What is Mixture of Experts?
Mixture of Experts (MoE) models are revolutionizing large language models (LLMs) by improving efficiency and scalability. This innovative architecture divides the model into specialized sub-networks, or "experts," each trained for specific data types or tasks. By activating only a relevant subset of experts based on the input, MoE models significantly boost capacity without proportionally increasing computational costs. This selective activation optimizes resource usage and enables handling complex tasks across diverse fields like natural language processing, computer vision, and recommendation systems. This article explores MoE models, their functionality, popular examples, and Python implementation.
This article is part of the Data Science Blogathon.
Table of Contents:
- What are Mixture of Experts (MoEs)?
- MoEs in Deep Learning
- How Do MoE Models Function?
- Prominent MoE-Based Models
- Python Implementation of MoEs
- Comparing Outputs from Different MoE Models
- DBRX
- DeepSeek-v2
- Frequently Asked Questions
What are Mixture of Experts (MoEs)?
MoE models enhance machine learning by employing multiple smaller, specialized models instead of a single large one. Each smaller model excels at a specific problem type. A "decision-maker" (gating mechanism) selects the appropriate model for each task, improving overall performance. Modern deep learning models, including transformers, use layered interconnected units ("neurons") that process data and pass results to subsequent layers. MoE mirrors this by dividing complex problems into specialized components ("experts"), each tackling a specific aspect.
Key Advantages of MoE Models:
- Faster pre-training compared to dense models.
- Quicker inference, even with similar parameter counts.
- High VRAM demand due to simultaneous storage of all experts in memory.
An MoE model comprises two main parts: Experts (specialized smaller neural networks) and a Router (which activates relevant experts based on input). This selective activation boosts efficiency.
MoEs in Deep Learning
In deep learning, MoE improves neural network performance by breaking down complex problems. Instead of a single large model, it uses multiple smaller "expert" models specializing in different input data aspects. A gating network determines which expert(s) to use for each input, enhancing efficiency and effectiveness.
How Do MoE Models Function?
MoE models operate as follows:
- Multiple Experts: The model contains several smaller neural networks ("experts"), each trained for specific input types or tasks.
- Gating Network: A separate neural network (gating network) decides which expert(s) to use for each input, assigning weights to indicate each expert's contribution to the final output.
- Dynamic Routing: The gating network dynamically selects the most relevant expert(s) for each input, optimizing efficiency.
- Combining Outputs: The selected experts' outputs are combined based on the gating network's assigned weights, producing the final prediction.
- Efficiency and Scalability: MoE models are efficient because only a few experts are activated for each input, reducing computational cost. Scalability is achieved by adding more experts to handle more complex tasks without significantly increasing computation per input.
Prominent MoE-Based Models
MoE models are increasingly important in AI due to their efficient scaling of LLMs while maintaining performance. Mixtral 8x7B, a notable example, uses a sparse MoE architecture, activating only a subset of experts for each input, leading to significant efficiency gains.
Mixtral 8x7B
Mixtral 8x7B is a decoder-only transformer. Input tokens are embedded into vectors and processed through decoder layers. The output is the probability of each location being occupied by a word, enabling text infill and prediction. Each decoder layer has an attention mechanism (for contextual information) and a Sparse Mixture of Experts (SMoE) section (individually processing each word vector). SMoE layers use multiple layers ("experts") and, for each input, a weighted sum of the most relevant experts' outputs is taken.
Key Features of Mixtral 8x7B:
- Total Experts: 8
- Active Experts: 2
- Decoder Layers: 32
- Vocab Size: 32000
- Embedding Size: 4096
- Expert Size: 5.6 billion parameters each (total 7 billion with shared components)
- Active Parameters: 12.8 billion
- Context Length: 32k Tokens
Mixtral 8x7B excels in text generation, comprehension, translation, summarization, and more.
DBRX
DBRX (Databricks) is a transformer-based decoder-only LLM trained using next-token prediction. It uses a fine-grained MoE architecture (132B total parameters, 36B active). It was pre-trained on 12T tokens of text and code data. DBRX is fine-grained, using many smaller experts (16 experts, 4 selected per input).
Key Architectural Features of DBRX:
- Fine-grained experts: A single FFN is divided into segments, each acting as an expert.
- Other techniques: Rotary Position Encodings (RoPE), Gated Linear Units (GLU), and Grouped Query Attention (GQA).
Key Features of DBRX:
- Total Experts: 16
- Active Experts per Layer: 4
- Decoder Layers: 24
- Active Parameters: 36 billion
- Total Parameters: 132 billion
- Context Length: 32k Tokens
DBRX excels in code generation, complex language understanding, and mathematical reasoning.
Deepseek-v2
Deepseek-v2 uses fine-grained experts and shared experts (always active) to integrate universal knowledge.
Key Features of Deepseek-v2:
- Total Parameters: 236 billion
- Active Parameters: 21 billion
- Routed Experts per Layer: 160 (2 selected)
- Shared Experts per Layer: 2
- Active Experts per Layer: 8
- Decoder Layers: 60
- Context Length: 128k Tokens
DeepSeek-v2 is adept at conversations, content creation, and code generation.
(Python Implementation and Output Comparisons sections removed for brevity, as they are lengthy code examples and detailed analyses.)
Frequently Asked Questions
Q1. What are Mixture of Experts (MoE) models? A. MoE models use a sparse architecture, activating only the most relevant experts for each task, leading to reduced computational resource usage.
Q2. What is the trade-off with MoE models? A. MoE models require significant VRAM to store all experts in memory, balancing computational power and memory requirements.
Q3. What is the active parameter count for Mixtral 8x7B? A. Mixtral 8x7B has 12.8 billion active parameters.
Q4. How does DBRX differ from other MoE models? A. DBRX uses a fine-grained MoE approach with more smaller experts.
Q5. What distinguishes DeepSeek-v2? A. DeepSeek-v2 combines fine-grained and shared experts, along with a large parameter set and long context length.
Conclusion
MoE models offer a highly efficient approach to deep learning. While requiring significant VRAM, their selective activation of experts makes them powerful tools for handling complex tasks across various domains. Mixtral 8x7B, DBRX, and DeepSeek-v2 represent significant advancements in this area, each with its own strengths and applications.
The above is the detailed content of What is Mixture of Experts?. For more information, please follow other related articles on the PHP Chinese website!

Hot AI Tools

Undresser.AI Undress
AI-powered app for creating realistic nude photos

AI Clothes Remover
Online AI tool for removing clothes from photos.

Undress AI Tool
Undress images for free

Clothoff.io
AI clothes remover

Video Face Swap
Swap faces in any video effortlessly with our completely free AI face swap tool!

Hot Article

Hot Tools

Notepad++7.3.1
Easy-to-use and free code editor

SublimeText3 Chinese version
Chinese version, very easy to use

Zend Studio 13.0.1
Powerful PHP integrated development environment

Dreamweaver CS6
Visual web development tools

SublimeText3 Mac version
God-level code editing software (SublimeText3)

Hot Topics










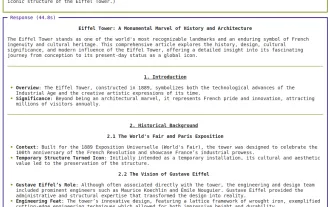
While working on Agentic AI, developers often find themselves navigating the trade-offs between speed, flexibility, and resource efficiency. I have been exploring the Agentic AI framework and came across Agno (earlier it was Phi-
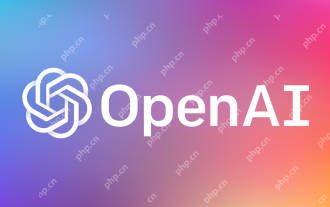
The release includes three distinct models, GPT-4.1, GPT-4.1 mini and GPT-4.1 nano, signaling a move toward task-specific optimizations within the large language model landscape. These models are not immediately replacing user-facing interfaces like
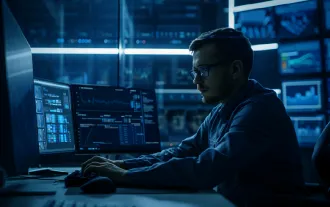
SQL's ALTER TABLE Statement: Dynamically Adding Columns to Your Database In data management, SQL's adaptability is crucial. Need to adjust your database structure on the fly? The ALTER TABLE statement is your solution. This guide details adding colu
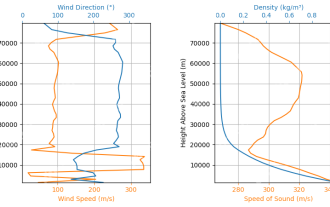
Simulate Rocket Launches with RocketPy: A Comprehensive Guide This article guides you through simulating high-power rocket launches using RocketPy, a powerful Python library. We'll cover everything from defining rocket components to analyzing simula
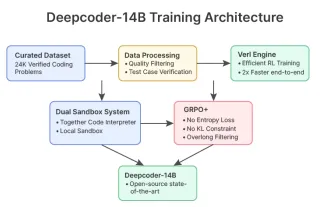
In a significant development for the AI community, Agentica and Together AI have released an open-source AI coding model named DeepCoder-14B. Offering code generation capabilities on par with closed-source competitors like OpenAI
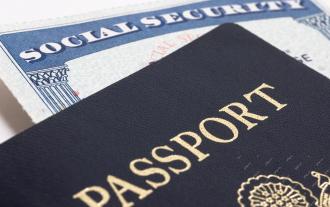
Chip giant Nvidia said on Monday it will start manufacturing AI supercomputers— machines that can process copious amounts of data and run complex algorithms— entirely within the U.S. for the first time. The announcement comes after President Trump si
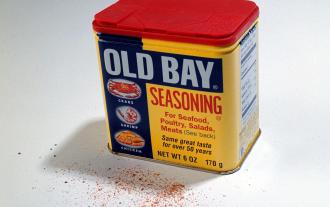
Guy Peri is McCormick’s Chief Information and Digital Officer. Though only seven months into his role, Peri is rapidly advancing a comprehensive transformation of the company’s digital capabilities. His career-long focus on data and analytics informs
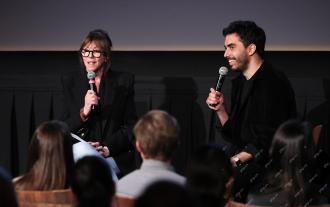
The film industry, alongside all creative sectors, from digital marketing to social media, stands at a technological crossroad. As artificial intelligence begins to reshape every aspect of visual storytelling and change the landscape of entertainment
