How to Use OpenAI's Responses API & Agent SDK? - Analytics Vidhya
OpenAI has been a leading solutions provider in the GenAI space. From the legendary ChatGPT to Sora, it is a go-to platform for all the working professionals out there. With Qwen and Claude gaining popularity among developers, OpenAI is back again with its latest updates, empowering developers to create more reliable and capable AI agents. The major highlights from the list include the Responses API and Agents SDK. In this blog, we will explore the Responses API and Agents SDK, understand how to access them, and learn how to use them to build real-world applications!
Table of contents
- What is the Responses API?
- How to use Responses API?
- How is the Responses API Different from the Completions API?
- Introducing the Agents SDK
- Build a Multi-agentic System using Agent SDK
- Why Do Developers Need Responses API & Agents SDK?
- Conclusion
- Frequently Asked Questions
What is the Responses API?
The Responses API is OpenAI’s newest API designed for simplifying the process of building AI-based applications. It combines the simplicity of the Chat Completions API with the powerful tool-use capabilities of the Assistants API. This means developers can now create agents that leverage multiple tools and handle complex, multi-step tasks more efficiently. This API reduced the reliance on complex prompt engineering and external integrations.
Our new API primitive: the Responses API. Combining the simplicity of Chat Completions with the tool-use of Assistants, this new foundation provides more flexibility in building agents. Web search, file search, or computer use are a couple lines of code!https://t.co/s5Zsy4Wvqy pic.twitter.com/ParhjhSJgV
— OpenAI Developers (@OpenAIDevs) March 11, 2025
Key Features of the Responses API
- Built-in tools like web search, file search, and computer use, allowing agents to interact with real-world data.
- Unified design that simplifies polymorphism and improves usability.
- Better observability, helping developers track agent behavior and optimize workflows.
- No additional costs, as it is charged based on token usage at OpenAI’s standard pricing.
With these tools, Responses API is a game changer towards building AI agents. Infact, going forward, Responses API will support all of OpenAI’s new and upcoming models. Let’s see how we can use it to build applications.
How to use Responses API?
To try Responses API:
- Install openai (if not already installed) and use OpenAI.
- Ensure you have the latest OpenAI library(pip install openai – -upgrade).
- Import OpenAI and Set Up the Client.
Once set up, you can request the Responses API. While basic API calls are common, its built-in capabilities make it powerful. Let’s explore three key features:
- File Search: Retrieve insights from documents.
- Web Search: Get real-time, cited information.
- Computer Use: Automate system interactions.
Now, let’s see them in action!
1. File Search
It enables models to retrieve information in a knowledge base of previously uploaded files through semantic and keyword search. Currently it doesn’t support csv files, you can check the list of supported file types here.
Note: Before using the file search, make sure to store your files in a vector database
Task: Names of people with domain as Data Science. (I used the following File.)
Code:
response = client.responses.create( model="gpt-4o-mini", input="Names of people with domain as Data Science", tools=[{ "type": "file_search", "vector_store_ids": [vector_store_id], "filters": { "type": "eq", "key": "Domain", "value": "Data Science" } }] ) print(response.output_text)
Output:
The person with the domain of Data Science is Alice Johnson [0].<br>[0] names_and_domains.pdf
2. Web Search
This feature allows models to search the web for the latest information before generating a response, ensuring that the data remains up to date. The model can choose to search the web or not based on the content of the input prompt.
Task : What are the best cafes in Vijay nagar?
Code:
response = client.responses.create( model="gpt-4o", tools=[{ "type": "web_search_preview", "user_location": { "type": "approximate", "country": "IN", "city": "Indore", "region": "Madhya Pradesh", } }], input="What are the best cafe in Vijay nagar?", ) print(response.output_text)
Output:
3. Computer Use
It is a practical application of Computer-using Agent(CUA) Model, which combines the vision capabilities of GPT-4o with advanced reasoning to simulate controlling computer interfaces and perform tasks.
Task: Check the latest blog on Analytics Vidhya website.
Code:
response = client.responses.create( model="computer-use-preview", tools=[{ "type": "computer_use_preview", "display_width": 1024, "display_height": 768, "environment": "browser" # other possible values: "mac", "windows", "ubuntu" }], input=[ { "role": "user", "content": "Check the latest blog on Analytics Vidhya website." } ], truncation="auto" ) print(response.output)
Output:
ResponseComputerToolCall(id='cu_67d147af346c8192b78719dd0e22856964fbb87c6a42e96', <br>action=ActionScreenshot(type='screenshot'), <br>call_id='call_a0w16G1BNEk09aYIV25vdkxY', pending_safety_checks=[], <br>status='completed', type='computer_call')
How is the Responses API Different from the Completions API?
Now that we have seen how the Responses API works, let’s see how different it is from the pre-existing Completions API.
Responses API vs Completions API: Execution
API | Responses API | Completions API |
Code |
from openai import OpenAI client = OpenAI() response = client.responses.create( model="gpt-4o", inputs=[ { "role": "user", "content": "Write a one-sentence bedtime story about a unicorn." } ] ) print(response.output_text) Copy after login |
from openai import OpenAI client = OpenAI() completion = client.chat.completions.create( model="gpt-4o", messages=[ { "role": "user", "content": "Write a one-sentence bedtime story about a unicorn." } ] ) print(completion.choices[0].message.content) Copy after login |
Output |
|
|
Responses API vs Completions API: Features
Here is a simplified breakdown of the various features of Chat Complerions APi and Responses API:
Capabilities | Responses API | Chat Completions API |
Text generation | ✅ | ✅ |
Audio | Coming soon | ✅ |
Vision | ✅ | ✅ |
Web search | ✅ | ✅ |
File search | ✅ | ❌ |
Computer use | ✅ | ❌ |
Code interpreter | Coming soon | ❌ |
Response Handling | Returns a single structured output | Returns choices array |
Conversation State | previous_response_id for continuity | Must be manually managed |
Storage Behavior | Stored by default (store: false to disable) | Stored by default |
Roadmap: What Will Continue, What Will Deprecate?
With Responses API going live, the burning question now is, would it affect the existing Chat Completions and Assistant API? Yes it would. Let’s look at how:
- Chat Completions API: OpenAI will continue updating it with new models, but only when the capabilities don’t require built-in tools.
- Web Search & File Search Tools: These will become more refined and powerful in the Responses API.
- Assistants API: The Responses API incorporates its best features while improving performance. OpenAI has announced that full feature parity is coming soon, and the Assistants API will be deprecated by mid-2026.
Introducing the Agents SDK
Building AI agents is not just about having a powerful API—it requires efficient orchestration. This is where OpenAI’s Agents SDK comes into play. The Agents SDK is an open-source toolkit that simplifies agent workflows. This agent building framework integrates seamlessly with the Responses API and Chat Completions API. Additionally, it is also compatible with models from various providers, provided they offer an API endpoint styled like Chat Completions.
Some of the key features of Agents SDK are:
- It allows developers to configure AI agents with built-in tools.
- It enables multi-agent orchestration, allowing seamless coordination of different agents as needed.
- It allows us to track the conversation & the flow of information between our agents.
- It allows an easier way to apply guardrails for safety and compliance.
- It ensures that developers can monitor and optimize agent performance with built-in observability tools.
Agent SDK isn’t a “new addition” to OpenAI’s jewels. It is an improved version of “Swarm”, the experimental SDK that OpenAI had released last year. While “Swarm” was just released for educational purposes, it became popular among developers and was adopted by several enterprises too. To cater to more enterprises and help them build production-grade agents seamlessly, Agents SDK has been released. Now that we know what Agents SDK has to offer, let’s see how we can use this framework to build our agentic system.
Also Read: Top 10 Generative AI Coding Extensions in VS Code
Build a Multi-agentic System using Agent SDK
We will build a multi-agent system that helps users with car recommendations and resale price estimation by leveraging LLM-powered agents and web search tools to provide accurate and up-to-date insights.
Step 1: Building a Simple AI Agent
We begin by creating a Car Advisor Agent that helps users choose a suitable car type based on their needs.
Code:
car_advisor = Agent( name="Car advisor", instructions= "You are an expert in advising suitable car type like sedan, hatchback etc to people based on their requirements.", model="gpt-4o", ) prompt = "I am looking for a car that I enjoy driving and comforatbly take 4 people. I plane to travel to hills. What type of car should I buy?" async def main(): result = await Runner.run(car_advisor, prompt) print(result.final_output) # Run the function in Jupyter await main()
Output:
Step 2: Build the Multi-Agent System
With the basic agent in place, we now create a multi-agent system incorporating different AI agents specialized in their respective domains. Here’s how it works:
Agents in the Multi-Agent System
- Car Sell Estimate Agent: It provides a resale price estimate based on car details.
- Car Model Advisor Agent: It suggests suitable car models based on budget and location.
- Triage Agent: It directs the query to the appropriate agent.
We will provide two different prompts to the agents and observe their outputs.
Code:
car_sell_estimate = Agent( name="Car sell estimate", instructions= "You are an expert in suggesting a suitable price of reselling a car based on its make, model, year of purchase, and condition.", handoff_description= "Car reselling price estimate expert", model="gpt-4o", tools=[WebSearchTool()] ) car_model_advisor = Agent( name="Car model advisor", instructions= "You are an expert in advising suitable car model to people based on their budget and location.", handoff_description= "Car model recommendation expert", model="gpt-4o", tools=[WebSearchTool()] ) triage_agent = Agent( name = "Triage Agent", instructions="You determine the appropriate agent for the task.", model = "gpt-4o", handoffs=[car_sell_estimate, car_model_advisor] ) Prompt 1: prompt = "I want to sell my Ecosport car in New Delhi. It is 3 years old and in good condition. 50000Km. What price should I expect?" async def main(): result = await Runner.run(triage_agent, prompt) print(result.final_output) # Run the function in Jupyter await main()
Output 1:
Prompt 2:
prompt = "I want to buy a high acceleration car, comfortable for 4 people for 20 lakhs in New Delhi. Which car should I buy?" async def main(): result = await Runner.run(triage_agent, prompt) print(result.final_output) # Run the function in Jupyter await main()
Output 2:
We got the car options as per our requirements! The implementation was simple and quick. You can use this agentic framework to build agents for travel support, financial planning, medical assistance, personalized shopping, automated research, and much more.
Agent’s SDK: A New Agentic Framework in Town?
OpenAI’s Agents SDK represents its strategic push toward providing a dedicated framework for AI agent development. The framework includes crew-like features through its triage agent, mimicking Crew AI’s features. Similarly, its handoff mechanisms closely resemble those of AutoGen, allowing efficient delegation of tasks among multiple agents.
Furthermore, LangChain’s strength in modular agent orchestration is mirrored in the way the Agents SDK provides structured workflows, ensuring smooth execution and adaptability. While Agents SDK offers nothing more than what the existing frameworks already do, it soon will give them a tough competition.
Also Read: Claude 3.7 Sonnet: The Best Coding Model Yet?
Why Do Developers Need Responses API & Agents SDK?
Responses API and Agents SDK provide developers with the tools & platform to build AI-driven applications. By reducing the reliance on manual prompt engineering and extensive custom logic, these tools allow developers to focus on creating intelligent workflows with minimal friction.
- Easy Integration: Developers no longer need to juggle multiple APIs for different tools; the Responses API consolidates web search, file search, and computer use into a single interface.
- Better Observability: With built-in monitoring and debugging tools, developers can optimize agent performance more easily.
- Scalability: The Agents SDK provides a structured approach to handling multi-agent workflows, enabling more robust automation.
- Improved Development Cycles: By eliminating the need for extensive prompt iteration and external tool integration, developers can prototype and deploy agent-based applications at a much faster pace.
Here’s a video to learn more about OpenAI’s Responses API and Agents SDK.
Conclusion
The introduction of OpenAI’s Responses API and Agents SDK is a game-changer for AI-driven automation. By leveraging these tools, we successfully built a multi-agent system very quickly with just a few lines of code. This implementation can be further expanded to include additional tools, integrations, and agent capabilities, paving the way for more intelligent and autonomous AI applications in various industries.
These tools are surely going to help developers and enterprises reduce development complexity, and create smarter, more scalable automation solutions. Whether it’s for customer support, research, business automation, or industry-specific AI applications, the Responses API and Agents SDK offer a powerful framework to build next-generation AI-powered systems with ease.
Frequently Asked Questions
Q1. What is OpenAI’s Responses API?A. The Responses API is OpenAI’s latest AI framework that simplifies agent development by integrating built-in tools like web search, file search, and computer use.
Q2. How is the Responses API different from the Completions API?A. Unlike the Completions API, the Responses API supports multi-tool integration, structured outputs, and built-in conversation state management.
Q3. What is OpenAI’s Agents SDK?A. The Agents SDK is an open-source framework that enables developers to build and orchestrate multi-agent systems with AI-powered automation.
Q4. How does the Agents SDK improve AI development?A. It allows seamless agent coordination, enhanced observability, built-in guardrails, and improved performance tracking.
Q5. Can the Responses API and Agents SDK be used together?A. Yes! The Agents SDK integrates with the Responses API to create powerful AI-driven applications.
Q6. Is OpenAI’s Agents SDK compatible with other AI models?A. Yes, it can work with third-party models that support Chat Completions API-style integrations.
Q7. What industries can benefit from multi-agent AI systems?A. Industries like automotive, finance, healthcare, customer support, and research can use AI-driven agents to optimize operations and decision-making.
The above is the detailed content of How to Use OpenAI's Responses API & Agent SDK? - Analytics Vidhya. For more information, please follow other related articles on the PHP Chinese website!

Hot AI Tools

Undresser.AI Undress
AI-powered app for creating realistic nude photos

AI Clothes Remover
Online AI tool for removing clothes from photos.

Undress AI Tool
Undress images for free

Clothoff.io
AI clothes remover

Video Face Swap
Swap faces in any video effortlessly with our completely free AI face swap tool!

Hot Article

Hot Tools

Notepad++7.3.1
Easy-to-use and free code editor

SublimeText3 Chinese version
Chinese version, very easy to use

Zend Studio 13.0.1
Powerful PHP integrated development environment

Dreamweaver CS6
Visual web development tools

SublimeText3 Mac version
God-level code editing software (SublimeText3)

Hot Topics










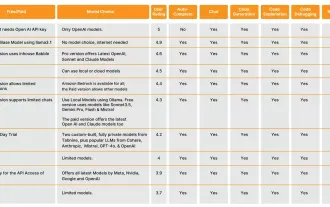
Hey there, Coding ninja! What coding-related tasks do you have planned for the day? Before you dive further into this blog, I want you to think about all your coding-related woes—better list those down. Done? – Let’
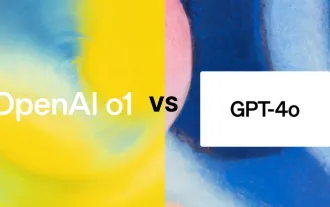
Introduction OpenAI has released its new model based on the much-anticipated “strawberry” architecture. This innovative model, known as o1, enhances reasoning capabilities, allowing it to think through problems mor
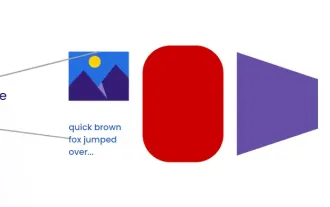
Introduction Imagine walking through an art gallery, surrounded by vivid paintings and sculptures. Now, what if you could ask each piece a question and get a meaningful answer? You might ask, “What story are you telling?
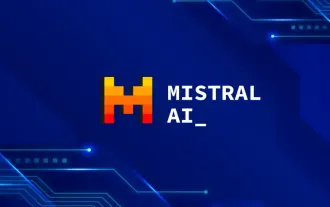
Introduction Mistral has released its very first multimodal model, namely the Pixtral-12B-2409. This model is built upon Mistral’s 12 Billion parameter, Nemo 12B. What sets this model apart? It can now take both images and tex
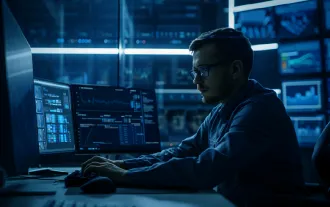
SQL's ALTER TABLE Statement: Dynamically Adding Columns to Your Database In data management, SQL's adaptability is crucial. Need to adjust your database structure on the fly? The ALTER TABLE statement is your solution. This guide details adding colu
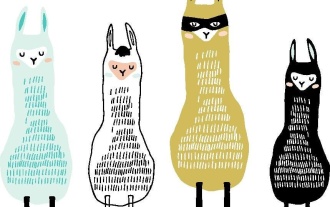
Troubled Benchmarks: A Llama Case Study In early April 2025, Meta unveiled its Llama 4 suite of models, boasting impressive performance metrics that positioned them favorably against competitors like GPT-4o and Claude 3.5 Sonnet. Central to the launc
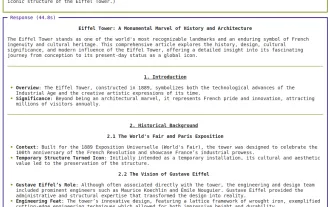
While working on Agentic AI, developers often find themselves navigating the trade-offs between speed, flexibility, and resource efficiency. I have been exploring the Agentic AI framework and came across Agno (earlier it was Phi-
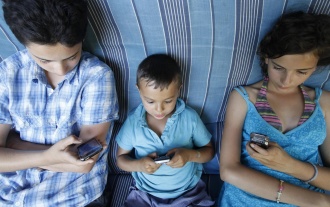
Can a video game ease anxiety, build focus, or support a child with ADHD? As healthcare challenges surge globally — especially among youth — innovators are turning to an unlikely tool: video games. Now one of the world’s largest entertainment indus
