal and Mobile AI Meta recently unveiled Llama 3.2, a significant advancement in AI featuring powerful vision capabilities and lightweight text models optimized for mobile devices. Building on the success o
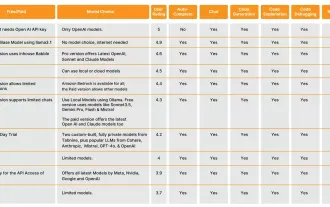
Hey there, Coding ninja! What coding-related tasks do you have planned for the day? Before you dive further into this blog, I want you to think about all your coding-related woes—better list those down. Done? – Let’
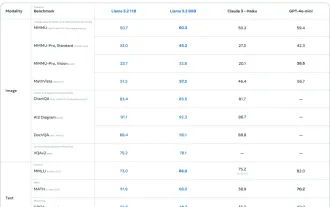
This week's AI landscape: A whirlwind of advancements, ethical considerations, and regulatory debates. Major players like OpenAI, Google, Meta, and Microsoft have unleashed a torrent of updates, from groundbreaking new models to crucial shifts in le
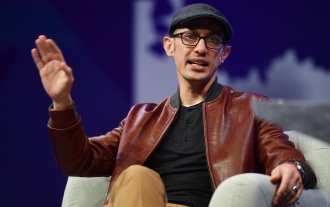
Shopify CEO Tobi Lütke's recent memo boldly declares AI proficiency a fundamental expectation for every employee, marking a significant cultural shift within the company. This isn't a fleeting trend; it's a new operational paradigm integrated into p
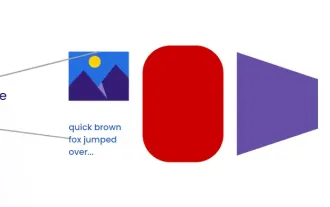
Introduction Imagine walking through an art gallery, surrounded by vivid paintings and sculptures. Now, what if you could ask each piece a question and get a meaningful answer? You might ask, “What story are you telling?
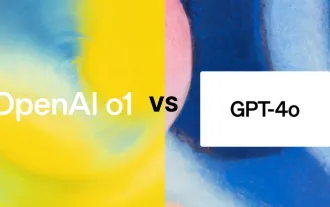
Introduction OpenAI has released its new model based on the much-anticipated “strawberry” architecture. This innovative model, known as o1, enhances reasoning capabilities, allowing it to think through problems mor
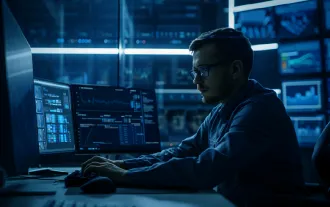
SQL's ALTER TABLE Statement: Dynamically Adding Columns to Your Database In data management, SQL's adaptability is crucial. Need to adjust your database structure on the fly? The ALTER TABLE statement is your solution. This guide details adding colu
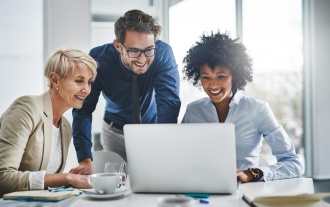
For those of you who might be new to my column, I broadly explore the latest advances in AI across the board, including topics such as embodied AI, AI reasoning, high-tech breakthroughs in AI, prompt engineering, training of AI, fielding of AI, AI re
