I Have Built A News Agent on Hugging Face
Unlock the Power of AI Agents: A Deep Dive into the Hugging Face Course
This article summarizes key learnings from the Hugging Face AI Agents course, covering the theoretical underpinnings, design principles, and practical implementation of AI agents. The course emphasizes building a strong foundation in AI agent fundamentals. This summary explores agent design, the role of Large Language Models (LLMs), and practical applications using the SmolAgent framework.
Table of Contents:
- Understanding AI Agents
- AI Agents and Tool Utilization
- LLMs: A Technical Overview
- Transformer Models: How They Work
- LLM Token Prediction
- Autoregressive Nature of LLMs
- The Attention Mechanism in Transformers
- Chat Templates for AI Agents
- The Importance of Chat Templates
- Defining AI Tools
- The AI Agent Workflow: Think-Act-Observe
- The ReAct Approach
- Building Agents with SmolAgents
- Conclusion
What are AI Agents?
An AI agent is an autonomous system capable of analyzing its environment, strategizing, and taking actions to achieve defined goals. Think of it as a virtual assistant capable of performing everyday tasks. The agent's internal workings involve reasoning and planning, breaking down complex tasks into smaller, manageable steps.
Technically, an agent comprises two key components: a cognitive core (the decision-making AI model, often an LLM) and an operational interface (the tools and resources used to execute actions). The effectiveness of an AI agent hinges on the seamless integration of these two components.
AI Agents and Tool Usage
AI agents leverage specialized tools to interact with their environment and achieve objectives. These tools can range from simple functions to complex APIs. Effective tool design is crucial; tools must be tailored to specific tasks, and a single action might involve multiple tools working in concert.
LLMs: The Brain of the Agent
Large Language Models (LLMs) are the core of many AI agents, processing text input and generating text output. Most modern LLMs utilize the Transformer architecture, employing an "attention" mechanism to focus on the most relevant parts of the input text. Decoder-based Transformers are particularly well-suited for generative tasks.
LLM Token Prediction and Autoregression
LLMs predict the next token in a sequence based on preceding tokens. This autoregressive process continues until a special End-of-Sequence (EOS) token is generated. Different decoding strategies (e.g., greedy search, beam search) exist to optimize this prediction process.
The Transformer Architecture: Attention is Key
The attention mechanism in Transformer models allows the model to focus on the most relevant parts of the input when generating output, significantly improving performance. Context length—the maximum number of tokens a model can process at once—is a critical factor influencing an LLM's capabilities.
Chat Templates and Their Importance
Chat templates structure conversations between users and AI agents, ensuring proper interpretation and processing of prompts by the LLM. They standardize formatting, incorporate special tokens, and manage context across multiple turns in a conversation. System messages within these templates provide instructions and guidelines for the agent's behavior.
AI Tools: Expanding Agent Capabilities
AI tools are functions that extend an LLM's capabilities, allowing it to interact with the real world. Examples include web search, image generation, data retrieval, and API interaction. Well-designed tools enhance an LLM's ability to perform complex tasks.
The AI Agent Workflow: Think-Act-Observe
The core workflow of an AI agent is a cycle of thinking, acting, and observing. The agent thinks about the next step, takes action using appropriate tools, and observes the results to inform subsequent actions. This iterative process ensures efficient and logical task completion.
The ReAct Approach
The ReAct approach emphasizes step-by-step reasoning, prompting the model to break down problems into smaller, manageable steps, leading to more structured and accurate solutions.
SmolAgents: Building Agents with Ease
The SmolAgents framework simplifies AI agent development. Different agent types (JSON Agent, Code Agent, Function-calling Agent) offer varying levels of control and flexibility. The course demonstrates building agents using this framework, showcasing its efficiency and ease of use.
Conclusion
The Hugging Face AI Agents course provides a solid foundation for understanding and building AI agents. This summary highlights key concepts and practical applications, emphasizing the importance of LLMs, tools, and structured workflows in creating effective AI agents. Future articles will delve deeper into frameworks like LangChain and LangGraph.
The above is the detailed content of I Have Built A News Agent on Hugging Face. For more information, please follow other related articles on the PHP Chinese website!

Hot AI Tools

Undresser.AI Undress
AI-powered app for creating realistic nude photos

AI Clothes Remover
Online AI tool for removing clothes from photos.

Undress AI Tool
Undress images for free

Clothoff.io
AI clothes remover

Video Face Swap
Swap faces in any video effortlessly with our completely free AI face swap tool!

Hot Article

Hot Tools

Notepad++7.3.1
Easy-to-use and free code editor

SublimeText3 Chinese version
Chinese version, very easy to use

Zend Studio 13.0.1
Powerful PHP integrated development environment

Dreamweaver CS6
Visual web development tools

SublimeText3 Mac version
God-level code editing software (SublimeText3)

Hot Topics
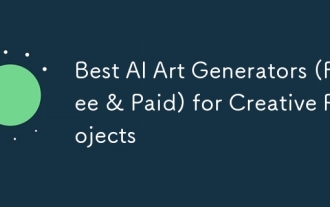
The article reviews top AI art generators, discussing their features, suitability for creative projects, and value. It highlights Midjourney as the best value for professionals and recommends DALL-E 2 for high-quality, customizable art.
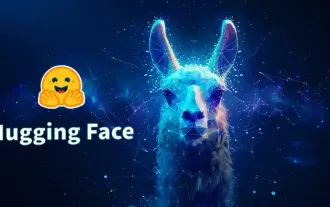
Meta's Llama 3.2: A Leap Forward in Multimodal and Mobile AI Meta recently unveiled Llama 3.2, a significant advancement in AI featuring powerful vision capabilities and lightweight text models optimized for mobile devices. Building on the success o
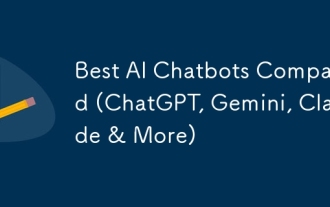
The article compares top AI chatbots like ChatGPT, Gemini, and Claude, focusing on their unique features, customization options, and performance in natural language processing and reliability.
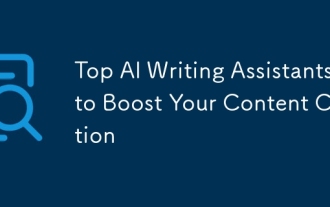
The article discusses top AI writing assistants like Grammarly, Jasper, Copy.ai, Writesonic, and Rytr, focusing on their unique features for content creation. It argues that Jasper excels in SEO optimization, while AI tools help maintain tone consist
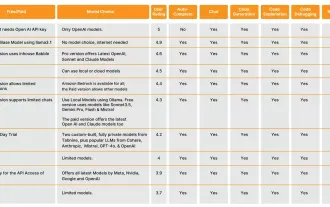
Hey there, Coding ninja! What coding-related tasks do you have planned for the day? Before you dive further into this blog, I want you to think about all your coding-related woes—better list those down. Done? – Let’
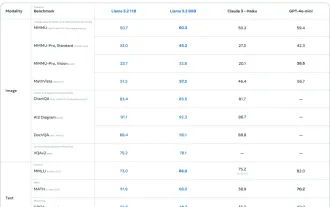
This week's AI landscape: A whirlwind of advancements, ethical considerations, and regulatory debates. Major players like OpenAI, Google, Meta, and Microsoft have unleashed a torrent of updates, from groundbreaking new models to crucial shifts in le
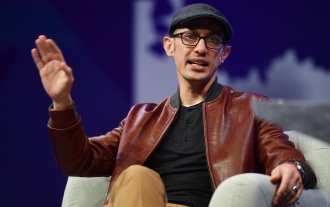
Shopify CEO Tobi Lütke's recent memo boldly declares AI proficiency a fundamental expectation for every employee, marking a significant cultural shift within the company. This isn't a fleeting trend; it's a new operational paradigm integrated into p
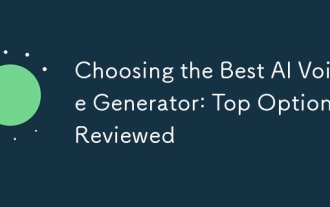
The article reviews top AI voice generators like Google Cloud, Amazon Polly, Microsoft Azure, IBM Watson, and Descript, focusing on their features, voice quality, and suitability for different needs.
