


MNIST handwritten numerical classification: the accuracy rate after pixel normalization is low. What is the problem?
TensorFlow MNIST classification: Causes and solutions for low accuracy after pixel normalization
When using TensorFlow for MNIST handwritten numerical classification, many developers may encounter a problem: after pixel normalization of the dataset, the accuracy of model training is extremely low. This article will analyze this problem in depth and provide solutions in conjunction with code examples.
The root cause of the problem lies in the misuse of the tf.nn.softmax_cross_entropy_with_logits
function. In the original code, the predicted value y_pred
uses the tf.nn.softmax
function to calculate the softmax probability:
y_pred = tf.nn.softmax(tf.matmul(x, w) b)
However, tf.nn.softmax_cross_entropy_with_logits
function expects the input of the linear output (logits), not the softmax probability. Pass y_pred
that has been converted into softmax to calculate the loss, resulting in errors in the calculation of the loss function, which in turn affects the model training effect.
Solution:
The key is to modify the calculation method of y_pred
and remove tf.nn.softmax
function:
y_pred = tf.matmul(x, w) b
At the same time, when calculating the accuracy, the tf.nn.softmax
function needs to be applied to y_pred
to obtain the probability distribution for comparison with the real tag:
correct_prediction = tf.equal(tf.argmax(y, 1), tf.argmax(tf.nn.softmax(y_pred), 1))
The modified code snippet is as follows (assuming some code already exists):
# ... (The code part of importing packages and setting hyperparameters remains unchanged) # Download the dataset mnist = input_data.read_data_sets('original_data/', one_hot=True) train_img = mnist.train.images train_label = mnist.train.labels test_img = mnist.test.images test_label = mnist.test.labels train_img /= 255.0 test_img /= 255.0 X = tf.compat.v1.placeholder(tf.float32, shape=[None, inputSize]) y = tf.compat.v1.placeholder(tf.float32, shape=[None, numClasses]) W = tf.Variable(tf.random_normal([inputSize, numClasses], stddev=0.1)) B = tf.Variable(tf.constant(0.1), [numClasses]) y_pred = tf.matmul(X, W) B # Modify: Remove softmax loss = tf.reduce_mean(tf.nn.softmax_cross_entropy_with_logits(labels=y, logits=y_pred)) 0.01 * tf.nn.l2_loss(W) opt = tf.train.GradientDescentOptimizer(learning_rate).minimize(loss) correct_prediction = tf.equal(tf.argmax(y, 1), tf.argmax(tf.nn.softmax(y_pred), 1)) # Modify: Apply softmax when calculating accuracy accuracy = tf.reduce_mean(tf.cast(correct_prediction, tf.float32)) saver = tf.train.Saver() multiclass_parameters = {} # ... (The running code part remains unchanged)
Through the above adjustments, tf.nn.softmax_cross_entropy_with_logits
function will receive the correct linear output and calculate the correct loss value, so that the model can be effectively trained and obtained higher accuracy. This once again emphasizes the importance of correctly understanding and using TensorFlow functions for building efficient deep learning models.
The above is the detailed content of MNIST handwritten numerical classification: the accuracy rate after pixel normalization is low. What is the problem?. For more information, please follow other related articles on the PHP Chinese website!

Hot AI Tools

Undresser.AI Undress
AI-powered app for creating realistic nude photos

AI Clothes Remover
Online AI tool for removing clothes from photos.

Undress AI Tool
Undress images for free

Clothoff.io
AI clothes remover

Video Face Swap
Swap faces in any video effortlessly with our completely free AI face swap tool!

Hot Article

Hot Tools

Notepad++7.3.1
Easy-to-use and free code editor

SublimeText3 Chinese version
Chinese version, very easy to use

Zend Studio 13.0.1
Powerful PHP integrated development environment

Dreamweaver CS6
Visual web development tools

SublimeText3 Mac version
God-level code editing software (SublimeText3)

Hot Topics
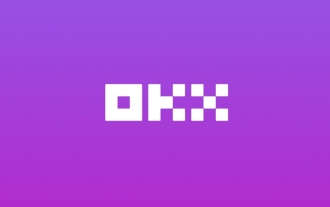
The Ouyi Exchange app supports downloading of Apple mobile phones, visit the official website, click the "Apple Mobile" option, obtain and install it in the App Store, register or log in to conduct cryptocurrency trading.
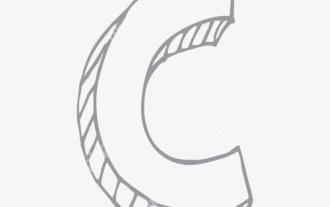
Using the chrono library in C can allow you to control time and time intervals more accurately. Let's explore the charm of this library. C's chrono library is part of the standard library, which provides a modern way to deal with time and time intervals. For programmers who have suffered from time.h and ctime, chrono is undoubtedly a boon. It not only improves the readability and maintainability of the code, but also provides higher accuracy and flexibility. Let's start with the basics. The chrono library mainly includes the following key components: std::chrono::system_clock: represents the system clock, used to obtain the current time. std::chron
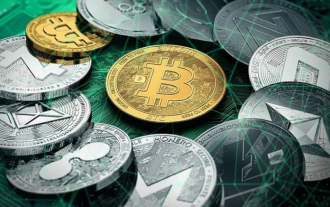
The top ten cryptocurrency trading platforms in the world include Binance, OKX, Gate.io, Coinbase, Kraken, Huobi Global, Bitfinex, Bittrex, KuCoin and Poloniex, all of which provide a variety of trading methods and powerful security measures.
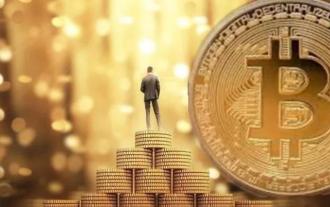
Recommended reliable digital currency trading platforms: 1. OKX, 2. Binance, 3. Coinbase, 4. Kraken, 5. Huobi, 6. KuCoin, 7. Bitfinex, 8. Gemini, 9. Bitstamp, 10. Poloniex, these platforms are known for their security, user experience and diverse functions, suitable for users at different levels of digital currency transactions
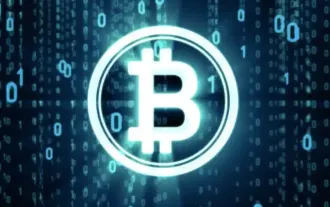
The top ten digital currency exchanges such as Binance, OKX, gate.io have improved their systems, efficient diversified transactions and strict security measures.
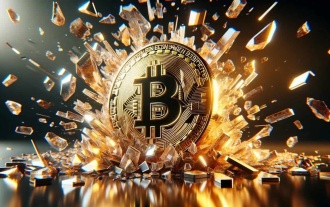
Bitcoin’s price ranges from $20,000 to $30,000. 1. Bitcoin’s price has fluctuated dramatically since 2009, reaching nearly $20,000 in 2017 and nearly $60,000 in 2021. 2. Prices are affected by factors such as market demand, supply, and macroeconomic environment. 3. Get real-time prices through exchanges, mobile apps and websites. 4. Bitcoin price is highly volatile, driven by market sentiment and external factors. 5. It has a certain relationship with traditional financial markets and is affected by global stock markets, the strength of the US dollar, etc. 6. The long-term trend is bullish, but risks need to be assessed with caution.
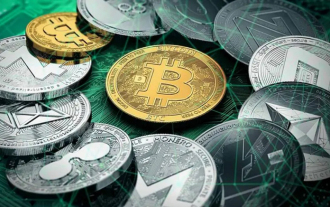
The top ten cryptocurrency exchanges in the world in 2025 include Binance, OKX, Gate.io, Coinbase, Kraken, Huobi, Bitfinex, KuCoin, Bittrex and Poloniex, all of which are known for their high trading volume and security.
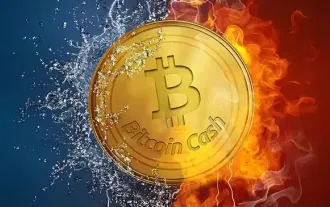
Bitcoin’s price fluctuations today are affected by many factors such as macroeconomics, policies, and market sentiment. Investors need to pay attention to technical and fundamental analysis to make informed decisions.
