How to operate distributed training of PyTorch on CentOS
PyTorch distributed training on CentOS system requires following the following steps:
-
PyTorch installation: The premise is that Python and pip are installed in CentOS system. Depending on your CUDA version, get the appropriate installation command from the PyTorch official website. For CPU-only training, you can use the following command:
pip install torch torchvision torchaudio
Copy after loginIf you need GPU support, make sure that the corresponding version of CUDA and cuDNN are installed and use the corresponding PyTorch version to install.
Distributed environment configuration: Distributed training usually requires multiple machines or single-machine multiple GPUs. All nodes participating in training must be able to network access to each other and correctly configure environment variables such as
MASTER_ADDR
(master node IP address) andMASTER_PORT
(any available port number).-
Distributed training script writing: Use PyTorch's
torch.distributed
package to write distributed training scripts.torch.nn.parallel.DistributedDataParallel
is used to wrap your model, whiletorch.distributed.launch
oraccelerate
libraries are used to start distributed training.Here is an example of a simplified distributed training script:
import torch import torch.nn as nn import torch.optim as optim from torch.nn.parallel import DistributedDataParallel as DDP import torch.distributed as dist def train(rank, world_size): dist.init_process_group(backend='nccl', init_method='env://') # Initialize the process group, use the nccl backend model = ... # Your model definition model.cuda(rank) # Move the model to the specified GPU ddp_model = DDP(model, device_ids=[rank]) # Use DDP to wrap the model criteria = nn.CrossEntropyLoss().cuda(rank) # Loss function optimizer = optim.Adam(ddp_model.parameters(), lr=0.001) # Optimizer dataset = ... # Your dataset sampler = torch.utils.data.distributed.DistributedSampler(dataset, num_replicas=world_size, rank=rank) loader = torch.utils.data.DataLoader(dataset, batch_size=..., sampler=sampler) for epoch in range(...): sampler.set_epoch(epoch) # For each epoch resampling, target in loader: data, target = data.cuda(rank), target.cuda(rank) optimizer.zero_grad() output = ddp_model(data) loss = criteria(output, target) loss.backward() optimizer.step() dist.destroy_process_group() # Destroy process group if __name__ == "__main__": import argparse parser = argparse.ArgumentParser() parser.add_argument('--world-size', type=int, default=2) parser.add_argument('--rank', type=int, default=0) args = parser.parse_args() train(args.rank, args.world_size)
Copy after login -
Distributed training startup: Use the
torch.distributed.launch
tool to start distributed training. For example, run on two GPUs:python -m torch.distributed.launch --nproc_per_node=2 your_training_script.py
Copy after loginIn the case of multiple nodes, ensure that each node runs the corresponding process and that nodes can access each other.
Monitoring and debugging: Distributed training may encounter network communication or synchronization problems. Use
nccl-tests
to test whether the communication between GPUs is normal. Detailed logging is essential for debugging.
Please note that the above steps provide a basic framework that may need to be adjusted according to specific needs and environment in actual applications. It is recommended to refer to the detailed instructions of the official PyTorch documentation on distributed training.
The above is the detailed content of How to operate distributed training of PyTorch on CentOS. For more information, please follow other related articles on the PHP Chinese website!

Hot AI Tools

Undresser.AI Undress
AI-powered app for creating realistic nude photos

AI Clothes Remover
Online AI tool for removing clothes from photos.

Undress AI Tool
Undress images for free

Clothoff.io
AI clothes remover

Video Face Swap
Swap faces in any video effortlessly with our completely free AI face swap tool!

Hot Article

Hot Tools

Notepad++7.3.1
Easy-to-use and free code editor

SublimeText3 Chinese version
Chinese version, very easy to use

Zend Studio 13.0.1
Powerful PHP integrated development environment

Dreamweaver CS6
Visual web development tools

SublimeText3 Mac version
God-level code editing software (SublimeText3)

Hot Topics










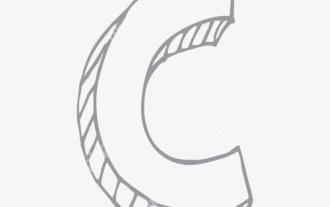
Using the chrono library in C can allow you to control time and time intervals more accurately. Let's explore the charm of this library. C's chrono library is part of the standard library, which provides a modern way to deal with time and time intervals. For programmers who have suffered from time.h and ctime, chrono is undoubtedly a boon. It not only improves the readability and maintainability of the code, but also provides higher accuracy and flexibility. Let's start with the basics. The chrono library mainly includes the following key components: std::chrono::system_clock: represents the system clock, used to obtain the current time. std::chron
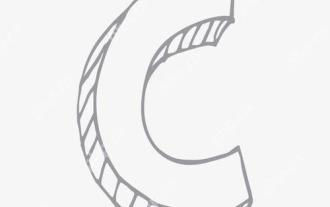
Measuring thread performance in C can use the timing tools, performance analysis tools, and custom timers in the standard library. 1. Use the library to measure execution time. 2. Use gprof for performance analysis. The steps include adding the -pg option during compilation, running the program to generate a gmon.out file, and generating a performance report. 3. Use Valgrind's Callgrind module to perform more detailed analysis. The steps include running the program to generate the callgrind.out file and viewing the results using kcachegrind. 4. Custom timers can flexibly measure the execution time of a specific code segment. These methods help to fully understand thread performance and optimize code.
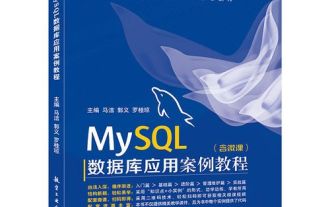
Efficient methods for batch inserting data in MySQL include: 1. Using INSERTINTO...VALUES syntax, 2. Using LOADDATAINFILE command, 3. Using transaction processing, 4. Adjust batch size, 5. Disable indexing, 6. Using INSERTIGNORE or INSERT...ONDUPLICATEKEYUPDATE, these methods can significantly improve database operation efficiency.
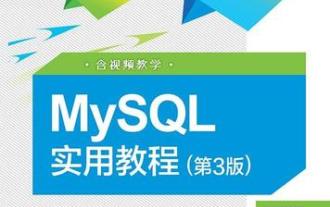
To safely and thoroughly uninstall MySQL and clean all residual files, follow the following steps: 1. Stop MySQL service; 2. Uninstall MySQL packages; 3. Clean configuration files and data directories; 4. Verify that the uninstallation is thorough.
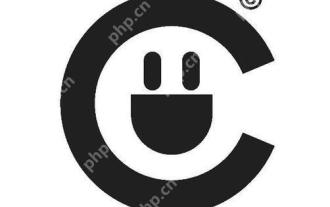
C code optimization can be achieved through the following strategies: 1. Manually manage memory for optimization use; 2. Write code that complies with compiler optimization rules; 3. Select appropriate algorithms and data structures; 4. Use inline functions to reduce call overhead; 5. Apply template metaprogramming to optimize at compile time; 6. Avoid unnecessary copying, use moving semantics and reference parameters; 7. Use const correctly to help compiler optimization; 8. Select appropriate data structures, such as std::vector.
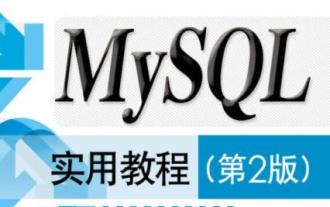
MySQL functions can be used for data processing and calculation. 1. Basic usage includes string processing, date calculation and mathematical operations. 2. Advanced usage involves combining multiple functions to implement complex operations. 3. Performance optimization requires avoiding the use of functions in the WHERE clause and using GROUPBY and temporary tables.
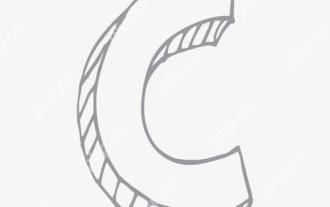
C performs well in real-time operating system (RTOS) programming, providing efficient execution efficiency and precise time management. 1) C Meet the needs of RTOS through direct operation of hardware resources and efficient memory management. 2) Using object-oriented features, C can design a flexible task scheduling system. 3) C supports efficient interrupt processing, but dynamic memory allocation and exception processing must be avoided to ensure real-time. 4) Template programming and inline functions help in performance optimization. 5) In practical applications, C can be used to implement an efficient logging system.
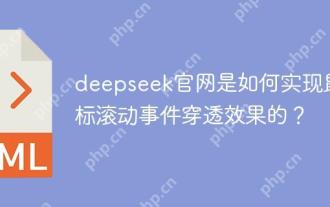
How to achieve the effect of mouse scrolling event penetration? When we browse the web, we often encounter some special interaction designs. For example, on deepseek official website, �...
