Performance Evaluation of Small Language Models
This article explores the advantages of Small Language Models (SLMs) over their larger counterparts, focusing on their efficiency and suitability for resource-constrained environments. SLMs, with fewer than 10 billion parameters, offer speed and resource efficiency crucial for edge computing and real-time applications. This article details their creation, applications, and implementation using Ollama on Google Colab.
This guide covers:
- Understanding SLMs: Learn the defining characteristics of SLMs and their key differences from LLMs.
- SLM Creation Techniques: Explore knowledge distillation, pruning, and quantization methods used to create efficient SLMs from LLMs.
- Performance Evaluation: Compare the performance of various SLMs (LLaMA 2, Microsoft Phi, Qwen 2, Gemma 2, Mistral 7B) through a comparative analysis of their outputs.
- Practical Implementation: A step-by-step guide to running SLMs on Google Colab using Ollama.
- Applications of SLMs: Discover the diverse applications where SLMs excel, including chatbots, virtual assistants, and edge computing scenarios.
Key Differences: SLMs vs. LLMs
SLMs are significantly smaller than LLMs, requiring less training data and computational resources. This results in faster inference times and lower costs. While LLMs excel at complex, general tasks, SLMs are optimized for specific tasks and are better suited for resource-limited devices. The table below summarizes the key distinctions:
Feature | Small Language Models (SLMs) | Large Language Models (LLMs) |
---|---|---|
Size | Significantly smaller (under 10 billion parameters) | Much larger (hundreds of billions or trillions of parameters) |
Training Data | Smaller, focused datasets | Massive, diverse datasets |
Training Time | Shorter (weeks) | Longer (months) |
Resources | Low computational requirements | High computational requirements |
Task Proficiency | Specialized tasks | General-purpose tasks |
Inference | Can run on edge devices | Typically requires powerful GPUs |
Response Time | Faster | Slower |
Cost | Lower | Higher |
Building SLMs: Techniques and Examples
This section details the methods used to create SLMs from LLMs:
- Knowledge Distillation: A smaller "student" model learns from the outputs of a larger "teacher" model.
- Pruning: Removes less important connections or neurons in the larger model.
- Quantization: Reduces the precision of model parameters, lowering memory requirements.
The article then presents a detailed comparison of several state-of-the-art SLMs, including LLaMA 2, Microsoft Phi, Qwen 2, Gemma 2, and Mistral 7B, highlighting their unique features and performance benchmarks.
Running SLMs with Ollama on Google Colab
A practical guide demonstrates how to use Ollama to run SLMs on Google Colab, providing code snippets for installation, model selection, and prompt execution. The article showcases the outputs from different models, allowing for a direct comparison of their performance on a sample task.
Conclusion and FAQs
The article concludes by summarizing the advantages of SLMs and their suitability for various applications. A frequently asked questions section addresses common queries about SLMs, knowledge distillation, and the differences between pruning and quantization. The key takeaway emphasizes the balance SLMs achieve between efficiency and performance, making them valuable tools for developers and businesses.
The above is the detailed content of Performance Evaluation of Small Language Models. For more information, please follow other related articles on the PHP Chinese website!

Hot AI Tools

Undresser.AI Undress
AI-powered app for creating realistic nude photos

AI Clothes Remover
Online AI tool for removing clothes from photos.

Undress AI Tool
Undress images for free

Clothoff.io
AI clothes remover

Video Face Swap
Swap faces in any video effortlessly with our completely free AI face swap tool!

Hot Article

Hot Tools

Notepad++7.3.1
Easy-to-use and free code editor

SublimeText3 Chinese version
Chinese version, very easy to use

Zend Studio 13.0.1
Powerful PHP integrated development environment

Dreamweaver CS6
Visual web development tools

SublimeText3 Mac version
God-level code editing software (SublimeText3)

Hot Topics










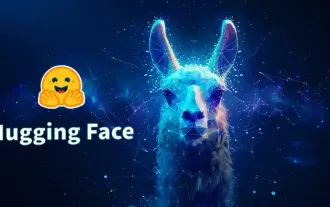
Meta's Llama 3.2: A Leap Forward in Multimodal and Mobile AI Meta recently unveiled Llama 3.2, a significant advancement in AI featuring powerful vision capabilities and lightweight text models optimized for mobile devices. Building on the success o
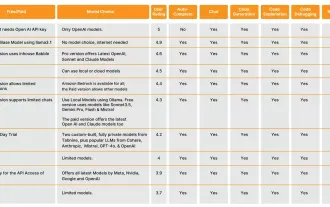
Hey there, Coding ninja! What coding-related tasks do you have planned for the day? Before you dive further into this blog, I want you to think about all your coding-related woes—better list those down. Done? – Let’
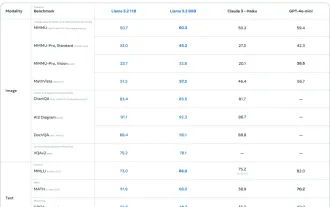
This week's AI landscape: A whirlwind of advancements, ethical considerations, and regulatory debates. Major players like OpenAI, Google, Meta, and Microsoft have unleashed a torrent of updates, from groundbreaking new models to crucial shifts in le
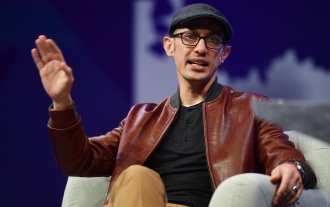
Shopify CEO Tobi Lütke's recent memo boldly declares AI proficiency a fundamental expectation for every employee, marking a significant cultural shift within the company. This isn't a fleeting trend; it's a new operational paradigm integrated into p
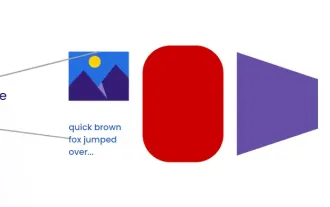
Introduction Imagine walking through an art gallery, surrounded by vivid paintings and sculptures. Now, what if you could ask each piece a question and get a meaningful answer? You might ask, “What story are you telling?
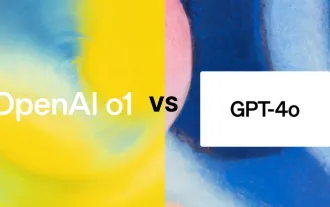
Introduction OpenAI has released its new model based on the much-anticipated “strawberry” architecture. This innovative model, known as o1, enhances reasoning capabilities, allowing it to think through problems mor
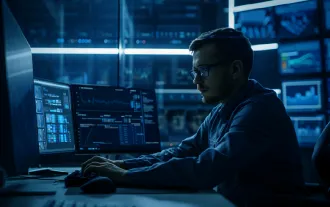
SQL's ALTER TABLE Statement: Dynamically Adding Columns to Your Database In data management, SQL's adaptability is crucial. Need to adjust your database structure on the fly? The ALTER TABLE statement is your solution. This guide details adding colu
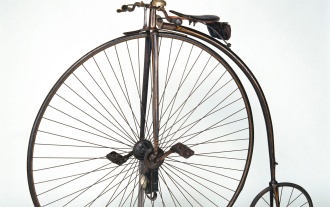
The 2025 Artificial Intelligence Index Report released by the Stanford University Institute for Human-Oriented Artificial Intelligence provides a good overview of the ongoing artificial intelligence revolution. Let’s interpret it in four simple concepts: cognition (understand what is happening), appreciation (seeing benefits), acceptance (face challenges), and responsibility (find our responsibilities). Cognition: Artificial intelligence is everywhere and is developing rapidly We need to be keenly aware of how quickly artificial intelligence is developing and spreading. Artificial intelligence systems are constantly improving, achieving excellent results in math and complex thinking tests, and just a year ago they failed miserably in these tests. Imagine AI solving complex coding problems or graduate-level scientific problems – since 2023
