PyTorch's performance optimization strategy on CentOS
Detailed explanation of PyTorch performance optimization strategy under CentOS system
This article will explore in-depth how to optimize PyTorch performance on CentOS system to improve the training and inference efficiency of deep learning models. Optimization strategies cover data loading, data manipulation, model architecture, distributed training, and other advanced techniques.
1. Data loading optimization
- Using SSD SSD: Migrate datasets to SSD, significantly improving I/O speed.
- Asynchronous data loading: Use
num_workers
parameter to enable asynchronous data loading, process data preparation and model training in parallel, and speed up the training process. - Fixed memory: Set
pin_memory=True
to reduce the data transmission delay between the CPU and GPU.
2. Data operation optimization
- Create tensors directly on the device: Create
torch.tensor
directly on the target device (GPU) to avoid unnecessary data transmission across devices. - Minimize data transmission: minimize data interaction between CPU and GPU, and put the calculations on the GPU as much as possible.
3. Model architecture optimization
- Mixed precision training: Use mixed precision training (such as FP16) to speed up the training process while ensuring model accuracy.
- Optimize batch size: Set the batch size to multiples of 8 to make full use of GPU memory.
- Turn off convolutional layer bias: For convolutional neural networks, turning off the bias of convolutional layers before batch normalization may improve performance.
4. Distributed training optimization
- Use
DistributedDataParallel
: UseDistributedDataParallel
instead ofDataParallel
to improve the efficiency and scalability of distributed training.
V. Other advanced optimization strategies
- Enable CuDNN automatic adjustment: Set
torch.backends.cudnn.benchmark = True
to allow CuDNN to automatically select the best convolution algorithm. - Use
channels_last
memory format: For convolutional neural networks, usingchannels_last
memory format can further improve GPU performance.
6. Performance analysis and optimization
- PyTorch Profiler: Use the PyTorch Profiler tool to analyze code performance bottlenecks and optimize it in a targeted manner.
7. Installation and configuration
- Installation preparation: Ensure that the system meets the installation requirements of PyTorch, including the operating system version, Python environment and necessary package management tools.
- Install PyTorch: Use
pip
orconda
to select the appropriate installation method according to the system configuration. - Installation Verification: Run a simple PyTorch script to verify that the installation is successful.
Through the rational use of the above strategies, you can significantly improve the performance of PyTorch on CentOS systems, thereby accelerating the training and inference process of deep learning models. Remember, the best optimization strategy depends on the specific model and data set and needs to be adjusted and tested according to actual conditions.
The above is the detailed content of PyTorch's performance optimization strategy on CentOS. For more information, please follow other related articles on the PHP Chinese website!

Hot AI Tools

Undresser.AI Undress
AI-powered app for creating realistic nude photos

AI Clothes Remover
Online AI tool for removing clothes from photos.

Undress AI Tool
Undress images for free

Clothoff.io
AI clothes remover

Video Face Swap
Swap faces in any video effortlessly with our completely free AI face swap tool!

Hot Article

Hot Tools

Notepad++7.3.1
Easy-to-use and free code editor

SublimeText3 Chinese version
Chinese version, very easy to use

Zend Studio 13.0.1
Powerful PHP integrated development environment

Dreamweaver CS6
Visual web development tools

SublimeText3 Mac version
God-level code editing software (SublimeText3)

Hot Topics










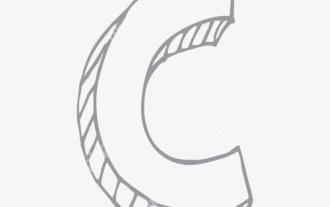
Using the chrono library in C can allow you to control time and time intervals more accurately. Let's explore the charm of this library. C's chrono library is part of the standard library, which provides a modern way to deal with time and time intervals. For programmers who have suffered from time.h and ctime, chrono is undoubtedly a boon. It not only improves the readability and maintainability of the code, but also provides higher accuracy and flexibility. Let's start with the basics. The chrono library mainly includes the following key components: std::chrono::system_clock: represents the system clock, used to obtain the current time. std::chron
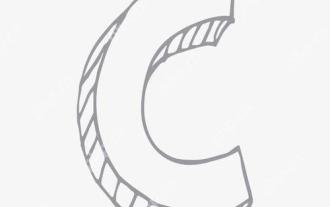
DMA in C refers to DirectMemoryAccess, a direct memory access technology, allowing hardware devices to directly transmit data to memory without CPU intervention. 1) DMA operation is highly dependent on hardware devices and drivers, and the implementation method varies from system to system. 2) Direct access to memory may bring security risks, and the correctness and security of the code must be ensured. 3) DMA can improve performance, but improper use may lead to degradation of system performance. Through practice and learning, we can master the skills of using DMA and maximize its effectiveness in scenarios such as high-speed data transmission and real-time signal processing.
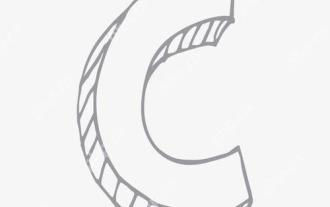
Measuring thread performance in C can use the timing tools, performance analysis tools, and custom timers in the standard library. 1. Use the library to measure execution time. 2. Use gprof for performance analysis. The steps include adding the -pg option during compilation, running the program to generate a gmon.out file, and generating a performance report. 3. Use Valgrind's Callgrind module to perform more detailed analysis. The steps include running the program to generate the callgrind.out file and viewing the results using kcachegrind. 4. Custom timers can flexibly measure the execution time of a specific code segment. These methods help to fully understand thread performance and optimize code.
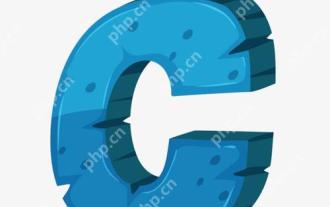
ABI compatibility in C refers to whether binary code generated by different compilers or versions can be compatible without recompilation. 1. Function calling conventions, 2. Name modification, 3. Virtual function table layout, 4. Structure and class layout are the main aspects involved.
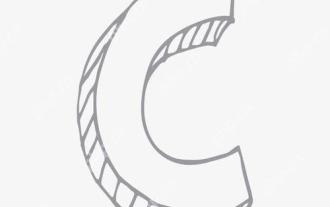
Handling high DPI display in C can be achieved through the following steps: 1) Understand DPI and scaling, use the operating system API to obtain DPI information and adjust the graphics output; 2) Handle cross-platform compatibility, use cross-platform graphics libraries such as SDL or Qt; 3) Perform performance optimization, improve performance through cache, hardware acceleration, and dynamic adjustment of the details level; 4) Solve common problems, such as blurred text and interface elements are too small, and solve by correctly applying DPI scaling.
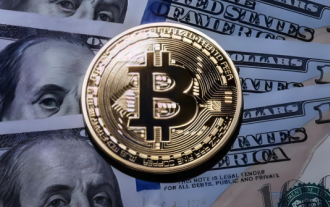
With the popularization and development of digital currency, more and more people are beginning to pay attention to and use digital currency apps. These applications provide users with a convenient way to manage and trade digital assets. So, what kind of software is a digital currency app? Let us have an in-depth understanding and take stock of the top ten digital currency apps in the world.
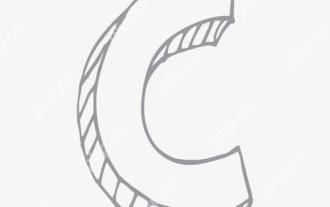
C performs well in real-time operating system (RTOS) programming, providing efficient execution efficiency and precise time management. 1) C Meet the needs of RTOS through direct operation of hardware resources and efficient memory management. 2) Using object-oriented features, C can design a flexible task scheduling system. 3) C supports efficient interrupt processing, but dynamic memory allocation and exception processing must be avoided to ensure real-time. 4) Template programming and inline functions help in performance optimization. 5) In practical applications, C can be used to implement an efficient logging system.
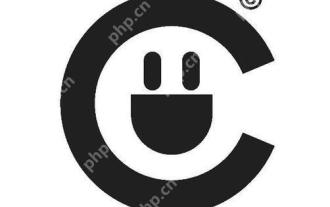
C code optimization can be achieved through the following strategies: 1. Manually manage memory for optimization use; 2. Write code that complies with compiler optimization rules; 3. Select appropriate algorithms and data structures; 4. Use inline functions to reduce call overhead; 5. Apply template metaprogramming to optimize at compile time; 6. Avoid unnecessary copying, use moving semantics and reference parameters; 7. Use const correctly to help compiler optimization; 8. Select appropriate data structures, such as std::vector.
