What is Heuristic Function in AI? - Analytics Vidhya
Introduction
Imagine navigating a complex maze – your goal is to escape as quickly as possible. How many paths exist? Now, picture having a map that highlights promising routes and dead ends. That's the essence of heuristic functions in artificial intelligence. These intelligent guides help AI systems make better, faster decisions, significantly simplifying problem-solving. This article explores heuristic functions, their role in AI, and their impact on problem-solving efficiency, highlighting their indispensable nature in the AI toolkit.
Key Takeaways
- Understand the function and role of heuristic functions within AI search algorithms.
- Learn how heuristic functions improve AI problem-solving capabilities.
- Explore various types of heuristic functions and their applications.
- Identify challenges and limitations associated with heuristic functions.
- Grasp methods for evaluating and optimizing heuristic functions in AI systems.
Table of contents
- What are Heuristic Functions?
- Types of Heuristic Functions
- Pathfinding with Heuristic Functions
- The Significance of Heuristic Functions in AI
- Applications of Heuristic Functions
- Challenges and Limitations
- Frequently Asked Questions
What are Heuristic Functions?
A heuristic function provides an estimated cost or distance between a given state and the desired goal within a search algorithm. This estimation allows the algorithm to prioritize promising paths, increasing the likelihood of finding a solution efficiently. Essentially, it offers direction, minimizing the search space and improving overall efficiency.
Types of Heuristic Functions
Heuristic functions vary in their accuracy and impact on algorithm performance. Let's examine key types:
Admissible Heuristics
An admissible heuristic never overestimates the actual cost to reach the goal. It provides a lower or equal estimate, ensuring the algorithm finds the optimal solution. This is crucial in algorithms like A*, where optimality is paramount.
Example: In A*, the straight-line distance (Euclidean distance) between nodes is an admissible heuristic.
Inadmissible Heuristics
Inadmissible heuristics can overestimate the cost to the goal. While not guaranteeing optimal solutions, they can significantly speed up the search process when speed is prioritized over absolute accuracy.
Example: Situations where computational cost reduction outweighs the need for an optimal solution might benefit from an inadmissible heuristic.
Consistent (Monotonic) Heuristics
A consistent heuristic ensures that the estimated cost to the goal decreases monotonically as the algorithm progresses. All consistent heuristics are admissible.
Example: In a maze, the cost of moving from one room to an adjacent room should not exceed the cost of moving from the previous room directly to the goal.
Dominating Heuristics
A dominating heuristic outperforms another if it provides higher (but still admissible) estimates without overestimation. Better heuristics lead to fewer paths explored.
Example: In graph traversal, a heuristic incorporating both distance and terrain difficulty dominates one considering only distance.
Pathfinding with Heuristic Functions
Heuristic functions are vital in pathfinding algorithms like A, used extensively in GPS navigation, robotics, and game development. Let's illustrate A with a code example and demonstrate the heuristic's role in improving search efficiency.
Problem Definition
We'll represent a grid where 0 denotes free space and 1 represents obstacles. The task is to find the shortest path from the top-left corner (start) to the bottom-right corner (goal), avoiding obstacles. The heuristic function guides path selection.
Heuristic: Euclidean Distance
We use Euclidean distance as our heuristic:
This estimates the straight-line distance from a node to the goal, prioritizing closer nodes.
A* Algorithm Walkthrough
The A* algorithm, incorporating the heuristic, works as follows:
Step 1: Heuristic Function
The Euclidean distance heuristic estimates the distance from the current node to the goal, guiding node prioritization.
Step 2: Neighbor Exploration
The algorithm explores neighboring nodes, adding valid (unblocked, in-bounds) neighbors to an open list.
Step 3: Node Prioritization
The open list is a priority queue, ordering nodes by their total estimated cost (f = g h), where g is the cost from the start and h is the heuristic estimate.
Step 4: Path Reconstruction
Upon reaching the goal, the algorithm reconstructs the shortest path using a backtracking mechanism.
(The detailed code implementation for A is omitted for brevity but would follow standard A algorithm structure, using the defined heuristic function.)
The Significance of Heuristic Functions in AI
Heuristic functions are crucial in AI, particularly for problems with large search spaces. Without them, algorithms would exhaustively explore all possibilities, leading to exponential increases in computation time and resource consumption. Their importance stems from:
- Efficiency: Heuristics drastically reduce the number of paths explored, saving time and computational resources.
- Scalability: They enable the application of algorithms to larger, more complex problems.
- Problem-Specific Knowledge: They leverage domain-specific knowledge to improve search effectiveness.
Applications of Heuristic Functions
Heuristic functions find widespread use in:
- Pathfinding: A* and Dijkstra's algorithm in GPS navigation and robotics.
- Game AI: Evaluating move outcomes in games like chess.
- Optimization: Finding near-optimal solutions to problems like the traveling salesman problem.
- Constraint Satisfaction: Guiding the search for solutions that satisfy all constraints.
Challenges and Limitations
Despite their benefits, heuristic functions have limitations:
- Design Complexity: Creating effective heuristics requires careful design and domain expertise.
- Problem Specificity: Heuristics are often problem-specific, limiting their generalizability.
- Computational Overhead: Calculating complex heuristics can add computational cost.
- Suboptimal Solutions: Inadmissible heuristics risk finding suboptimal solutions.
Conclusion
Heuristic functions are fundamental to AI, powering many search algorithms and problem-solving techniques. Their ability to provide informed guidance makes AI systems more efficient and practical. However, effective design and optimization are crucial for maximizing their benefits.
Frequently Asked Questions
Q1. What is a heuristic function in AI?
A1. A heuristic function estimates the cost or distance from a current state to a goal state, guiding search algorithms.
Q2. Why are heuristic functions important?
A2. They enable efficient navigation of complex search spaces by prioritizing promising paths.
Q3. What are admissible heuristics?
A3. Admissible heuristics never overestimate the cost to reach the goal, guaranteeing optimal solutions (in algorithms like A*).
Q4. Do heuristic functions always guarantee optimal solutions?
A4. No. While admissible heuristics do, inadmissible heuristics may provide faster but suboptimal solutions.
Q5. Where are heuristic functions commonly used?
A5. In pathfinding, game AI, optimization problems, and constraint satisfaction problems.
The above is the detailed content of What is Heuristic Function in AI? - Analytics Vidhya. For more information, please follow other related articles on the PHP Chinese website!

Hot AI Tools

Undresser.AI Undress
AI-powered app for creating realistic nude photos

AI Clothes Remover
Online AI tool for removing clothes from photos.

Undress AI Tool
Undress images for free

Clothoff.io
AI clothes remover

Video Face Swap
Swap faces in any video effortlessly with our completely free AI face swap tool!

Hot Article

Hot Tools

Notepad++7.3.1
Easy-to-use and free code editor

SublimeText3 Chinese version
Chinese version, very easy to use

Zend Studio 13.0.1
Powerful PHP integrated development environment

Dreamweaver CS6
Visual web development tools

SublimeText3 Mac version
God-level code editing software (SublimeText3)

Hot Topics










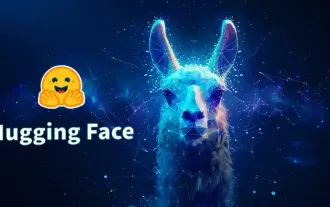
Meta's Llama 3.2: A Leap Forward in Multimodal and Mobile AI Meta recently unveiled Llama 3.2, a significant advancement in AI featuring powerful vision capabilities and lightweight text models optimized for mobile devices. Building on the success o
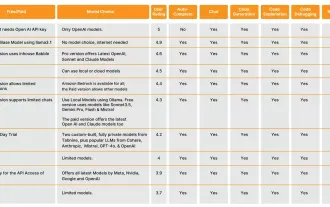
Hey there, Coding ninja! What coding-related tasks do you have planned for the day? Before you dive further into this blog, I want you to think about all your coding-related woes—better list those down. Done? – Let’
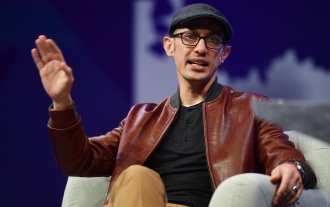
Shopify CEO Tobi Lütke's recent memo boldly declares AI proficiency a fundamental expectation for every employee, marking a significant cultural shift within the company. This isn't a fleeting trend; it's a new operational paradigm integrated into p
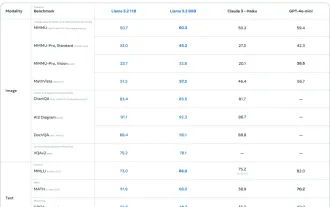
This week's AI landscape: A whirlwind of advancements, ethical considerations, and regulatory debates. Major players like OpenAI, Google, Meta, and Microsoft have unleashed a torrent of updates, from groundbreaking new models to crucial shifts in le
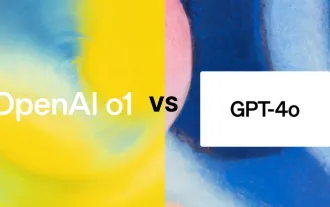
Introduction OpenAI has released its new model based on the much-anticipated “strawberry” architecture. This innovative model, known as o1, enhances reasoning capabilities, allowing it to think through problems mor
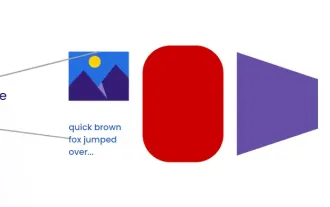
Introduction Imagine walking through an art gallery, surrounded by vivid paintings and sculptures. Now, what if you could ask each piece a question and get a meaningful answer? You might ask, “What story are you telling?
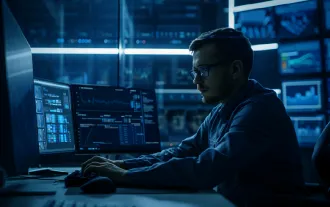
SQL's ALTER TABLE Statement: Dynamically Adding Columns to Your Database In data management, SQL's adaptability is crucial. Need to adjust your database structure on the fly? The ALTER TABLE statement is your solution. This guide details adding colu
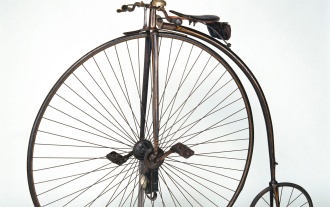
The 2025 Artificial Intelligence Index Report released by the Stanford University Institute for Human-Oriented Artificial Intelligence provides a good overview of the ongoing artificial intelligence revolution. Let’s interpret it in four simple concepts: cognition (understand what is happening), appreciation (seeing benefits), acceptance (face challenges), and responsibility (find our responsibilities). Cognition: Artificial intelligence is everywhere and is developing rapidly We need to be keenly aware of how quickly artificial intelligence is developing and spreading. Artificial intelligence systems are constantly improving, achieving excellent results in math and complex thinking tests, and just a year ago they failed miserably in these tests. Imagine AI solving complex coding problems or graduate-level scientific problems – since 2023
