Automating CSV to PostgreSQL Ingestion with Airflow and Docker
This tutorial demonstrates building a robust data pipeline using Apache Airflow, Docker, and PostgreSQL to automate data transfer from CSV files to a database. We'll cover core Airflow concepts like DAGs, tasks, and operators for efficient workflow management.
This project showcases creating a reliable data pipeline that reads CSV data and writes it to a PostgreSQL database. We'll integrate various Airflow components to ensure efficient data handling and maintain data integrity.
Learning Objectives:
- Grasp core Apache Airflow concepts: DAGs, tasks, and operators.
- Set up and configure Apache Airflow with Docker for workflow automation.
- Integrate PostgreSQL for data management within Airflow pipelines.
- Master reading CSV files and automating data insertion into a PostgreSQL database.
- Build and deploy scalable, efficient data pipelines using Airflow and Docker.
Prerequisites:
- Docker Desktop, VS Code, Docker Compose
- Basic understanding of Docker containers and commands
- Basic Linux commands
- Basic Python knowledge
- Experience building Docker images from Dockerfiles and using Docker Compose
What is Apache Airflow?
Apache Airflow (Airflow) is a platform for programmatically authoring, scheduling, and monitoring workflows. Defining workflows as code improves maintainability, version control, testing, and collaboration. Its user interface simplifies visualizing pipelines, monitoring progress, and troubleshooting.
Airflow Terminology:
- Workflow: A step-by-step process to achieve a goal (e.g., baking a cake).
-
DAG (Directed Acyclic Graph): A workflow blueprint showing task dependencies and execution order. It's a visual representation of the workflow.
- Task: A single action within a workflow (e.g., mixing ingredients).
-
Operators: Building blocks of tasks, defining actions like running Python scripts or executing SQL. Key operators include
PythonOperator
,DummyOperator
, andPostgresOperator
. - XComs (Cross-Communications): Enable tasks to communicate and share data.
- Connections: Manage credentials for connecting to external systems (e.g., databases).
Setting up Apache Airflow with Docker and Dockerfile:
Using Docker ensures a consistent and reproducible environment. A Dockerfile
automates image creation. The following instructions should be saved as Dockerfile
(no extension):
FROM apache/airflow:2.9.1-python3.9 USER root COPY requirements.txt /requirements.txt RUN pip3 install --upgrade pip && pip3 install --no-cache-dir -r /requirements.txt RUN pip3 install apache-airflow-providers-apache-spark apache-airflow-providers-amazon RUN apt-get update && apt-get install -y gcc python3-dev openjdk-17-jdk && apt-get clean
This Dockerfile
uses an official Airflow image, installs dependencies from requirements.txt
, and installs necessary Airflow providers (Spark and AWS examples are shown; you may need others).
Docker Compose Configuration:
docker-compose.yml
orchestrates the Docker containers. The following configuration defines services for the webserver, scheduler, triggerer, CLI, init, and PostgreSQL. Note the use of the x-airflow-common
section for shared settings and the connection to the PostgreSQL database. (The full docker-compose.yml
is too long to include here but the key sections are shown above).
Project Setup and Execution:
- Create a project directory.
- Add the
Dockerfile
anddocker-compose.yml
files. - Create
requirements.txt
listing necessary Python packages (e.g., pandas). - Run
docker-compose up -d
to start the containers. - Access the Airflow UI at
http://localhost:8080
. - Create a PostgreSQL connection in the Airflow UI (using
write_to_psql
as the connection ID). - Create a sample
input.csv
file.
DAG and Python Function:
The Airflow DAG (sample.py
) defines the workflow:
- A
PostgresOperator
creates the database table. - A
PythonOperator
(generate_insert_queries
) reads the CSV and generates SQLINSERT
statements, saving them todags/sql/insert_queries.sql
. - Another
PostgresOperator
executes the generated SQL.
(The full sample.py
code is too long to include here but the key sections are shown above).
Conclusion:
This project demonstrates a complete data pipeline using Airflow, Docker, and PostgreSQL. It highlights the benefits of automation and the use of Docker for reproducible environments. The use of operators and the DAG structure are key to efficient workflow management.
(The remaining sections, including FAQs and Github Repo, are omitted for brevity. They are present in the original input.)
The above is the detailed content of Automating CSV to PostgreSQL Ingestion with Airflow and Docker. For more information, please follow other related articles on the PHP Chinese website!

Hot AI Tools

Undresser.AI Undress
AI-powered app for creating realistic nude photos

AI Clothes Remover
Online AI tool for removing clothes from photos.

Undress AI Tool
Undress images for free

Clothoff.io
AI clothes remover

Video Face Swap
Swap faces in any video effortlessly with our completely free AI face swap tool!

Hot Article

Hot Tools

Notepad++7.3.1
Easy-to-use and free code editor

SublimeText3 Chinese version
Chinese version, very easy to use

Zend Studio 13.0.1
Powerful PHP integrated development environment

Dreamweaver CS6
Visual web development tools

SublimeText3 Mac version
God-level code editing software (SublimeText3)

Hot Topics










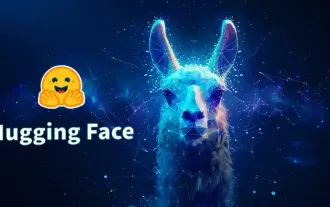
Meta's Llama 3.2: A Leap Forward in Multimodal and Mobile AI Meta recently unveiled Llama 3.2, a significant advancement in AI featuring powerful vision capabilities and lightweight text models optimized for mobile devices. Building on the success o
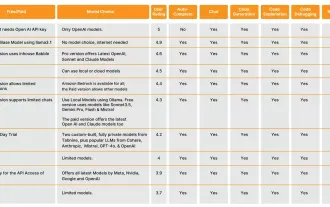
Hey there, Coding ninja! What coding-related tasks do you have planned for the day? Before you dive further into this blog, I want you to think about all your coding-related woes—better list those down. Done? – Let’
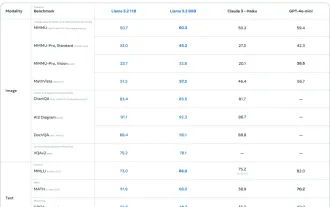
This week's AI landscape: A whirlwind of advancements, ethical considerations, and regulatory debates. Major players like OpenAI, Google, Meta, and Microsoft have unleashed a torrent of updates, from groundbreaking new models to crucial shifts in le
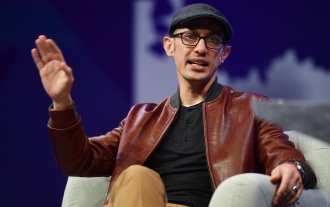
Shopify CEO Tobi Lütke's recent memo boldly declares AI proficiency a fundamental expectation for every employee, marking a significant cultural shift within the company. This isn't a fleeting trend; it's a new operational paradigm integrated into p
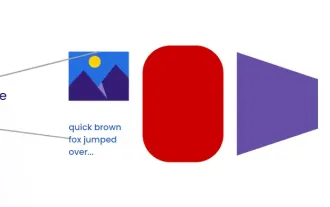
Introduction Imagine walking through an art gallery, surrounded by vivid paintings and sculptures. Now, what if you could ask each piece a question and get a meaningful answer? You might ask, “What story are you telling?
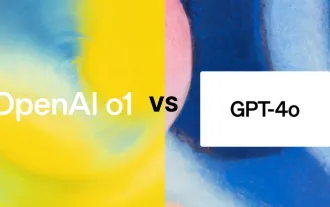
Introduction OpenAI has released its new model based on the much-anticipated “strawberry” architecture. This innovative model, known as o1, enhances reasoning capabilities, allowing it to think through problems mor
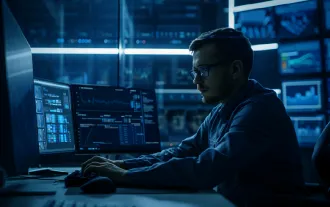
SQL's ALTER TABLE Statement: Dynamically Adding Columns to Your Database In data management, SQL's adaptability is crucial. Need to adjust your database structure on the fly? The ALTER TABLE statement is your solution. This guide details adding colu
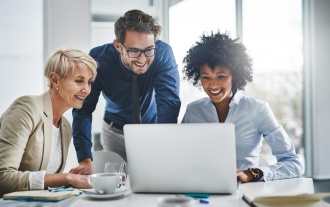
For those of you who might be new to my column, I broadly explore the latest advances in AI across the board, including topics such as embodied AI, AI reasoning, high-tech breakthroughs in AI, prompt engineering, training of AI, fielding of AI, AI re
