11 Essential GenAI Skills for Engineering Students
Generative AI: 11 Essential Skills for Engineering Students to Thrive
Generative AI is revolutionizing industries, creating exciting opportunities for innovation. For engineering students, mastering GenAI is no longer optional; it's crucial for future success. This article outlines 11 key skills that will equip engineering students to thrive in this rapidly evolving field.
Table of Contents:
- Understanding Neural Networks
- Programming Language Proficiency
- Model Training and Fine-tuning
- Mastering Transformer Architectures
- The Art of Prompt Engineering
- Data Ethics and AI Bias Mitigation
- Hands-on Experience with GenAI Tools
- Essential Mathematical Foundations
- Collaboration and Interdisciplinary Skills
- Integrating AI into Engineering Applications
- RAG and AI Agents: The Future of AI
- Conclusion
- Frequently Asked Questions
1. Understanding Neural Networks:
Neural networks are the foundation of GenAI. Understanding their architecture, activation functions, and backpropagation is vital for comprehending how GenAI systems generate text, images, and more. Familiarity with CNNs and Transformers, leading architectures in GenAI, is also essential. Practical experience building GANs and fine-tuning transformers for specific tasks is highly beneficial.
Key Focus Areas: Network architecture, activation functions, backpropagation, CNNs, Transformers, GANs.
2. Programming Language Proficiency:
Proficiency in Python, with its rich ecosystem of libraries like TensorFlow, PyTorch, and Hugging Face, is indispensable. Students should master algorithm design, debugging, and version control. Experience with coding competitions and open-source projects enhances practical skills.
Key Focus Areas: Python, TensorFlow, PyTorch, Hugging Face, algorithm design, debugging, version control.
3. Model Training and Fine-tuning:
Mastering model training and fine-tuning is crucial for adapting pre-trained models to specific tasks and datasets. Understanding loss functions, optimization algorithms, data preprocessing, and hyperparameter management are key components. Experience fine-tuning large language models and working with RAG systems is highly valuable.
Key Focus Areas: Training pipelines, data preprocessing, hyperparameter tuning, loss functions, optimization algorithms, fine-tuning large language models, RAG systems.
4. Mastering Transformer Architectures:
Transformers are revolutionizing GenAI. Understanding the self-attention mechanism, parallel processing, and multi-head attention is critical. Skills in model optimization, big data handling, and transfer learning are essential for efficient and effective use of transformers.
Key Focus Areas: Self-attention, parallel processing, multi-head attention, model optimization, big data handling, transfer learning.
5. The Art of Prompt Engineering:
Crafting effective prompts is crucial for guiding LLMs and other GenAI models to produce desired outputs. Students should learn to optimize clarity, utilize context effectively, and iteratively refine prompts for improved results.
Key Focus Areas: Prompt optimization, context utilization, iterative refinement, task-specific prompts.
6. Data Ethics and AI Bias Mitigation:
Addressing ethical concerns and mitigating bias in GenAI is paramount. Students should understand the potential for bias in data and models, and learn strategies for diverse data collection, bias detection, and the application of ethical frameworks.
Key Focus Areas: Data bias, ethical frameworks, fairness-aware algorithms, bias detection and mitigation.
7. Hands-on Experience with GenAI Tools:
Practical experience with tools like TensorFlow, PyTorch, Hugging Face, and OpenAI's API is invaluable. Projects involving text-to-image generation, chatbot development, and model fine-tuning provide crucial hands-on learning.
Key Focus Areas: TensorFlow, PyTorch, Hugging Face, OpenAI API, practical project experience.
8. Essential Mathematical Foundations:
A strong foundation in linear algebra, calculus, probability theory, and optimization is essential for understanding the underlying principles of GenAI models.
Key Focus Areas: Linear algebra, calculus, probability theory, optimization algorithms.
9. Collaboration and Interdisciplinary Skills:
GenAI development often requires collaboration across disciplines. Students need strong communication, teamwork, and problem-solving skills to work effectively in multidisciplinary teams.
Key Focus Areas: Cross-functional communication, teamwork, creative problem-solving.
10. Integrating AI into Engineering Applications:
Applying GenAI to solve real-world engineering problems is crucial. Students should learn to identify suitable applications, preprocess data, deploy models, and integrate AI solutions into existing systems.
Key Focus Areas: Problem identification, data preprocessing, model deployment, cross-disciplinary knowledge.
11. RAG and AI Agents: The Future of AI:
Understanding RAG systems and AI agents is crucial for building advanced, autonomous systems. Experience with tools like LlamaIndex, LangChain, AutoGen, and CrewAI is highly beneficial.
Key Focus Areas: RAG systems, AI agents, LlamaIndex, LangChain, AutoGen, CrewAI.
Conclusion:
By mastering these 11 essential skills, engineering students can position themselves for success in the rapidly evolving field of generative AI. This knowledge empowers them to create innovative solutions and drive advancements across numerous industries.
Frequently Asked Questions (FAQs): (Similar to the original, but rephrased for conciseness)
- Q1: What programming languages are essential? A: Python is paramount, with JavaScript and R useful for specific tasks.
- Q2: Why are mathematical foundations important? A: They're crucial for understanding and optimizing AI models.
- Q3: What is prompt engineering? A: It's designing effective inputs to guide AI models.
- Q4: How can students gain hands-on experience? A: Through real-world projects using TensorFlow, PyTorch, and Hugging Face.
- Q5: What key tools should students learn? A: TensorFlow, PyTorch, OpenAI's API, and Hugging Face.
- Q6: What are the most crucial GenAI skills? A: Understanding neural networks, programming proficiency, data ethics, and hands-on experience with leading AI frameworks. These are essential for creating impactful AI applications.
The above is the detailed content of 11 Essential GenAI Skills for Engineering Students. For more information, please follow other related articles on the PHP Chinese website!

Hot AI Tools

Undresser.AI Undress
AI-powered app for creating realistic nude photos

AI Clothes Remover
Online AI tool for removing clothes from photos.

Undress AI Tool
Undress images for free

Clothoff.io
AI clothes remover

Video Face Swap
Swap faces in any video effortlessly with our completely free AI face swap tool!

Hot Article

Hot Tools

Notepad++7.3.1
Easy-to-use and free code editor

SublimeText3 Chinese version
Chinese version, very easy to use

Zend Studio 13.0.1
Powerful PHP integrated development environment

Dreamweaver CS6
Visual web development tools

SublimeText3 Mac version
God-level code editing software (SublimeText3)

Hot Topics










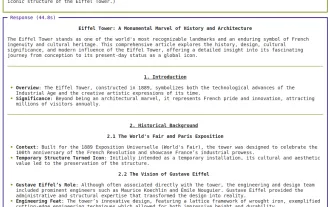
While working on Agentic AI, developers often find themselves navigating the trade-offs between speed, flexibility, and resource efficiency. I have been exploring the Agentic AI framework and came across Agno (earlier it was Phi-
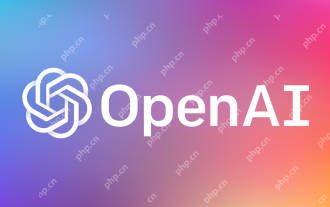
The release includes three distinct models, GPT-4.1, GPT-4.1 mini and GPT-4.1 nano, signaling a move toward task-specific optimizations within the large language model landscape. These models are not immediately replacing user-facing interfaces like
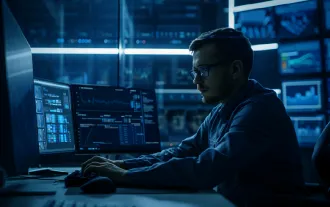
SQL's ALTER TABLE Statement: Dynamically Adding Columns to Your Database In data management, SQL's adaptability is crucial. Need to adjust your database structure on the fly? The ALTER TABLE statement is your solution. This guide details adding colu
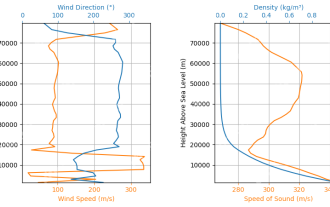
Simulate Rocket Launches with RocketPy: A Comprehensive Guide This article guides you through simulating high-power rocket launches using RocketPy, a powerful Python library. We'll cover everything from defining rocket components to analyzing simula
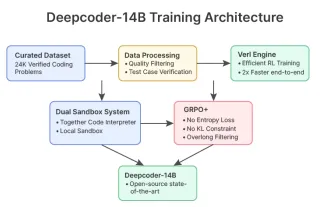
In a significant development for the AI community, Agentica and Together AI have released an open-source AI coding model named DeepCoder-14B. Offering code generation capabilities on par with closed-source competitors like OpenAI
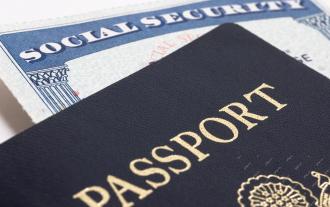
Chip giant Nvidia said on Monday it will start manufacturing AI supercomputers— machines that can process copious amounts of data and run complex algorithms— entirely within the U.S. for the first time. The announcement comes after President Trump si
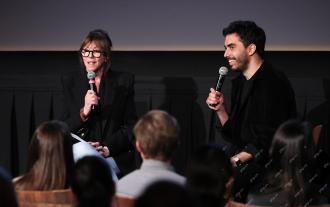
The film industry, alongside all creative sectors, from digital marketing to social media, stands at a technological crossroad. As artificial intelligence begins to reshape every aspect of visual storytelling and change the landscape of entertainment
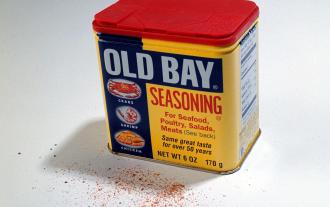
Guy Peri is McCormick’s Chief Information and Digital Officer. Though only seven months into his role, Peri is rapidly advancing a comprehensive transformation of the company’s digital capabilities. His career-long focus on data and analytics informs
