Why RAG Fails and How to Fix It?
Retrieval-Augmented Generation (RAG) significantly enhances Large Language Models (LLMs) by incorporating external knowledge sources, resulting in more accurate and contextually relevant responses. However, RAG systems are not without their flaws, frequently producing inaccurate or irrelevant outputs. These limitations hinder the application of RAG across various fields, including customer service, research, and content creation. Understanding these shortcomings is vital for developing more reliable retrieval-based AI. This article delves into the reasons behind RAG failures and explores strategies to boost RAG performance, leading to more efficient and scalable systems. Improved RAG models promise more consistent, high-quality AI outputs.
Table of Contents
- What is RAG?
- RAG's Limitations
- Retrieval Process Failures and Solutions
- Query-Document Mismatches
- Deficiencies in Search/Retrieval Algorithms
- Chunking Challenges
- Embedding Issues in RAG Systems
- Inefficient Retrieval Problems
- Generation Process Failures and Solutions
- Context Integration Difficulties
- Reasoning Limitations
- Response Formatting Problems
- Context Window Management
- System-Level Failures and Solutions
- Time and Latency Issues
- Evaluation Difficulties
- Architectural Constraints
- Cost and Resource Optimization
- Conclusion
- Frequently Asked Questions
What is RAG?
RAG, or Retrieval-Augmented Generation, is a sophisticated natural language processing technique that combines retrieval methods with generative AI models to deliver more precise and contextually appropriate answers. Unlike models relying solely on training data, RAG dynamically accesses external information to inform its responses.
Key RAG Components:
- Retrieval System: This component extracts relevant information from external sources, providing up-to-date knowledge. A robust retrieval system is crucial for high-quality responses; a poorly designed one can lead to inaccuracies or missing information.
- Generative Model: An LLM processes retrieved data and user queries to generate coherent responses. The accuracy of the generative model depends heavily on the quality of the retrieved data.
- System Configuration: This manages retrieval strategies, model parameters, indexing, and validation to optimize speed, accuracy, and efficiency. Effective configuration is essential for a well-functioning system.
Learn More: Understanding Retrieval Augmented Generation (RAG)
RAG's Limitations
While RAG enhances LLMs by incorporating external knowledge, improving accuracy and contextual relevance, it faces significant challenges that limit its overall reliability and effectiveness. Recognizing these limitations is crucial for developing more robust systems.
These limitations fall into three main categories:
- Retrieval Process Failures
- Generation Process Failures
- System-Level Failures
By addressing these issues and implementing targeted improvements, we can build more reliable and effective RAG systems.
Watch This to Learn More: Addressing Real-World Challenges in RAG Systems
(The remaining sections detailing Retrieval Process Failures, Generation Process Failures, System-Level Failures, Conclusion, and FAQs would follow a similar pattern of rephrasing and restructuring, maintaining the original content and image placement.)
The above is the detailed content of Why RAG Fails and How to Fix It?. For more information, please follow other related articles on the PHP Chinese website!

Hot AI Tools

Undresser.AI Undress
AI-powered app for creating realistic nude photos

AI Clothes Remover
Online AI tool for removing clothes from photos.

Undress AI Tool
Undress images for free

Clothoff.io
AI clothes remover

Video Face Swap
Swap faces in any video effortlessly with our completely free AI face swap tool!

Hot Article

Hot Tools

Notepad++7.3.1
Easy-to-use and free code editor

SublimeText3 Chinese version
Chinese version, very easy to use

Zend Studio 13.0.1
Powerful PHP integrated development environment

Dreamweaver CS6
Visual web development tools

SublimeText3 Mac version
God-level code editing software (SublimeText3)

Hot Topics










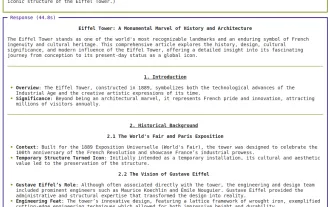
While working on Agentic AI, developers often find themselves navigating the trade-offs between speed, flexibility, and resource efficiency. I have been exploring the Agentic AI framework and came across Agno (earlier it was Phi-
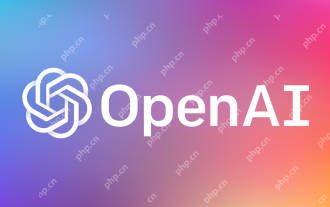
The release includes three distinct models, GPT-4.1, GPT-4.1 mini and GPT-4.1 nano, signaling a move toward task-specific optimizations within the large language model landscape. These models are not immediately replacing user-facing interfaces like
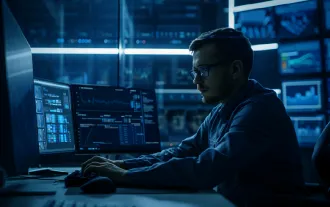
SQL's ALTER TABLE Statement: Dynamically Adding Columns to Your Database In data management, SQL's adaptability is crucial. Need to adjust your database structure on the fly? The ALTER TABLE statement is your solution. This guide details adding colu
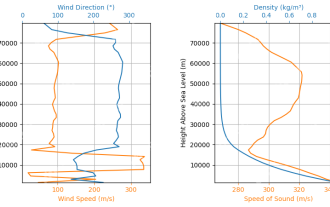
Simulate Rocket Launches with RocketPy: A Comprehensive Guide This article guides you through simulating high-power rocket launches using RocketPy, a powerful Python library. We'll cover everything from defining rocket components to analyzing simula
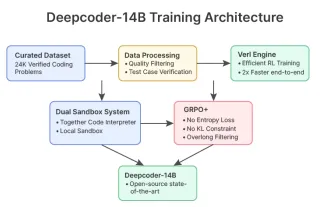
In a significant development for the AI community, Agentica and Together AI have released an open-source AI coding model named DeepCoder-14B. Offering code generation capabilities on par with closed-source competitors like OpenAI
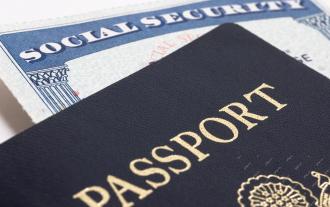
Chip giant Nvidia said on Monday it will start manufacturing AI supercomputers— machines that can process copious amounts of data and run complex algorithms— entirely within the U.S. for the first time. The announcement comes after President Trump si
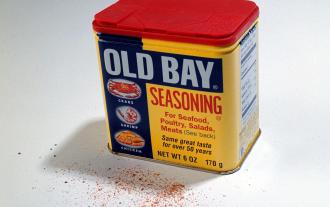
Guy Peri is McCormick’s Chief Information and Digital Officer. Though only seven months into his role, Peri is rapidly advancing a comprehensive transformation of the company’s digital capabilities. His career-long focus on data and analytics informs
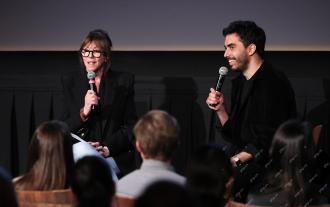
The film industry, alongside all creative sectors, from digital marketing to social media, stands at a technological crossroad. As artificial intelligence begins to reshape every aspect of visual storytelling and change the landscape of entertainment
