Building Custom Tools for AI Agents Using smolagents
LLMs are revolutionizing various fields, moving beyond web-based chatbots to integrate into enterprises and government. A significant advancement is the creation of custom tools for AI agents using smolagents
, expanding their capabilities. smolagents
empowers AI agents to utilize tools, perform actions within defined environments, and even interact with other agents.
This approach enhances LLM-powered AI systems' autonomy, improving their reliability for complete end-to-end task execution.
Learning Objectives
- Understand AI agents, their distinction from traditional LLMs, and their role in modern AI applications with custom tools.
- Explore why AI agents require custom tools for real-time data access, action execution, and improved decision-making.
- Gain practical experience in integrating and deploying AI agents using
smolagents
for real-world scenarios. - Learn to create and integrate custom tools for enhanced AI agent functionality using
smolagents
. - Master hosting and interacting with an AI agent utilizing your custom tools, creating a more interactive and intelligent chatbot experience.
*This article is part of the***Data Science Blogathon.
Table of contents
- Prerequisites
- Understanding Agents in Generative AI
- Agent Workflow
- Components of an AI Agent
- The Necessity of Tools
- The
smolagents
Library - Our Codebase
- Building Our First Tool
- Final Step: Deployment
- Summary
- Frequently Asked Questions
Prerequisites
This tutorial targets intermediate developers and data professionals familiar with basic LLMs. The following is assumed:
- Intermediate Python programming skills.
- Basic LLM usage in code.
- Familiarity with the GenAI ecosystem.
- Fundamental understanding of the Hugging Face platform and the
transformers
library in Python.
Further recommended background for optimal learning:
- Experience with LLM libraries like LangChain or Ollama.
- Basic Machine Learning theory knowledge.
- API usage and problem-solving with API responses.
Understanding Agents in Generative AI
Consider ChatGPT: it answers questions, writes code, and more. This capability extends to task completion—you provide a request, and it executes the entire task.
For example, an LLM can search the web and reason; combining these, it can create a travel itinerary. You might ask: "Plan a Himachal Pradesh vacation from April 1st to 7th, focusing on snow, skiing, ropeways, and green landscapes. Find the cheapest flights from Kolkata."
The AI would then compare flight costs, suggest locations, and find hotels, demonstrating an agentic approach in AI.
Agent Workflow
An agent uses an LLM that interacts with the external world solely through text.
The agent receives input as text, reasons using language, and outputs text. Tools are crucial here, providing values the agent uses to generate its textual response. Actions can range from market transactions to image generation.
The workflow is: Understand -> Reason -> Interact, or more broadly: Thought -> Action -> Observation.
Components of an AI Agent
An AI agent comprises:
- The agent's "brain" (an LLM like llama3, phi4, or GPT4).
- External tools the agent can invoke (APIs, other agents, calculators, etc.).
smolagents
lets you create any Python function with an LLM tuned for function calling. Our example will include tools for dog facts, timezone retrieval, and image generation, using a Qwen LLM.
The Necessity of Tools
LLMs are no longer just text-completion tools. They're components in larger systems, often needing input from non-Generative AI parts.
Tools bridge the gap between GenAI and other system components. LLMs have limitations:
- Knowledge cut-off dates.
- Hallucinations.
- Unpredictable refusal to answer.
- Suboptimal web search choices.
Deterministic tools address these issues.
The smolagents
Library
smolagents
(Hugging Face) is a framework for building agents. Unlike some libraries that output JSON, smolagents
directly outputs Python code, improving efficiency.
Our Codebase
The GitHub repository contains:
-
Gradio_UI.py
: Gradio UI code for user interaction. -
agent.json
: Agent configuration. -
requirements.txt
: Project dependencies. -
prompts.yaml
: Example prompts and responses (using Jinja templating). -
app.py
: The core application logic.
Building Our First Tool
We'll use a dog facts API (https://www.php.cn/link/0feaf58e2a12936c84c2510541b6e75a). To make a Python function usable by the AI agent:
- Use the
@tool
decorator. - Write a clear docstring.
- Add type annotations.
- Ensure a clear return value.
- Include ample comments.
@tool def get_amazing_dog_fact()-> str: """Fetches a random dog fact from a public API.""" # ... (API call and error handling) ...
A timezone tool:
@tool def get_current_time_in_timezone(timezone: str) -> str: """Gets the current time in a specified timezone.""" # ... (timezone handling) ...
An image generation tool can also be integrated:
image_generation_tool = load_tool("agents-course/text-to-image", trust_remote_code=True)
The Qwen2.5-Coder-32B-Instruct model is used (requires application for access):
model = HfApiModel( max_tokens=2096, temperature=0.5, model_id='Qwen/Qwen2.5-Coder-32B-Instruct', # ... )
Prompts are loaded from prompts.yaml
. The agent is created:
agent = CodeAgent( model=model, tools=[get_amazing_dog_fact, get_current_time_in_timezone, image_generation_tool], # ... )
The tools
argument lists the available functions.
Final Step: Deployment
The agent can be deployed on Hugging Face Spaces.
Summary
AI agents enhance LLM capabilities through tool integration, increasing autonomy and enabling complex task completion. smolagents
simplifies agent creation, and custom tools extend functionality beyond standard LLMs. Deployment on platforms like Hugging Face Spaces facilitates easy sharing and interaction.
Frequently Asked Questions
Q1. What is an AI agent? An AI agent is an LLM-powered system interacting with tools to perform tasks.
Q2. Why are custom tools needed? They enable real-time data access, command execution, and actions beyond LLM capabilities.
Q3. What is smolagents
? A Hugging Face framework for creating AI agents using custom tools.
Q4. How to create custom tools? Define functions, decorate with @tool
, and integrate into the agent.
Q5. Where to deploy? Platforms like Hugging Face Spaces.
(Note: Images are assumed to be included as in the original input.)
The above is the detailed content of Building Custom Tools for AI Agents Using smolagents. For more information, please follow other related articles on the PHP Chinese website!

Hot AI Tools

Undresser.AI Undress
AI-powered app for creating realistic nude photos

AI Clothes Remover
Online AI tool for removing clothes from photos.

Undress AI Tool
Undress images for free

Clothoff.io
AI clothes remover

Video Face Swap
Swap faces in any video effortlessly with our completely free AI face swap tool!

Hot Article

Hot Tools

Notepad++7.3.1
Easy-to-use and free code editor

SublimeText3 Chinese version
Chinese version, very easy to use

Zend Studio 13.0.1
Powerful PHP integrated development environment

Dreamweaver CS6
Visual web development tools

SublimeText3 Mac version
God-level code editing software (SublimeText3)

Hot Topics










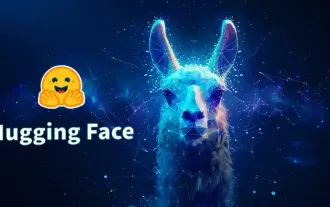
Meta's Llama 3.2: A Leap Forward in Multimodal and Mobile AI Meta recently unveiled Llama 3.2, a significant advancement in AI featuring powerful vision capabilities and lightweight text models optimized for mobile devices. Building on the success o
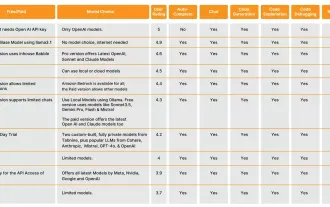
Hey there, Coding ninja! What coding-related tasks do you have planned for the day? Before you dive further into this blog, I want you to think about all your coding-related woes—better list those down. Done? – Let’
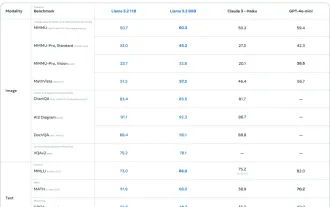
This week's AI landscape: A whirlwind of advancements, ethical considerations, and regulatory debates. Major players like OpenAI, Google, Meta, and Microsoft have unleashed a torrent of updates, from groundbreaking new models to crucial shifts in le
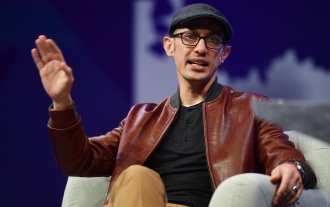
Shopify CEO Tobi Lütke's recent memo boldly declares AI proficiency a fundamental expectation for every employee, marking a significant cultural shift within the company. This isn't a fleeting trend; it's a new operational paradigm integrated into p
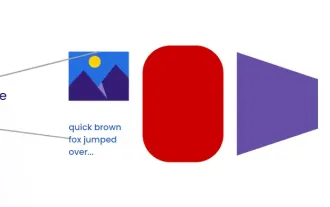
Introduction Imagine walking through an art gallery, surrounded by vivid paintings and sculptures. Now, what if you could ask each piece a question and get a meaningful answer? You might ask, “What story are you telling?
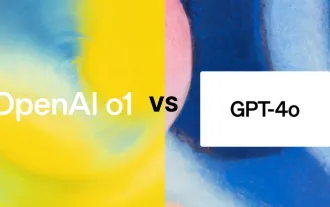
Introduction OpenAI has released its new model based on the much-anticipated “strawberry” architecture. This innovative model, known as o1, enhances reasoning capabilities, allowing it to think through problems mor
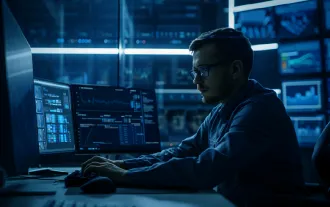
SQL's ALTER TABLE Statement: Dynamically Adding Columns to Your Database In data management, SQL's adaptability is crucial. Need to adjust your database structure on the fly? The ALTER TABLE statement is your solution. This guide details adding colu
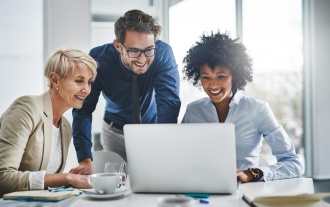
For those of you who might be new to my column, I broadly explore the latest advances in AI across the board, including topics such as embodied AI, AI reasoning, high-tech breakthroughs in AI, prompt engineering, training of AI, fielding of AI, AI re
