Explain the concepts of Big O notation and time complexity analysis.
Explain the concepts of Big O notation and time complexity analysis.
Big O notation is a mathematical notation used in computer science to describe the performance or complexity of an algorithm. It specifically focuses on how the runtime or space requirements of an algorithm grow as the size of the input increases. Big O notation provides an upper bound on the growth rate of the algorithm, which means it describes the worst-case scenario of an algorithm's performance.
Time complexity analysis, on the other hand, is the process of determining the amount of time an algorithm takes to complete as a function of the length of the input. It is typically expressed using Big O notation. Time complexity analysis helps in understanding how the execution time of an algorithm scales with the size of the input data. This analysis is crucial for predicting the performance of an algorithm when dealing with large datasets.
For example, if an algorithm has a time complexity of O(n), it means that the execution time of the algorithm grows linearly with the size of the input. If the input size doubles, the execution time will also roughly double. In contrast, an algorithm with a time complexity of O(n^2) will have its execution time increase quadratically with the input size, making it much less efficient for large inputs.
What are some common time complexities and their Big O notations?
There are several common time complexities and their corresponding Big O notations that are frequently encountered in algorithm analysis:
- O(1) - Constant Time Complexity: The execution time of the algorithm does not change with the size of the input. An example is accessing an element in an array by its index.
- O(log n) - Logarithmic Time Complexity: The execution time grows logarithmically with the size of the input. This is typical of algorithms that divide the problem size by a constant factor in each step, such as binary search.
- O(n) - Linear Time Complexity: The execution time grows linearly with the size of the input. An example is traversing a list once.
- O(n log n) - Linearithmic Time Complexity: The execution time grows as the product of the input size and its logarithm. This is common in efficient sorting algorithms like Merge Sort and Quick Sort.
- O(n^2) - Quadratic Time Complexity: The execution time grows quadratically with the size of the input. This is typical of algorithms with nested loops, such as simple sorting algorithms like Bubble Sort.
- O(2^n) - Exponential Time Complexity: The execution time grows exponentially with the size of the input. This is seen in algorithms that generate all possible solutions, such as certain brute-force approaches.
- O(n!) - Factorial Time Complexity: The execution time grows factorially with the size of the input. This is seen in algorithms that generate all permutations, such as the Traveling Salesman Problem solved by brute force.
How can Big O notation help in comparing the efficiency of different algorithms?
Big O notation is a powerful tool for comparing the efficiency of different algorithms because it provides a standardized way to express the growth rate of an algorithm's time or space requirements. Here's how it helps in comparing algorithms:
- Scalability Analysis: Big O notation allows developers to understand how an algorithm's performance scales with increasing input sizes. By comparing the Big O notations of different algorithms, one can determine which algorithm will perform better as the input size grows.
- Worst-Case Scenario: Big O notation focuses on the worst-case scenario, which is crucial for ensuring that an algorithm can handle the most challenging inputs. This helps in making informed decisions about which algorithm to use in critical applications.
- Simplified Comparison: Big O notation simplifies the comparison by ignoring constants and lower-order terms, focusing only on the dominant factor that affects the growth rate. This makes it easier to compare algorithms without getting bogged down in minor details.
- Trade-off Analysis: When multiple algorithms can solve a problem, Big O notation helps in analyzing trade-offs between time and space complexity. For example, an algorithm with O(n log n) time complexity might be preferred over one with O(n^2) time complexity, even if the latter has a lower space complexity.
- Optimization Guidance: Understanding Big O notation can guide developers in optimizing algorithms. By identifying the dominant factor in an algorithm's time complexity, developers can focus their optimization efforts on reducing that factor.
In what practical scenarios is understanding time complexity analysis crucial for software development?
Understanding time complexity analysis is crucial in several practical scenarios in software development:
- Large-Scale Data Processing: When dealing with big data, understanding time complexity is essential for choosing algorithms that can efficiently process large datasets. For example, in data analytics, algorithms with O(n log n) time complexity, such as sorting algorithms, are preferred over those with O(n^2) complexity.
- Real-Time Systems: In real-time systems, such as embedded systems or control systems, where timely responses are critical, understanding time complexity helps in ensuring that algorithms meet strict timing constraints. Algorithms with predictable and low time complexity are preferred.
- Database Query Optimization: In database management, understanding the time complexity of query operations can significantly impact the performance of database applications. For instance, choosing the right indexing strategy can reduce the time complexity of search operations from O(n) to O(log n).
- Algorithm Design and Optimization: When designing new algorithms or optimizing existing ones, time complexity analysis is crucial for making informed decisions about trade-offs between different approaches. It helps in identifying bottlenecks and improving the overall efficiency of the software.
- Resource-Constrained Environments: In environments with limited computational resources, such as mobile devices or IoT devices, understanding time complexity helps in selecting algorithms that are efficient in terms of both time and space. This ensures that the software runs smoothly within the constraints of the hardware.
- Scalability Planning: For applications that are expected to scale, understanding time complexity is essential for planning and ensuring that the software can handle increased loads without significant performance degradation. This is particularly important in cloud computing and web services.
By understanding and applying time complexity analysis, developers can make more informed decisions, leading to more efficient and scalable software solutions.
The above is the detailed content of Explain the concepts of Big O notation and time complexity analysis.. For more information, please follow other related articles on the PHP Chinese website!

Hot AI Tools

Undresser.AI Undress
AI-powered app for creating realistic nude photos

AI Clothes Remover
Online AI tool for removing clothes from photos.

Undress AI Tool
Undress images for free

Clothoff.io
AI clothes remover

Video Face Swap
Swap faces in any video effortlessly with our completely free AI face swap tool!

Hot Article

Hot Tools

Notepad++7.3.1
Easy-to-use and free code editor

SublimeText3 Chinese version
Chinese version, very easy to use

Zend Studio 13.0.1
Powerful PHP integrated development environment

Dreamweaver CS6
Visual web development tools

SublimeText3 Mac version
God-level code editing software (SublimeText3)

Hot Topics










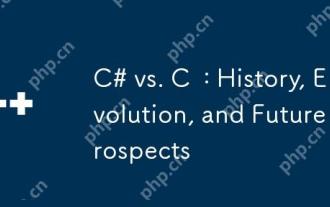
The history and evolution of C# and C are unique, and the future prospects are also different. 1.C was invented by BjarneStroustrup in 1983 to introduce object-oriented programming into the C language. Its evolution process includes multiple standardizations, such as C 11 introducing auto keywords and lambda expressions, C 20 introducing concepts and coroutines, and will focus on performance and system-level programming in the future. 2.C# was released by Microsoft in 2000. Combining the advantages of C and Java, its evolution focuses on simplicity and productivity. For example, C#2.0 introduced generics and C#5.0 introduced asynchronous programming, which will focus on developers' productivity and cloud computing in the future.
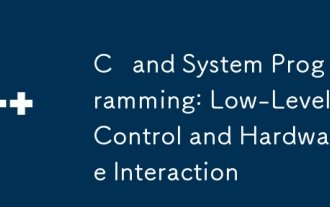
C is suitable for system programming and hardware interaction because it provides control capabilities close to hardware and powerful features of object-oriented programming. 1)C Through low-level features such as pointer, memory management and bit operation, efficient system-level operation can be achieved. 2) Hardware interaction is implemented through device drivers, and C can write these drivers to handle communication with hardware devices.
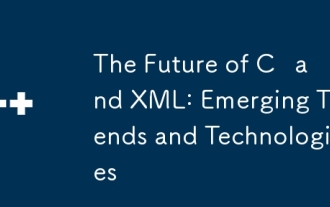
The future development trends of C and XML are: 1) C will introduce new features such as modules, concepts and coroutines through the C 20 and C 23 standards to improve programming efficiency and security; 2) XML will continue to occupy an important position in data exchange and configuration files, but will face the challenges of JSON and YAML, and will develop in a more concise and easy-to-parse direction, such as the improvements of XMLSchema1.1 and XPath3.1.
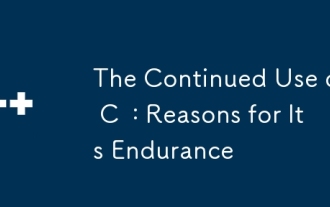
C Reasons for continuous use include its high performance, wide application and evolving characteristics. 1) High-efficiency performance: C performs excellently in system programming and high-performance computing by directly manipulating memory and hardware. 2) Widely used: shine in the fields of game development, embedded systems, etc. 3) Continuous evolution: Since its release in 1983, C has continued to add new features to maintain its competitiveness.
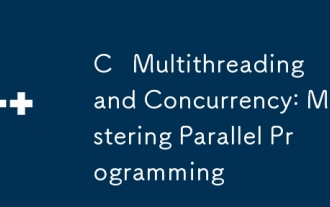
C The core concepts of multithreading and concurrent programming include thread creation and management, synchronization and mutual exclusion, conditional variables, thread pooling, asynchronous programming, common errors and debugging techniques, and performance optimization and best practices. 1) Create threads using the std::thread class. The example shows how to create and wait for the thread to complete. 2) Synchronize and mutual exclusion to use std::mutex and std::lock_guard to protect shared resources and avoid data competition. 3) Condition variables realize communication and synchronization between threads through std::condition_variable. 4) The thread pool example shows how to use the ThreadPool class to process tasks in parallel to improve efficiency. 5) Asynchronous programming uses std::as
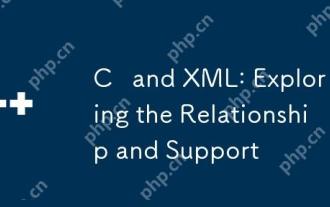
C interacts with XML through third-party libraries (such as TinyXML, Pugixml, Xerces-C). 1) Use the library to parse XML files and convert them into C-processable data structures. 2) When generating XML, convert the C data structure to XML format. 3) In practical applications, XML is often used for configuration files and data exchange to improve development efficiency.
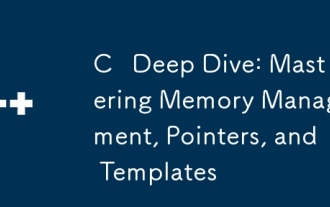
C's memory management, pointers and templates are core features. 1. Memory management manually allocates and releases memory through new and deletes, and pay attention to the difference between heap and stack. 2. Pointers allow direct operation of memory addresses, and use them with caution. Smart pointers can simplify management. 3. Template implements generic programming, improves code reusability and flexibility, and needs to understand type derivation and specialization.
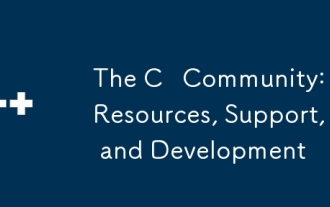
C Learners and developers can get resources and support from StackOverflow, Reddit's r/cpp community, Coursera and edX courses, open source projects on GitHub, professional consulting services, and CppCon. 1. StackOverflow provides answers to technical questions; 2. Reddit's r/cpp community shares the latest news; 3. Coursera and edX provide formal C courses; 4. Open source projects on GitHub such as LLVM and Boost improve skills; 5. Professional consulting services such as JetBrains and Perforce provide technical support; 6. CppCon and other conferences help careers
