Agentic RAG for Analyzing Customer Issues
This article explores Agentic RAG, an advanced AI technique that significantly improves the capabilities of Large Language Models (LLMs). Unlike traditional, or "Naive," RAG, which passively retrieves information, Agentic RAG incorporates autonomous agents to actively manage data retrieval and decision-making processes. This enhancement allows for more sophisticated reasoning and handling of complex queries.
Agentic RAG: A Powerful Enhancement
Agentic RAG combines the strengths of Retrieval-Augmented Generation (RAG) with the decision-making power of AI agents. This hybrid approach creates a framework where retrieval and generation are integrated within a multi-agent system. Agents can request specific information and make informed decisions based on the retrieved data, resulting in more accurate and contextually relevant responses.
Agentic RAG vs. Naive RAG: Key Differences
The core difference lies in the active role of agents. Naive RAG simply retrieves data when requested, while Agentic RAG uses agents to determine when, how, and what to retrieve. This proactive approach is crucial for handling complex tasks that require multi-step reasoning. Naive RAG struggles with:
- Summarization: Synthesizing information from multiple sources.
- Comparison: Analyzing and contrasting data from different sources.
- Multi-part Queries: Addressing questions requiring sequential steps and information gathering.
Real-World Applications of Agentic RAG
The addition of AI agents unlocks numerous applications requiring multi-step reasoning:
- Legal Research: Comparing legal documents and identifying key clauses.
- Market Analysis: Conducting competitive analyses of leading brands.
- Medical Diagnosis: Integrating patient data with the latest research.
- Financial Analysis: Processing financial reports and generating key investment insights.
- Compliance: Ensuring regulatory compliance by comparing policies with laws.
Building Agentic RAG with Python and CrewAI
This section demonstrates building an Agentic RAG system using Python and CrewAI to analyze customer support tickets. The example uses a dataset of customer issues for various tech products.
The system summarizes top customer complaints for each brand. The steps involve:
-
Installing Libraries: Installing necessary Python packages (
llama-index
,crewai
). - Importing Libraries: Importing required modules.
- Reading Data: Loading the customer issue dataset.
- Setting API Key: Configuring the OpenAI API key.
- LLM Initialization: Initializing the Large Language Model.
- Creating Index and Query Engine: Building a vector store index for efficient searching.
- Creating a Tool: Creating a tool based on the query engine.
- Defining Agents: Defining agents with specific roles ("Customer Ticket Analyst," "Product Content Specialist").
- Creating Tasks: Assigning tasks to agents.
- Instantiating the Crew: Running the agents and tasks sequentially.
Conclusion: The Future of RAG
Agentic RAG represents a significant advancement in Retrieval-Augmented Generation. Its ability to handle complex queries and provide more nuanced insights makes it a powerful tool across various industries. The use of Python and CrewAI simplifies the implementation process, making this technology more accessible to developers.
Key Takeaways:
- Agentic RAG's dynamic decision-making surpasses Naive RAG's limitations.
- It excels in complex queries requiring multi-step reasoning.
- It finds applications in diverse fields demanding advanced data analysis.
- CrewAI facilitates straightforward Python implementation.
- It's adaptable to various data analysis scenarios.
Frequently Asked Questions (FAQ):
-
Q1: What's the key difference between Agentic and Naive RAG? A1: Agentic RAG uses active agents for decision-making, while Naive RAG passively retrieves information.
-
Q2: Why does Naive RAG struggle with complex queries? A2: Its passive nature limits its ability to handle multi-step reasoning and complex information synthesis.
-
Q3: How is Agentic RAG applied in real-world scenarios? A3: It's used in legal, medical, financial, and customer support domains for advanced data analysis.
-
Q4: Can I implement Agentic RAG using Python? A4: Yes, using libraries like CrewAI.
-
Q5: Which industries benefit most from Agentic RAG? A5: Industries dealing with complex data analysis, such as law, healthcare, finance, and customer support.
(Note: Image URLs remain unchanged.)
The above is the detailed content of Agentic RAG for Analyzing Customer Issues. For more information, please follow other related articles on the PHP Chinese website!

Hot AI Tools

Undresser.AI Undress
AI-powered app for creating realistic nude photos

AI Clothes Remover
Online AI tool for removing clothes from photos.

Undress AI Tool
Undress images for free

Clothoff.io
AI clothes remover

Video Face Swap
Swap faces in any video effortlessly with our completely free AI face swap tool!

Hot Article

Hot Tools

Notepad++7.3.1
Easy-to-use and free code editor

SublimeText3 Chinese version
Chinese version, very easy to use

Zend Studio 13.0.1
Powerful PHP integrated development environment

Dreamweaver CS6
Visual web development tools

SublimeText3 Mac version
God-level code editing software (SublimeText3)

Hot Topics










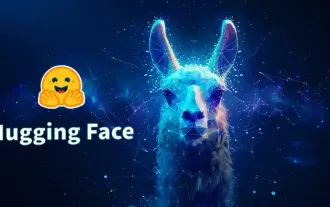
Meta's Llama 3.2: A Leap Forward in Multimodal and Mobile AI Meta recently unveiled Llama 3.2, a significant advancement in AI featuring powerful vision capabilities and lightweight text models optimized for mobile devices. Building on the success o
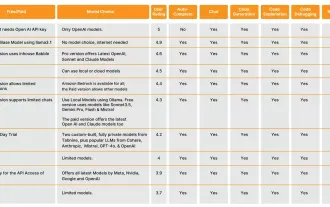
Hey there, Coding ninja! What coding-related tasks do you have planned for the day? Before you dive further into this blog, I want you to think about all your coding-related woes—better list those down. Done? – Let’
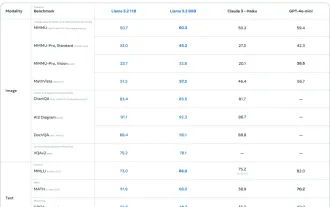
This week's AI landscape: A whirlwind of advancements, ethical considerations, and regulatory debates. Major players like OpenAI, Google, Meta, and Microsoft have unleashed a torrent of updates, from groundbreaking new models to crucial shifts in le
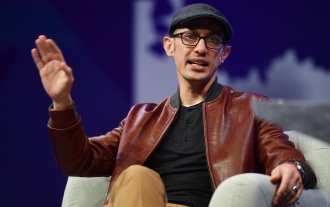
Shopify CEO Tobi Lütke's recent memo boldly declares AI proficiency a fundamental expectation for every employee, marking a significant cultural shift within the company. This isn't a fleeting trend; it's a new operational paradigm integrated into p
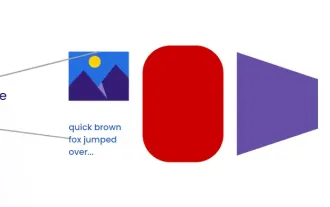
Introduction Imagine walking through an art gallery, surrounded by vivid paintings and sculptures. Now, what if you could ask each piece a question and get a meaningful answer? You might ask, “What story are you telling?
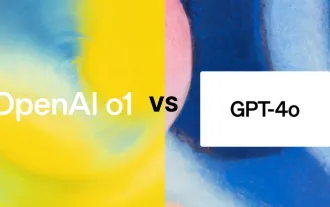
Introduction OpenAI has released its new model based on the much-anticipated “strawberry” architecture. This innovative model, known as o1, enhances reasoning capabilities, allowing it to think through problems mor
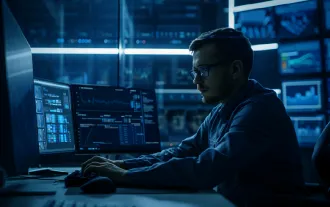
SQL's ALTER TABLE Statement: Dynamically Adding Columns to Your Database In data management, SQL's adaptability is crucial. Need to adjust your database structure on the fly? The ALTER TABLE statement is your solution. This guide details adding colu
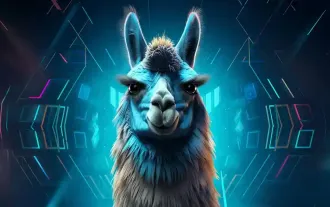
Meta's Llama 3.2: A Multimodal AI Powerhouse Meta's latest multimodal model, Llama 3.2, represents a significant advancement in AI, boasting enhanced language comprehension, improved accuracy, and superior text generation capabilities. Its ability t
