A Guide to 400 Categorized Large Language Model Datasets
This groundbreaking survey, "Datasets for Large Language Models: A Comprehensive Survey," released in February 2024, unveils a treasure trove of over 400 meticulously categorized datasets for Large Language Model (LLM) development. Compiled by Yang Liu, Jiahuan Cao, Chongyu Liu, Kai Ding, and Lianwen Jin, this resource is a goldmine for researchers and developers. It's not just a static collection; it's regularly updated, ensuring its continued relevance.
The paper provides a comprehensive overview of LLM datasets, essential for understanding the foundation of these powerful models. The datasets are categorized across seven key dimensions: Pre-training Corpora, Instruction Fine-tuning Datasets, Preference Datasets, Evaluation Datasets, Traditional NLP Datasets, Multi-modal Large Language Models (MLLMs) Datasets, and Retrieval Augmented Generation (RAG) Datasets. The sheer scale is impressive, with over 774.5 TB of data for pre-training alone and 700 million instances across other categories, spanning 32 domains and 8 languages.
Key Dataset Categories and Examples:
The survey details various dataset types, including:
-
Pre-training Corpora: Massive text collections for initial LLM training. Examples include MADLAD-400 (2.8T tokens), FineWeb (15TB tokens), and BookCorpusOpen (17,868 books). These are further broken down into general corpora (webpages, books, language texts) and domain-specific corpora (finance, medical, mathematics).
-
Instruction Fine-tuning Datasets: Pairs of instructions and corresponding answers to refine model behavior. Examples include databricks-dolly-15K and Alpaca_data. These are also categorized into general and domain-specific (medical, code) datasets.
-
Preference Datasets: Used to evaluate and improve model outputs by comparing multiple responses. Examples include Chatbot_arena_conversations and hh-rlhf.
-
Evaluation Datasets: Specifically designed to benchmark LLM performance on various tasks. Examples include AlpacaEval and BayLing-80.
-
Traditional NLP Datasets: Datasets used for pre-LLM NLP tasks. Examples include BoolQ, CosmosQA, and PubMedQA.
-
Multi-modal Large Language Models (MLLMs) Datasets: Datasets combining text and other modalities (images, videos). Examples include mOSCAR and MMRS-1M.
-
Retrieval Augmented Generation (RAG) Datasets: Datasets that enhance LLMs with external data retrieval capabilities. Examples include CRUD-RAG and WikiEval.
Source: Datasets for Large Language Models: A Comprehensive Survey
The survey's architecture is illustrated below:
Conclusion and Further Exploration:
This survey serves as a vital resource, guiding researchers and developers in the LLM field. The provided repository (Awesome-LLMs-Datasets) offers a complete roadmap for accessing and utilizing these invaluable datasets. The detailed categorization and comprehensive statistics make it an essential tool for anyone working with or researching LLMs. The paper also addresses key challenges and suggests future research directions.
The above is the detailed content of A Guide to 400 Categorized Large Language Model Datasets. For more information, please follow other related articles on the PHP Chinese website!

Hot AI Tools

Undresser.AI Undress
AI-powered app for creating realistic nude photos

AI Clothes Remover
Online AI tool for removing clothes from photos.

Undress AI Tool
Undress images for free

Clothoff.io
AI clothes remover

Video Face Swap
Swap faces in any video effortlessly with our completely free AI face swap tool!

Hot Article

Hot Tools

Notepad++7.3.1
Easy-to-use and free code editor

SublimeText3 Chinese version
Chinese version, very easy to use

Zend Studio 13.0.1
Powerful PHP integrated development environment

Dreamweaver CS6
Visual web development tools

SublimeText3 Mac version
God-level code editing software (SublimeText3)

Hot Topics










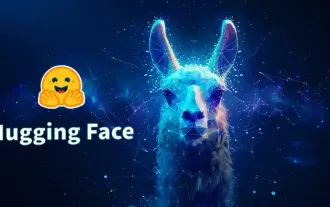
Meta's Llama 3.2: A Leap Forward in Multimodal and Mobile AI Meta recently unveiled Llama 3.2, a significant advancement in AI featuring powerful vision capabilities and lightweight text models optimized for mobile devices. Building on the success o
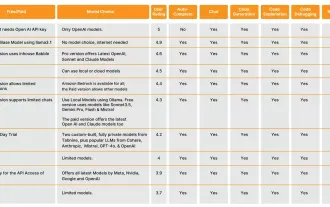
Hey there, Coding ninja! What coding-related tasks do you have planned for the day? Before you dive further into this blog, I want you to think about all your coding-related woes—better list those down. Done? – Let’
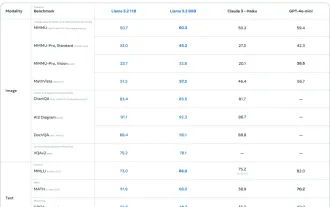
This week's AI landscape: A whirlwind of advancements, ethical considerations, and regulatory debates. Major players like OpenAI, Google, Meta, and Microsoft have unleashed a torrent of updates, from groundbreaking new models to crucial shifts in le
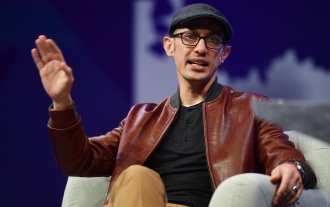
Shopify CEO Tobi Lütke's recent memo boldly declares AI proficiency a fundamental expectation for every employee, marking a significant cultural shift within the company. This isn't a fleeting trend; it's a new operational paradigm integrated into p
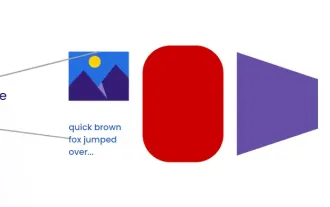
Introduction Imagine walking through an art gallery, surrounded by vivid paintings and sculptures. Now, what if you could ask each piece a question and get a meaningful answer? You might ask, “What story are you telling?
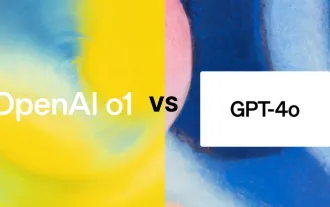
Introduction OpenAI has released its new model based on the much-anticipated “strawberry” architecture. This innovative model, known as o1, enhances reasoning capabilities, allowing it to think through problems mor
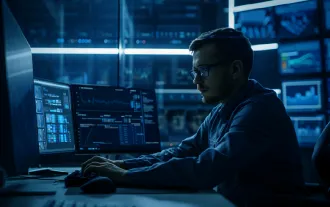
SQL's ALTER TABLE Statement: Dynamically Adding Columns to Your Database In data management, SQL's adaptability is crucial. Need to adjust your database structure on the fly? The ALTER TABLE statement is your solution. This guide details adding colu
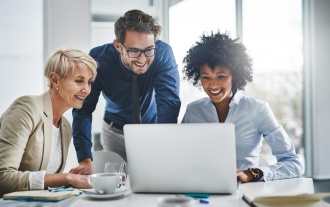
For those of you who might be new to my column, I broadly explore the latest advances in AI across the board, including topics such as embodied AI, AI reasoning, high-tech breakthroughs in AI, prompt engineering, training of AI, fielding of AI, AI re
