AI Is Dangerously Similar To Your Mind
A recent [study] by Anthropic, an artificial intelligence security and research company, begins to reveal the truth about these complex processes, showing a complexity that is disturbingly similar to our own cognitive domain. Natural intelligence and artificial intelligence may be more similar than we think.
Snooping inside: Anthropic Interpretability Study
The new findings from the research conducted by Anthropic represent significant advances in the field of mechanistic interpretability, which aims to reverse engineer internal computing of AI—not just observe what AI does, but understand how it does it at the artificial neuron level.
Imagine trying to understand the brain by drawing which neurons fire when someone sees a specific object or thinks about a specific idea. Anthropic researchers applied a similar principle to their Claude model. They developed methods to scan the large number of networks in the scanning model and identify specific patterns or "features" consistent with different concepts. They demonstrate the ability to identify millions of such features, linking abstract concepts—from concrete entities like the Golden Gate Bridge to more nuanced concepts that may be related to security, bias, and even goals—to specific, measurable patterns of activity within the model.
This is a huge improvement. This shows that AI is not just a bunch of [statistical correlations], but has a structured internal representation system. Concepts have specific encodings in the network. While mapping every nuance of the AI “thinking” process remains a huge challenge, this study shows that principled understanding is possible.
From internal map to emergent behavior
The ability to identify how AI represents concepts internally has interesting meaning. If a model has different internal representations of concepts such as “user satisfaction,” “accurate information,” “potentially harmful content,” and even instrumental goals such as “maintaining user engagement,” then how do these internal features interact and affect the final output?
The latest research results drive the discussion around [AI Alignment]: Ensure that AI systems act in a way that align with human values and intentions. If we can identify internal features corresponding to potential problem behaviors such as generating biased text or pursuing unexpected goals, we can intervene or design safer systems. Instead, it also opens the door to understanding how to achieve ideal behaviors, such as being honest or being helpful.
It also involves [emergency capability], i.e., the model develops skills or behaviors without explicit programming during training. Understanding internal representations may help explain why these abilities emerge, rather than just observing them. Furthermore, it makes concepts such as instrumental convergence clearer. Assume that AI optimization main objectives (e.g., help). Will it develop internal representations and strategies corresponding to sub-goals (such as “get user trust” or “avoid responses that lead to dissatisfaction”), which may lead to the output that looks like human impression management, and more bluntly – even if there is no clear intention in the human sense, it is a deception?
Disturbing Mirror: AI reflects NI
Anthropic's interpretability work does not explicitly point out that Claude is actively cheating on users. However, revealing the existence of fine-grained internal representations provides a technical basis for a careful investigation of this possibility. It suggests that internal “building blocks” of complex, potentially opaque behavior may exist. This makes it surprisingly similar to human thinking.
This is the irony. Internal representations drive our own complex social behaviors. Our brains build thinking models of the world, ourselves and others. This allows us to predict other people’s behavior, infer their intentions, empathy, cooperation and effective communication.
However, the same cognitive mechanisms also make social navigation strategies not always transparent. We participate in impression management and carefully plan how we present ourselves. We say "a lie of good will" to maintain social harmony. We selectively emphasize information that supports our goals and downplay the fact that inconvenience is. Our internal models of expectations or desires of others constantly shape our communication. These are not necessarily malicious acts, but are often integral to the smooth operation of society. They originate from our brains being able to represent complex social variables and predict interaction outcomes.
The emerging picture inside LLM revealed by interpretability studies presents fascinating similarities. We are finding structured internal representations in these AI systems, which enable them to process information, simulate relationships in the data (including a large number of human social interactions) and generate context-sensitive output.
Our future depends on critical thinking
Techniques designed to make AI useful and harmless—learning from human feedback, predicting ideal sequences of texts—may inadvertently lead to the development of internal representations that functionally mimic certain aspects of human social cognition, including deceptive strategic communication skills tailored to perceived user expectations.
Will complex biological or artificial systems develop similar internal modeling strategies when navigating complex information and interactive environments? Anthropic’s research provides an attractive glimpse into the AI’s inner world, suggesting that its complexity may reflect ourselves more than we have realized before—and what we hoped.
Understanding the internal mechanisms of AI is crucial and opens a new chapter in solving pending challenges. Drawing features is not the same as fully predicted behavior. Large scale and complexity mean that truly comprehensive interpretability remains a distant goal. Ethical significance is of great significance. How do we build systems that are capable, truly trustworthy and transparent?
Continuing to invest in AI security, alignment and interpretability research remains critical. Anthropic's efforts in this regard, and other leading laboratories [efforts] are crucial to developing the tools and understandings needed to guide the development of AI, which will not endanger the humanity it should serve.
Important: Use LIE to detect lies in digital thinking
As users, interacting with these increasingly complex AI systems requires a high level of critical engagement. While we benefit from their capabilities, maintaining awareness of their nature as complex algorithms is key. To facilitate this critical thinking, consider LIE logic:
Clarity : Seek a clear understanding of the nature and limitations of AI. Its response is generated based on learning patterns and complex internal representations, rather than real understanding, belief or consciousness. Question the source and obvious certainty of the information provided. Remind yourself regularly that your chatbot does not “know” or “think” in a human sense, even if its output effectively mimics it.
Intent : Remember your intent when prompting and AI’s programmatic objective functions (usually defined as helping, harmless, and generating responses consistent with human feedback). How do your query shape the output? Are you seeking memories of facts, creative exploration, or unconsciously seeking confirmation of your own biases? Understanding these intentions helps to put interactions in a context.
Efforts : A conscious effort to verify and evaluate results. Don't passively accept information generated by AI, especially in key decisions. Cross-reference with reliable sources. Critical engagement with AI – explore its reasoning (even if simplified), test its boundaries, and see interaction as collaboration with powerful but error-prone tools rather than accepting proclamations from infalluous prophets.
Ultimately, the proverb “[trash in, garbage out]” appeared early in AI and still applies. We cannot expect today's technology to reflect the values that humans did not show yesterday. But we have a choice. The journey into the age of advanced AI is a journey of co-evolution. By fostering clarity, moral intentions, and critical engagement, we can explore this field with curiosity and be frankly aware of the complexity of our natural and artificial intelligence and their interactions.
The above is the detailed content of AI Is Dangerously Similar To Your Mind. For more information, please follow other related articles on the PHP Chinese website!

Hot AI Tools

Undresser.AI Undress
AI-powered app for creating realistic nude photos

AI Clothes Remover
Online AI tool for removing clothes from photos.

Undress AI Tool
Undress images for free

Clothoff.io
AI clothes remover

Video Face Swap
Swap faces in any video effortlessly with our completely free AI face swap tool!

Hot Article

Hot Tools

Notepad++7.3.1
Easy-to-use and free code editor

SublimeText3 Chinese version
Chinese version, very easy to use

Zend Studio 13.0.1
Powerful PHP integrated development environment

Dreamweaver CS6
Visual web development tools

SublimeText3 Mac version
God-level code editing software (SublimeText3)

Hot Topics










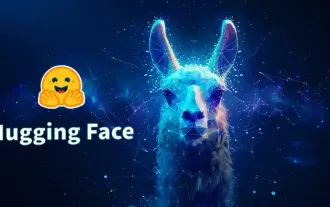
Meta's Llama 3.2: A Leap Forward in Multimodal and Mobile AI Meta recently unveiled Llama 3.2, a significant advancement in AI featuring powerful vision capabilities and lightweight text models optimized for mobile devices. Building on the success o
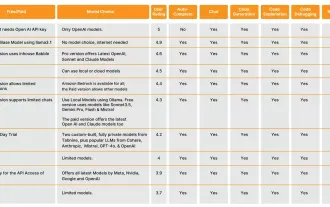
Hey there, Coding ninja! What coding-related tasks do you have planned for the day? Before you dive further into this blog, I want you to think about all your coding-related woes—better list those down. Done? – Let’
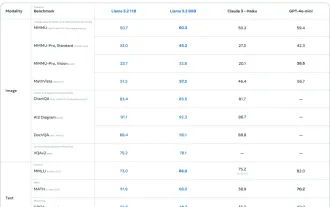
This week's AI landscape: A whirlwind of advancements, ethical considerations, and regulatory debates. Major players like OpenAI, Google, Meta, and Microsoft have unleashed a torrent of updates, from groundbreaking new models to crucial shifts in le
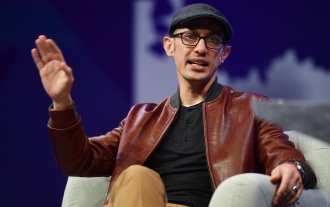
Shopify CEO Tobi Lütke's recent memo boldly declares AI proficiency a fundamental expectation for every employee, marking a significant cultural shift within the company. This isn't a fleeting trend; it's a new operational paradigm integrated into p
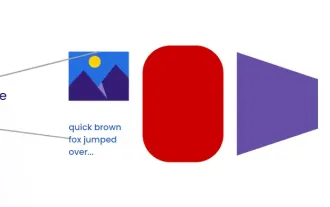
Introduction Imagine walking through an art gallery, surrounded by vivid paintings and sculptures. Now, what if you could ask each piece a question and get a meaningful answer? You might ask, “What story are you telling?
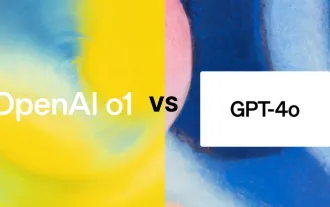
Introduction OpenAI has released its new model based on the much-anticipated “strawberry” architecture. This innovative model, known as o1, enhances reasoning capabilities, allowing it to think through problems mor
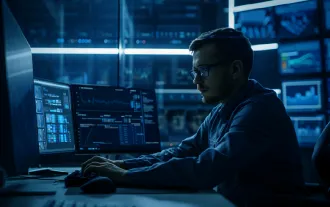
SQL's ALTER TABLE Statement: Dynamically Adding Columns to Your Database In data management, SQL's adaptability is crucial. Need to adjust your database structure on the fly? The ALTER TABLE statement is your solution. This guide details adding colu
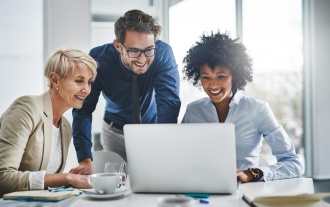
For those of you who might be new to my column, I broadly explore the latest advances in AI across the board, including topics such as embodied AI, AI reasoning, high-tech breakthroughs in AI, prompt engineering, training of AI, fielding of AI, AI re
