


Mastering Prompt Engineering with Functional Testing: A Systematic Guide to Reliable LLM Outputs
Optimizing prompts for large language models (LLMs) can quickly become complex. While initial success might seem easy—using specialist personas, clear instructions, specific formats, and examples—scaling up reveals contradictions and unexpected failures. Minor prompt changes can break previously working aspects. This iterative, trial-and-error approach lacks structure and scientific rigor.
Functional testing offers a solution. Inspired by scientific methodology, it uses automated input-output testing, iterative runs, and algorithmic scoring to make prompt engineering data-driven and repeatable. This eliminates guesswork and manual validation, enabling efficient and confident prompt refinement.
This article details a systematic approach to mastering prompt engineering, ensuring reliable LLM outputs even for intricate AI tasks.
Balancing Precision and Consistency in Prompt Optimization
Adding numerous rules to a prompt can create internal contradictions, leading to unpredictable behavior. This is particularly true when starting with general rules and adding exceptions. Specific rules might conflict with primary instructions or each other. Even minor changes—reordering instructions, rewording, or adding detail—can alter the model's interpretation and prioritization. Over-specification increases the risk of flawed results; finding the right balance between clarity and detail is crucial for consistent, relevant responses. Manual testing becomes overwhelming with multiple competing specifications. A scientific approach prioritizing repeatability and reliability is necessary.
From Laboratory to AI: Iterative Testing for Reliable LLM Responses
Scientific experiments use replicates to ensure reproducibility. Similarly, LLMs require multiple iterations to account for their non-deterministic nature. A single test isn't sufficient due to inherent response variability. At least five iterations per use case are recommended to assess reproducibility and identify inconsistencies. This is especially important when optimizing prompts with numerous competing requirements.
A Systematic Approach: Functional Testing for Prompt Optimization
This structured evaluation methodology includes:
- Data Fixtures: Predefined input-output pairs designed to test various requirements and edge cases. These represent controlled scenarios for efficient evaluation under different conditions.
- Automated Test Validation: Automated comparison of expected outputs (from fixtures) with actual LLM responses. This ensures consistency and minimizes human error.
- Multiple Iterations: Multiple runs for each test case to assess LLM response variability, mirroring scientific triplicates.
- Algorithmic Scoring: Objective, quantitative scoring of results, reducing manual evaluation. This provides clear metrics for data-driven prompt optimization.
Step 1: Defining Test Data Fixtures
Creating effective fixtures is crucial. A fixture isn't just any input-output pair; it must be carefully designed to accurately evaluate LLM performance for a specific requirement. This requires:
- A thorough understanding of the task and model behavior to minimize ambiguity and bias.
- Foresight into algorithmic evaluation.
A fixture includes:
- Input Example: Representative data covering various scenarios.
- Expected Output: The anticipated LLM response for comparison during validation.
Step 2: Running Automated Tests
After defining fixtures, automated tests systematically evaluate LLM performance.
Execution Process:
- Multiple Iterations: The same input is fed to the LLM multiple times (e.g., five iterations).
- Response Comparison: Each response is compared to the expected output.
- Scoring Mechanism: Each comparison results in a pass (1) or fail (0) score.
- Final Score Calculation: Scores are aggregated to calculate an overall score representing the success rate.
Example: Removing Author Signatures from an Article
A simple example involves removing author signatures. Fixtures could include various signature styles. Validation checks for signature absence in the output. A perfect score indicates successful removal; lower scores highlight areas needing prompt adjustment.
Benefits of This Method:
- Reliable results through multiple iterations.
- Efficient process through automation.
- Data-driven optimization.
- Side-by-side evaluation of prompt versions.
- Quick iterative improvement.
Systematic Prompt Testing: Beyond Prompt Optimization
This approach extends beyond initial optimization:
- Model Comparison: Efficiently compare different LLMs (ChatGPT, Claude, etc.) and versions on the same tasks.
- Version Upgrades: Validate prompt performance after model updates.
- Cost Optimization: Determine the best performance-to-cost ratio.
Overcoming Challenges:
The primary challenge is preparing test fixtures. However, the upfront investment pays off significantly in reduced debugging time and improved model efficiency.
Quick Pros and Cons:
Advantages:
- Continuous improvement.
- Better maintenance.
- More flexibility.
- Cost optimization.
- Time savings.
Challenges:
- Initial time investment.
- Defining measurable validation criteria.
- Cost of multiple tests (though often negligible).
Conclusion: When to Implement This Approach
This systematic testing is not always necessary, especially for simple tasks. However, for complex AI tasks requiring high precision and reliability, it's invaluable. It transforms prompt engineering from a subjective process into a measurable, scalable, and robust one. The decision to implement it should depend on project complexity. For high-precision needs, the investment is worthwhile.
The above is the detailed content of Mastering Prompt Engineering with Functional Testing: A Systematic Guide to Reliable LLM Outputs . For more information, please follow other related articles on the PHP Chinese website!

Hot AI Tools

Undresser.AI Undress
AI-powered app for creating realistic nude photos

AI Clothes Remover
Online AI tool for removing clothes from photos.

Undress AI Tool
Undress images for free

Clothoff.io
AI clothes remover

Video Face Swap
Swap faces in any video effortlessly with our completely free AI face swap tool!

Hot Article

Hot Tools

Notepad++7.3.1
Easy-to-use and free code editor

SublimeText3 Chinese version
Chinese version, very easy to use

Zend Studio 13.0.1
Powerful PHP integrated development environment

Dreamweaver CS6
Visual web development tools

SublimeText3 Mac version
God-level code editing software (SublimeText3)

Hot Topics










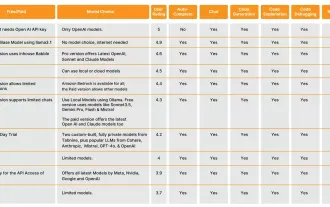
Hey there, Coding ninja! What coding-related tasks do you have planned for the day? Before you dive further into this blog, I want you to think about all your coding-related woes—better list those down. Done? – Let’
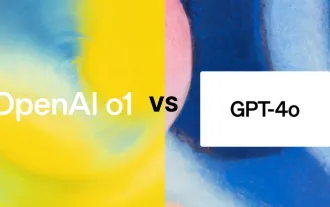
Introduction OpenAI has released its new model based on the much-anticipated “strawberry” architecture. This innovative model, known as o1, enhances reasoning capabilities, allowing it to think through problems mor
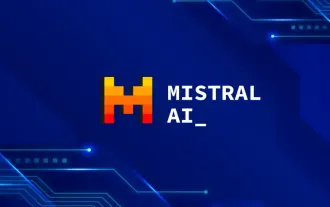
Introduction Mistral has released its very first multimodal model, namely the Pixtral-12B-2409. This model is built upon Mistral’s 12 Billion parameter, Nemo 12B. What sets this model apart? It can now take both images and tex
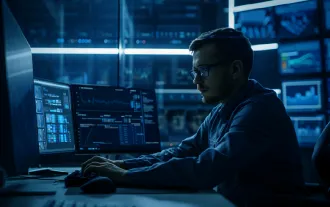
SQL's ALTER TABLE Statement: Dynamically Adding Columns to Your Database In data management, SQL's adaptability is crucial. Need to adjust your database structure on the fly? The ALTER TABLE statement is your solution. This guide details adding colu
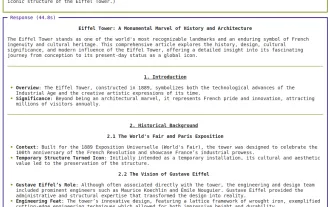
While working on Agentic AI, developers often find themselves navigating the trade-offs between speed, flexibility, and resource efficiency. I have been exploring the Agentic AI framework and came across Agno (earlier it was Phi-
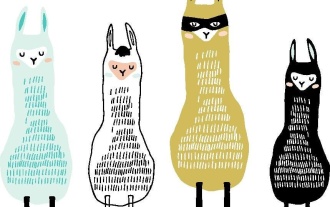
Troubled Benchmarks: A Llama Case Study In early April 2025, Meta unveiled its Llama 4 suite of models, boasting impressive performance metrics that positioned them favorably against competitors like GPT-4o and Claude 3.5 Sonnet. Central to the launc
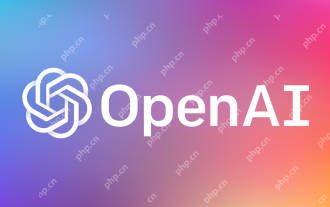
The release includes three distinct models, GPT-4.1, GPT-4.1 mini and GPT-4.1 nano, signaling a move toward task-specific optimizations within the large language model landscape. These models are not immediately replacing user-facing interfaces like
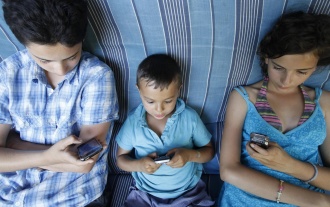
Can a video game ease anxiety, build focus, or support a child with ADHD? As healthcare challenges surge globally — especially among youth — innovators are turning to an unlikely tool: video games. Now one of the world’s largest entertainment indus
