Building Multi-Vector Chatbot with LangChain, Milvus, and Cohere
This article details building a medical chatbot using multiple vectorstores, enhancing its ability to understand and respond to user queries based on uploaded medical reports and doctor-patient conversations. The chatbot leverages LangChain, Milvus, and Cohere for improved AI interactions.
The learning objectives cover utilizing open-source medical datasets, building vectorstore services, integrating LLMs and embeddings, and constructing a multi-vector chatbot with LangChain, Milvus, and Cohere. The article also explains integrating vectorstores and retrieval mechanisms for context-aware responses.
The construction process is broken down into steps:
-
Importing Libraries and Modules: Necessary Python libraries and modules, including
dotenv
,LangChain
, and custom service modules, are imported for managing environment variables and interacting with various services. -
Loading Data: A medical conversation dataset (downloadable from a provided URL) is loaded using pandas. The data includes patient queries and doctor responses.
-
Ingesting Data: The
Ingestion
class processes and stores the medical data into the vectorstore. Both the conversation dataset and a sample patient report (also downloadable) are ingested. -
Initializing Services: Embeddings, vector store, and LLM services are initialized using factory classes, allowing for flexibility in choosing different providers.
-
Creating Retrievers: Two retrievers are created: one for doctor-patient conversations and another for medical reports. An ensemble retriever combines these for a broader knowledge base.
-
Managing Conversation History: A SQL-based system is used to store and manage chat history for context-aware responses.
-
Generating Responses: A
ChatPromptTemplate
structures the chatbot's responses, guiding it to use retrieved information effectively. -
Creating History Aware RAG Chain: The components are combined to create a Retrieval Augmented Generation (RAG) chain, enabling the chatbot to answer questions based on the combined knowledge from both vectorstores and the conversation history.
The article demonstrates the chatbot's functionality with example queries, showing how it uses information from both the patient report and the conversation dataset to provide relevant answers. The conclusion emphasizes the significance of this approach in advancing AI in healthcare, highlighting the benefits of a flexible and scalable architecture.
Key takeaways reiterate the article's core concepts: building a multi-vector chatbot, integrating vectorstores for context-aware responses, the importance of data processing and model training, personalization, scalability, and the role of embeddings and LLMs.
A FAQ section addresses common questions about medical chatbots, their functionality, vectorstores, personalization, and data privacy.
(Note: The image URLs are placeholders. The actual images from the original input should be included here in their original format.)
The above is the detailed content of Building Multi-Vector Chatbot with LangChain, Milvus, and Cohere. For more information, please follow other related articles on the PHP Chinese website!

Hot AI Tools

Undresser.AI Undress
AI-powered app for creating realistic nude photos

AI Clothes Remover
Online AI tool for removing clothes from photos.

Undress AI Tool
Undress images for free

Clothoff.io
AI clothes remover

Video Face Swap
Swap faces in any video effortlessly with our completely free AI face swap tool!

Hot Article

Hot Tools

Notepad++7.3.1
Easy-to-use and free code editor

SublimeText3 Chinese version
Chinese version, very easy to use

Zend Studio 13.0.1
Powerful PHP integrated development environment

Dreamweaver CS6
Visual web development tools

SublimeText3 Mac version
God-level code editing software (SublimeText3)

Hot Topics










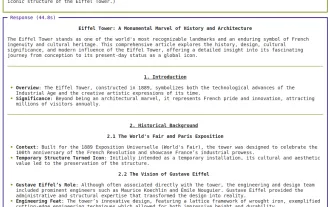
While working on Agentic AI, developers often find themselves navigating the trade-offs between speed, flexibility, and resource efficiency. I have been exploring the Agentic AI framework and came across Agno (earlier it was Phi-
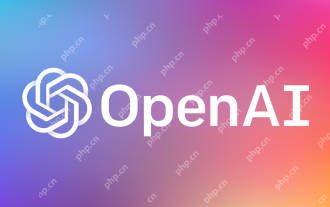
The release includes three distinct models, GPT-4.1, GPT-4.1 mini and GPT-4.1 nano, signaling a move toward task-specific optimizations within the large language model landscape. These models are not immediately replacing user-facing interfaces like
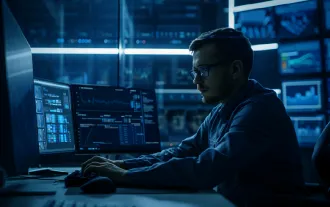
SQL's ALTER TABLE Statement: Dynamically Adding Columns to Your Database In data management, SQL's adaptability is crucial. Need to adjust your database structure on the fly? The ALTER TABLE statement is your solution. This guide details adding colu
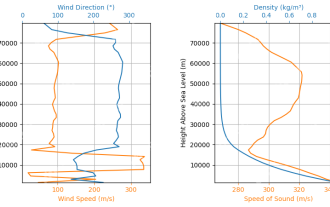
Simulate Rocket Launches with RocketPy: A Comprehensive Guide This article guides you through simulating high-power rocket launches using RocketPy, a powerful Python library. We'll cover everything from defining rocket components to analyzing simula
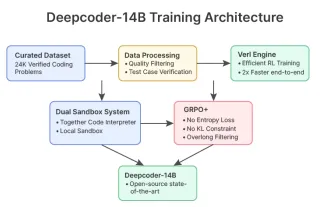
In a significant development for the AI community, Agentica and Together AI have released an open-source AI coding model named DeepCoder-14B. Offering code generation capabilities on par with closed-source competitors like OpenAI
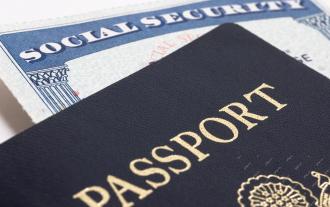
Chip giant Nvidia said on Monday it will start manufacturing AI supercomputers— machines that can process copious amounts of data and run complex algorithms— entirely within the U.S. for the first time. The announcement comes after President Trump si
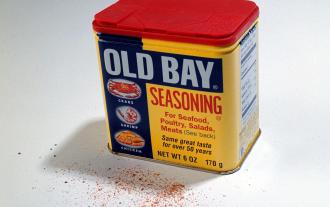
Guy Peri is McCormick’s Chief Information and Digital Officer. Though only seven months into his role, Peri is rapidly advancing a comprehensive transformation of the company’s digital capabilities. His career-long focus on data and analytics informs
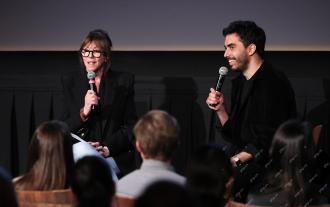
The film industry, alongside all creative sectors, from digital marketing to social media, stands at a technological crossroad. As artificial intelligence begins to reshape every aspect of visual storytelling and change the landscape of entertainment
