Google's SigLIP: A Significant Momentum in CLIP's Framework
Google's SigLIP: A Superior Image Classification Model
Image classification has revolutionized computer vision, delivering more accurate results through advanced models. Zero-shot classification and image-pair analysis are particularly prominent applications. Google's SigLIP model stands out, boasting impressive performance benchmarks. It's an image embedding model based on the CLIP framework, but enhanced with a superior sigmoid loss function.
SigLIP processes image-text pairs, generating vector representations and probabilities. Its efficiency allows for classification even with smaller datasets while maintaining scalability. The key differentiator is the sigmoid loss function, surpassing CLIP's performance by focusing on individual image-text pair matches rather than overall best matches.
Key Features and Capabilities:
- Multimodal Model: Combines image and text processing for enhanced accuracy.
- Vision Transformer Encoder: Divides images into patches for efficient vector embedding.
- Transformer Encoder for Text: Converts text sequences into dense embeddings.
- Zero-Shot Classification: Classifies images without prior training on specific labels.
- Image-Text Similarity Scores: Provides scores reflecting the similarity between images and their descriptions.
- Scalable Architecture: Handles large datasets efficiently thanks to the sigmoid loss function.
Model Architecture:
SigLIP employs a CLIP-like architecture but with crucial modifications. The image undergoes processing via a vision transformer encoder, while text is handled by a transformer encoder. This multimodal approach allows for both image-based and text-based input, enabling diverse applications.
The model's contrastive learning framework aligns image and text representations, improving overall performance.
Performance and Scalability:
The sigmoid loss function allows for significant scaling improvements compared to CLIP. While further optimization is ongoing (e.g., with SoViT-400m), SigLIP already shows promising results.
Inference with SigLIP:
Here's a simplified guide to running inference:
-
Import Libraries: Use
transformers
,PIL
, andrequests
. -
Load the Model: Employ the
pipeline
function fromtransformers
to load the pre-trainedgoogle/siglip-so400m-patch14-384
model. -
Prepare the Image: Load the image using PIL from a local path or URL via
requests
. -
Perform Inference: Use the loaded model to obtain the
logits
(scores) for the image against candidate labels.
SigLIP vs. CLIP:
The key advantage of SigLIP lies in its sigmoid loss function. Unlike CLIP's softmax, which struggles with scenarios where the image class isn't among the labels, SigLIP provides more accurate and nuanced results.
Applications:
SigLIP's capabilities extend to various applications:
- Image Search: Building search engines based on text descriptions.
- Image Captioning: Generating captions for images.
- Visual Question Answering: Answering questions about images.
Conclusion:
Google's SigLIP represents a significant advancement in image classification. Its sigmoid loss function and efficient architecture lead to improved accuracy and scalability, making it a powerful tool for various computer vision tasks.
Key Takeaways:
- SigLIP utilizes a sigmoid loss function for superior zero-shot classification performance.
- Its multimodal approach enhances accuracy and versatility.
- It's highly scalable and suitable for large-scale applications.
Resources:
Frequently Asked Questions:
-
Q1: What's the core difference between SigLIP and CLIP? A1: SigLIP employs a sigmoid loss function for improved accuracy in zero-shot classification.
-
Q2: What are SigLIP's primary applications? A2: Image classification, captioning, retrieval, and visual question answering.
-
Q3: How does SigLIP handle zero-shot classification? A3: By comparing images to provided text labels, even without prior training on those labels.
-
Q4: Why is the sigmoid loss function beneficial? A4: It allows for independent evaluation of image-text pairs, leading to more accurate predictions.
(Note: Replace "https://www.php.cn/https://www.php.cn/https://www.php.cn/link/2bec63f5d312303621583b97ff7c68bf/2bec63f5d312303621583b97ff7c68bf/2bec63f5d312303621583b97ff7c68bf" placeholders with actual https://www.php.cn/https://www.php.cn/https://www.php.cn/link/2bec63f5d312303621583b97ff7c68bf/2bec63f5d312303621583b97ff7c68bf/2bec63f5d312303621583b97ff7c68bfs to the resources.)
The above is the detailed content of Google's SigLIP: A Significant Momentum in CLIP's Framework. For more information, please follow other related articles on the PHP Chinese website!

Hot AI Tools

Undresser.AI Undress
AI-powered app for creating realistic nude photos

AI Clothes Remover
Online AI tool for removing clothes from photos.

Undress AI Tool
Undress images for free

Clothoff.io
AI clothes remover

Video Face Swap
Swap faces in any video effortlessly with our completely free AI face swap tool!

Hot Article

Hot Tools

Notepad++7.3.1
Easy-to-use and free code editor

SublimeText3 Chinese version
Chinese version, very easy to use

Zend Studio 13.0.1
Powerful PHP integrated development environment

Dreamweaver CS6
Visual web development tools

SublimeText3 Mac version
God-level code editing software (SublimeText3)

Hot Topics
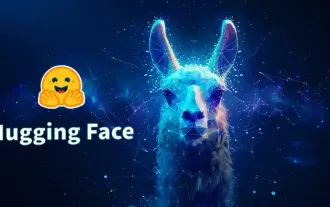
Meta's Llama 3.2: A Leap Forward in Multimodal and Mobile AI Meta recently unveiled Llama 3.2, a significant advancement in AI featuring powerful vision capabilities and lightweight text models optimized for mobile devices. Building on the success o
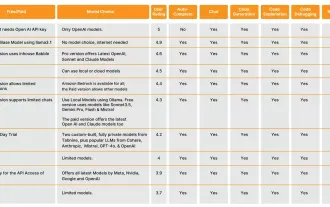
Hey there, Coding ninja! What coding-related tasks do you have planned for the day? Before you dive further into this blog, I want you to think about all your coding-related woes—better list those down. Done? – Let’
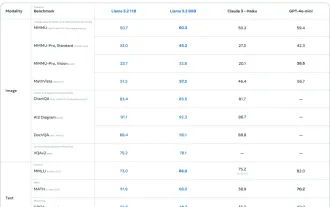
This week's AI landscape: A whirlwind of advancements, ethical considerations, and regulatory debates. Major players like OpenAI, Google, Meta, and Microsoft have unleashed a torrent of updates, from groundbreaking new models to crucial shifts in le
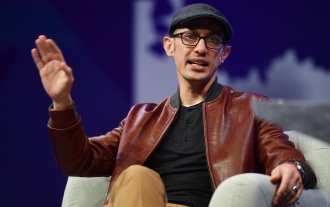
Shopify CEO Tobi Lütke's recent memo boldly declares AI proficiency a fundamental expectation for every employee, marking a significant cultural shift within the company. This isn't a fleeting trend; it's a new operational paradigm integrated into p
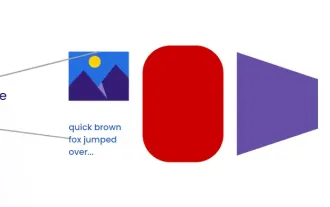
Introduction Imagine walking through an art gallery, surrounded by vivid paintings and sculptures. Now, what if you could ask each piece a question and get a meaningful answer? You might ask, “What story are you telling?
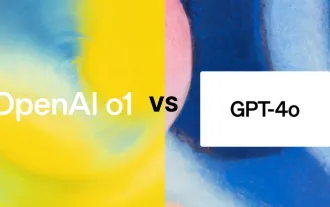
Introduction OpenAI has released its new model based on the much-anticipated “strawberry” architecture. This innovative model, known as o1, enhances reasoning capabilities, allowing it to think through problems mor
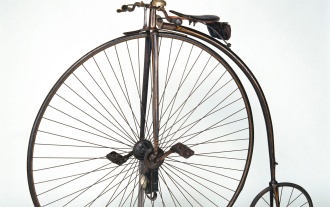
The 2025 Artificial Intelligence Index Report released by the Stanford University Institute for Human-Oriented Artificial Intelligence provides a good overview of the ongoing artificial intelligence revolution. Let’s interpret it in four simple concepts: cognition (understand what is happening), appreciation (seeing benefits), acceptance (face challenges), and responsibility (find our responsibilities). Cognition: Artificial intelligence is everywhere and is developing rapidly We need to be keenly aware of how quickly artificial intelligence is developing and spreading. Artificial intelligence systems are constantly improving, achieving excellent results in math and complex thinking tests, and just a year ago they failed miserably in these tests. Imagine AI solving complex coding problems or graduate-level scientific problems – since 2023
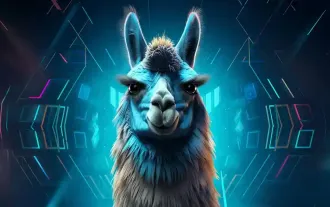
Meta's Llama 3.2: A Multimodal AI Powerhouse Meta's latest multimodal model, Llama 3.2, represents a significant advancement in AI, boasting enhanced language comprehension, improved accuracy, and superior text generation capabilities. Its ability t
