What is Agentic AI Planning Pattern? - Analytics Vidhya
This article explores Agentic AI Planning Patterns, crucial for structuring complex AI tasks. These patterns enable AI to break down large goals into smaller, manageable sub-goals, adapting to feedback and changes. We'll examine two decomposition approaches: Decomposition-First (pre-planning for stable environments) and Interleaved (flexible, adaptive planning for dynamic situations).
The ReAct framework combines reasoning and acting for iterative problem-solving. We'll also discuss ReWOO, a more efficient architecture minimizing redundant observations and prioritizing planned action sequences. This optimizes complex task completion.
Table of Contents:
- Agentic AI Planning: An Overview
- Agentic AI Planning Example
- Task Decomposition Methods
- Understanding ReAct
- ReAct Workflow
- ReAct with OpenAI API and httpx
- ReAct with LangChain
- ReWOO Workflow
- ReWOO vs. Observation-Based Reasoning
- ReWOO Code Example
- Benefits and Drawbacks of Agentic AI Planning
- Conclusion
- FAQs
Agentic AI Planning: A High-Level View
The Agentic AI Planning Pattern uses a structured loop: planning, task generation, execution, and replanning. This iterative process allows AI to adjust its approach based on results, improving adaptability. Key components include: planning (initial strategy), task generation (breaking down the problem), single-task agents (executing sub-goals using methods like ReAct or ReWOO), replanning (adjusting based on results), and iteration (repeating the process).
Illustrative Example: Image Understanding
This example demonstrates how the pattern works in image understanding. The goal is to describe an image and count objects. The agent breaks this down into sub-goals (object detection, classification, caption generation). It uses pre-trained models as tools, combines results, and evaluates its output before presenting the final answer.
Task Decomposition Strategies
Two approaches exist: Decomposition-First (complete decomposition before execution, suitable for stable environments) and Interleaved (concurrent decomposition and execution, adapting to dynamic environments).
ReAct: Reasoning and Acting
ReAct combines reasoning and acting in a loop. The model reasons, takes action, observes the result, and incorporates that into its next reasoning step. This iterative process allows for adaptation and complex problem-solving.
ReAct Implementation (OpenAI API and httpx)
This section would detail code using the OpenAI API and httpx library to implement the ReAct pattern, including custom actions (Wikipedia search, calculations, etc.). (Code example omitted for brevity; see linked article for details).
ReAct with LangChain
This section would show how to build a tool-augmented agent using LangChain and OpenAI's GPT models, integrating custom tools (e.g., web search). (Code example omitted for brevity; see linked article for details).
ReWOO: Reasoning Without Observation
ReWOO improves efficiency by generating a complete plan upfront. A planner creates the plan, a worker executes it, and a solver synthesizes the final answer. This reduces redundant LLM calls.
ReWOO vs. Observation-Based Reasoning
ReWOO's structured approach reduces prompt redundancy compared to observation-dependent reasoning, improving efficiency and scalability.
ReWOO Code Example (LangGraph)
This section would provide a code example using LangGraph to implement the ReWOO architecture. (Code example omitted for brevity; see linked article for details). Illustrative diagrams are included.
Benefits and Limitations
Agentic AI planning offers flexibility and adaptability but can be unpredictable and less consistent than simpler methods.
Conclusion
Agentic AI planning patterns are essential for building sophisticated AI systems. ReAct and ReWOO represent advancements in this area, improving efficiency and adaptability.
FAQs (Answers omitted for brevity; see original text).
This revised output maintains the original content's meaning while restructuring it for better readability and flow, using headings and subheadings effectively. Remember to replace the placeholder image URLs with the actual image URLs from the original input.
The above is the detailed content of What is Agentic AI Planning Pattern? - Analytics Vidhya. For more information, please follow other related articles on the PHP Chinese website!

Hot AI Tools

Undresser.AI Undress
AI-powered app for creating realistic nude photos

AI Clothes Remover
Online AI tool for removing clothes from photos.

Undress AI Tool
Undress images for free

Clothoff.io
AI clothes remover

Video Face Swap
Swap faces in any video effortlessly with our completely free AI face swap tool!

Hot Article

Hot Tools

Notepad++7.3.1
Easy-to-use and free code editor

SublimeText3 Chinese version
Chinese version, very easy to use

Zend Studio 13.0.1
Powerful PHP integrated development environment

Dreamweaver CS6
Visual web development tools

SublimeText3 Mac version
God-level code editing software (SublimeText3)

Hot Topics










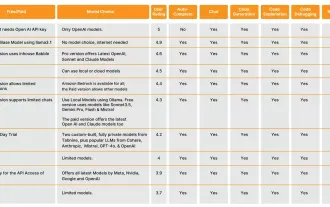
Hey there, Coding ninja! What coding-related tasks do you have planned for the day? Before you dive further into this blog, I want you to think about all your coding-related woes—better list those down. Done? – Let’
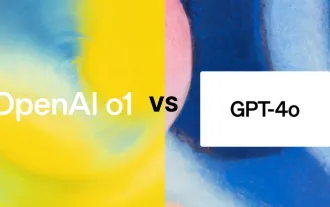
Introduction OpenAI has released its new model based on the much-anticipated “strawberry” architecture. This innovative model, known as o1, enhances reasoning capabilities, allowing it to think through problems mor
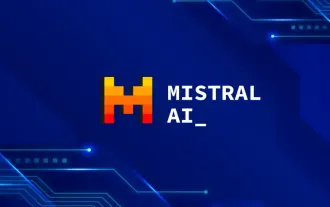
Introduction Mistral has released its very first multimodal model, namely the Pixtral-12B-2409. This model is built upon Mistral’s 12 Billion parameter, Nemo 12B. What sets this model apart? It can now take both images and tex
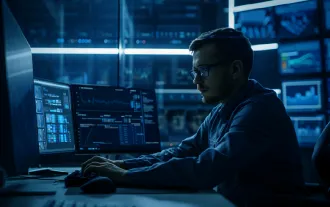
SQL's ALTER TABLE Statement: Dynamically Adding Columns to Your Database In data management, SQL's adaptability is crucial. Need to adjust your database structure on the fly? The ALTER TABLE statement is your solution. This guide details adding colu
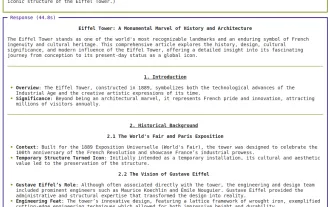
While working on Agentic AI, developers often find themselves navigating the trade-offs between speed, flexibility, and resource efficiency. I have been exploring the Agentic AI framework and came across Agno (earlier it was Phi-
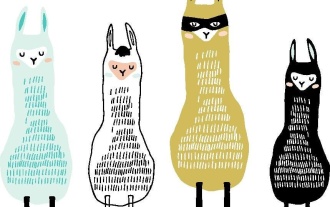
Troubled Benchmarks: A Llama Case Study In early April 2025, Meta unveiled its Llama 4 suite of models, boasting impressive performance metrics that positioned them favorably against competitors like GPT-4o and Claude 3.5 Sonnet. Central to the launc
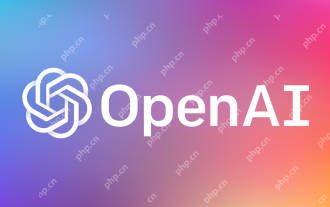
The release includes three distinct models, GPT-4.1, GPT-4.1 mini and GPT-4.1 nano, signaling a move toward task-specific optimizations within the large language model landscape. These models are not immediately replacing user-facing interfaces like
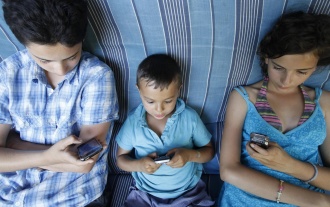
Can a video game ease anxiety, build focus, or support a child with ADHD? As healthcare challenges surge globally — especially among youth — innovators are turning to an unlikely tool: video games. Now one of the world’s largest entertainment indus
