How to Build Multimodal RAG Using Docling?
Unlocking Multimodal AI with Docling: A Guide to Building Retrieval-Augmented Generation Systems
Artificial intelligence (AI) is revolutionizing data processing, and Multimodal Retrieval-Augmented Generation (RAG) is at the forefront of this transformation. RAG systems excel at handling diverse data types—text, images, audio, and video—a critical capability for navigating the predominantly unstructured data found in many enterprises. This capability enhances contextual understanding, improves accuracy, and broadens AI's application across various sectors, including healthcare, customer service, and education.
This article explores Docling, an open-source toolkit from IBM designed to simplify document processing for generative AI applications, specifically focusing on building multimodal RAG capabilities. Docling converts diverse file formats (PDFs, DOCX, images, etc.) into structured outputs (JSON, Markdown), seamlessly integrating with popular AI frameworks like LangChain and LlamaIndex. This simplifies the extraction of unstructured data and supports advanced layout analysis, making complex enterprise data accessible for AI-driven insights.
Key Learning Objectives:
- Understanding Docling: Learn how Docling extracts multimodal information from unstructured files.
- Docling's Architecture: Examine Docling's pipeline and core AI components.
- Docling's Distinctive Features: Discover what sets Docling apart from other solutions.
- Building a Multimodal RAG System: Implement a system using Docling for data extraction and retrieval.
- End-to-End Workflow: Master the process of extracting data from a PDF, generating image descriptions, and querying a vector database using Phi 4.
Docling for Unstructured Data Processing:
Docling, an open-source toolkit from IBM, efficiently converts unstructured files (PDFs, DOCX, images) into structured formats (JSON, Markdown). Leveraging advanced AI models like DocLayNet (for layout analysis) and TableFormer (for table recognition), Docling accurately extracts text, tables, and images while preserving the document's structure. Its seamless integration with LangChain and LlamaIndex supports RAG and question-answering applications. Its lightweight design ensures efficient performance on standard hardware, offering a cost-effective alternative to cloud-based solutions and prioritizing data privacy.
The Docling Pipeline:
Docling employs a linear pipeline. Documents are initially parsed (PDF backend), extracting text tokens with coordinates and rendering page bitmaps. AI models then process each page independently to extract layout and table structures. Finally, a post-processing stage aggregates page results, adds metadata, detects language, infers reading order, and assembles a structured document object (JSON or Markdown).
Core AI Models within Docling:
Docling moves beyond traditional, computationally expensive OCR. It utilizes computer vision models specifically trained for visual component identification and categorization.
- Layout Analysis Model: Based on RT-DETR and trained using DocLayNet (a large, human-annotated dataset), this model acts as an object detector, identifying and classifying elements like text blocks, images, tables, and captions. It processes images at 72 dpi, enabling efficient CPU processing.
- TableFormer Model: This vision-transformer model excels at reconstructing table structures from images, handling complexities like missing borders, empty cells, and inconsistent formatting.
Docling's Key Advantages:
- Versatile Format Support: Processes PDFs, DOCX, PPTX, HTML, images, and more, exporting to JSON and Markdown.
- Advanced PDF Handling: Includes layout analysis, reading order detection, table recognition, and OCR (optional) for scanned documents.
- Unified Document Representation: Uses a consistent format for easier processing and analysis.
- AI-Ready Integration: Seamlessly integrates with LangChain and LlamaIndex.
- Local Execution: Enables secure processing of sensitive data.
- Efficient Performance: Significantly faster than traditional OCR.
- Modular Architecture: Easily customizable and extensible.
- Open-Source Availability: Freely available under the MIT license.
Building a Multimodal RAG System with Docling (Python Implementation):
This section details building a RAG system using Docling, extracting text, images, and tables from a PDF, generating image descriptions, and querying a vector database. The complete code is available in a Google Colab notebook (link provided in the original article). The steps involve installing libraries, loading the Docling converter, chunking text, processing tables, encoding images, using a vision language model (e.g., llama3.2-vision via Ollama) for image description generation, storing data in a vector database (e.g., Milvus), and querying the system using an LLM (e.g., Phi 4 via Ollama). The example uses a sample PDF ("accenture.pdf") with charts to demonstrate multimodal retrieval.
(Note: The detailed code snippets from the original article would be included here, but due to length constraints, they are omitted. Refer to the original article for the complete code.)
Analyzing the RAG System:
The article demonstrates querying the system with several questions, showcasing its ability to accurately retrieve and synthesize information from text, tables, and image descriptions within the PDF. The results are visually confirmed using screenshots from the PDF.
Conclusion:
Docling is a powerful tool for transforming unstructured data into a format suitable for generative AI. Its combination of advanced AI models, seamless framework integration, and open-source nature makes it a valuable asset for building robust and efficient multimodal RAG systems. Its cost-effectiveness and support for local execution are particularly beneficial for enterprises handling sensitive information.
(Note: The "Frequently Asked Questions" section from the original article is omitted here due to length constraints. It provides further clarification on RAG, Docling's capabilities, and its suitability for enterprise use.)
The above is the detailed content of How to Build Multimodal RAG Using Docling?. For more information, please follow other related articles on the PHP Chinese website!

Hot AI Tools

Undresser.AI Undress
AI-powered app for creating realistic nude photos

AI Clothes Remover
Online AI tool for removing clothes from photos.

Undress AI Tool
Undress images for free

Clothoff.io
AI clothes remover

Video Face Swap
Swap faces in any video effortlessly with our completely free AI face swap tool!

Hot Article

Hot Tools

Notepad++7.3.1
Easy-to-use and free code editor

SublimeText3 Chinese version
Chinese version, very easy to use

Zend Studio 13.0.1
Powerful PHP integrated development environment

Dreamweaver CS6
Visual web development tools

SublimeText3 Mac version
God-level code editing software (SublimeText3)

Hot Topics
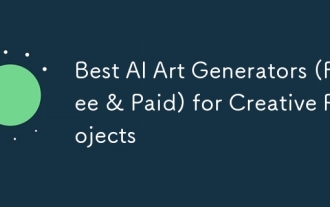
The article reviews top AI art generators, discussing their features, suitability for creative projects, and value. It highlights Midjourney as the best value for professionals and recommends DALL-E 2 for high-quality, customizable art.
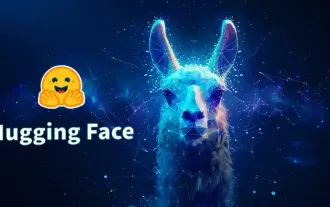
Meta's Llama 3.2: A Leap Forward in Multimodal and Mobile AI Meta recently unveiled Llama 3.2, a significant advancement in AI featuring powerful vision capabilities and lightweight text models optimized for mobile devices. Building on the success o
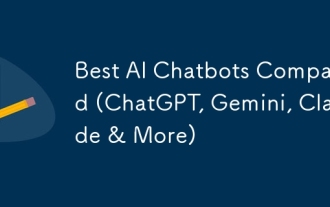
The article compares top AI chatbots like ChatGPT, Gemini, and Claude, focusing on their unique features, customization options, and performance in natural language processing and reliability.
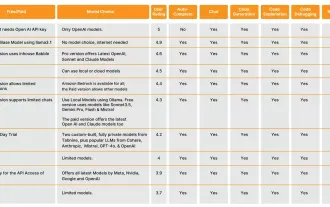
Hey there, Coding ninja! What coding-related tasks do you have planned for the day? Before you dive further into this blog, I want you to think about all your coding-related woes—better list those down. Done? – Let’
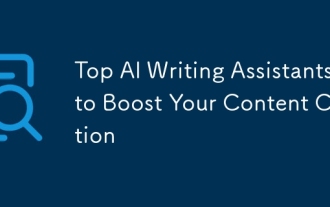
The article discusses top AI writing assistants like Grammarly, Jasper, Copy.ai, Writesonic, and Rytr, focusing on their unique features for content creation. It argues that Jasper excels in SEO optimization, while AI tools help maintain tone consist
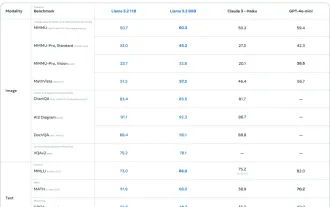
This week's AI landscape: A whirlwind of advancements, ethical considerations, and regulatory debates. Major players like OpenAI, Google, Meta, and Microsoft have unleashed a torrent of updates, from groundbreaking new models to crucial shifts in le
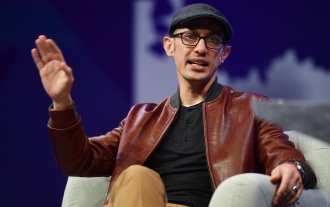
Shopify CEO Tobi Lütke's recent memo boldly declares AI proficiency a fundamental expectation for every employee, marking a significant cultural shift within the company. This isn't a fleeting trend; it's a new operational paradigm integrated into p
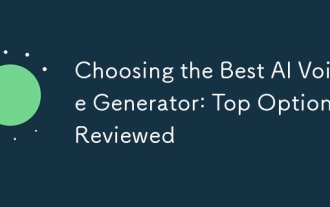
The article reviews top AI voice generators like Google Cloud, Amazon Polly, Microsoft Azure, IBM Watson, and Descript, focusing on their features, voice quality, and suitability for different needs.
