Corrective RAG (CRAG) in Action
Retrieval-Augmented Generation (RAG) empowers large language models (LLMs) by incorporating information retrieval. This allows LLMs to access external knowledge bases, resulting in more accurate, current, and contextually appropriate responses. Corrective RAG (CRAG), an advanced RAG technique, further enhances accuracy by introducing self-reflection and self-assessment mechanisms for retrieved documents.
Key Learning Objectives
This article covers:
- CRAG's core mechanism and its integration with web search.
- CRAG's document relevance evaluation using binary scoring and query rewriting.
- Key distinctions between CRAG and traditional RAG.
- Hands-on CRAG implementation using Python, LangChain, and Tavily.
- Practical skills in configuring evaluators, query rewriters, and web search tools to optimize retrieval and response accuracy.
Published as part of the Data Science Blogathon.
Table of Contents
- CRAG's Underlying Mechanism
- CRAG vs. Traditional RAG
- Practical CRAG Implementation
- CRAG's Challenges
- Conclusion
- Frequently Asked Questions
CRAG's Underlying Mechanism
CRAG enhances the dependability of LLM outputs by integrating web search into its retrieval and generation processes (see Figure 1).
Document Retrieval:
- Data Ingestion: Relevant data is indexed, and web search tools (like Tavily AI) are configured for real-time data retrieval.
- Initial Retrieval: Documents are retrieved from a static knowledge base based on the user's query.
Relevance Assessment:
An evaluator assesses retrieved document relevance. If over 70% of documents are deemed irrelevant, corrective actions are initiated; otherwise, response generation proceeds.
Web Search Integration:
If document relevance is insufficient, CRAG uses web search:
- Query Refinement: The original query is modified to optimize web search results.
- Web Search Execution: Tools like Tavily AI fetch additional data, ensuring access to current and diverse information.
Response Generation:
CRAG synthesizes data from both initial retrieval and web searches to create a coherent, accurate response.
CRAG vs. Traditional RAG
CRAG actively verifies and refines retrieved information, unlike traditional RAG, which relies on retrieved documents without verification. CRAG often incorporates real-time web search, providing access to the most up-to-date information, unlike traditional RAG's reliance on static knowledge bases. This makes CRAG ideal for applications requiring high accuracy and real-time data integration.
Practical CRAG Implementation
This section details a CRAG implementation using Python, LangChain, and Tavily.
Step 1: Library Installation
Install necessary libraries:
!pip install tiktoken langchain-openai langchainhub chromadb langchain langgraph tavily-python !pip install -qU pypdf langchain_community
Step 2: API Key Configuration
Set your API keys:
import os os.environ["TAVILY_API_KEY"] = "" os.environ["OPENAI_API_KEY"] = ""
Step 3: Library Imports
Import required libraries (code omitted for brevity, but similar to the original example).
Step 4: Document Chunking and Retriever Creation
(Code omitted for brevity, but similar to the original example, using PyPDFLoader, RecursiveCharacterTextSplitter, OpenAIEmbeddings, and Chroma).
Step 5: RAG Chain Setup
(Code omitted for brevity, but similar to the original example, using hub.pull("rlm/rag-prompt")
and ChatOpenAI
).
Step 6: Evaluator Setup
(Code omitted for brevity, but similar to the original example, defining the Evaluator
class and using ChatOpenAI
for evaluation).
Step 7: Query Rewriter Setup
(Code omitted for brevity, but similar to the original example, using ChatOpenAI
for query rewriting).
Step 8: Web Search Setup
from langchain_community.tools.tavily_search import TavilySearchResults web_search_tool = TavilySearchResults(k=3)
Step 9-12: LangGraph Workflow Setup and Execution
(Code omitted for brevity, but conceptually similar to the original example, defining the GraphState
, function nodes (retrieve
, generate
, evaluate_documents
, transform_query
, web_search
), and connecting them using StateGraph
.) The final output and comparison with traditional RAG are also conceptually similar.
CRAG's Challenges
CRAG's effectiveness depends heavily on the evaluator's accuracy. A weak evaluator can introduce errors. Scalability and adaptability are also concerns, requiring continuous updates and training. Web search integration introduces the risk of biased or unreliable information, necessitating robust filtering mechanisms.
Conclusion
CRAG significantly improves LLM output accuracy and reliability. Its ability to evaluate and supplement retrieved information with real-time web data makes it valuable for applications demanding high precision and up-to-date information. However, continuous refinement is crucial to address the challenges related to evaluator accuracy and web data reliability.
Key Takeaways (similar to the original, but rephrased for conciseness)
- CRAG enhances LLM responses using web search for current, relevant information.
- Its evaluator ensures high-quality information for response generation.
- Query transformation optimizes web search results.
- CRAG dynamically integrates real-time web data, unlike traditional RAG.
- CRAG actively verifies information, reducing errors.
- CRAG is beneficial for applications needing high accuracy and real-time data.
Frequently Asked Questions (similar to the original, but rephrased for conciseness)
- Q1: What is CRAG? A: An advanced RAG framework integrating web search for improved accuracy and reliability.
- Q2: CRAG vs. Traditional RAG? A: CRAG actively verifies and refines retrieved information.
- Q3: The evaluator's role? A: Assessing document relevance and triggering corrections.
- Q4: Insufficient documents? A: CRAG supplements with web search.
- Q5: Handling unreliable web content? A: Advanced filtering methods are needed.
(Note: The image remains unchanged and is included as in the original input.)
The above is the detailed content of Corrective RAG (CRAG) in Action. For more information, please follow other related articles on the PHP Chinese website!

Hot AI Tools

Undresser.AI Undress
AI-powered app for creating realistic nude photos

AI Clothes Remover
Online AI tool for removing clothes from photos.

Undress AI Tool
Undress images for free

Clothoff.io
AI clothes remover

Video Face Swap
Swap faces in any video effortlessly with our completely free AI face swap tool!

Hot Article

Hot Tools

Notepad++7.3.1
Easy-to-use and free code editor

SublimeText3 Chinese version
Chinese version, very easy to use

Zend Studio 13.0.1
Powerful PHP integrated development environment

Dreamweaver CS6
Visual web development tools

SublimeText3 Mac version
God-level code editing software (SublimeText3)

Hot Topics
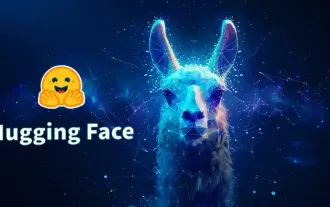
Meta's Llama 3.2: A Leap Forward in Multimodal and Mobile AI Meta recently unveiled Llama 3.2, a significant advancement in AI featuring powerful vision capabilities and lightweight text models optimized for mobile devices. Building on the success o
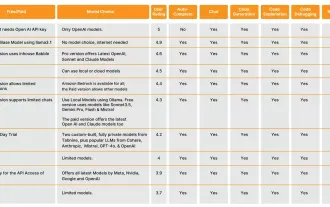
Hey there, Coding ninja! What coding-related tasks do you have planned for the day? Before you dive further into this blog, I want you to think about all your coding-related woes—better list those down. Done? – Let’
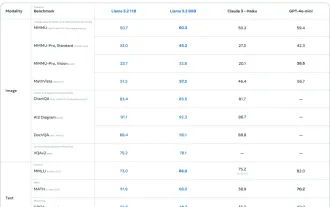
This week's AI landscape: A whirlwind of advancements, ethical considerations, and regulatory debates. Major players like OpenAI, Google, Meta, and Microsoft have unleashed a torrent of updates, from groundbreaking new models to crucial shifts in le
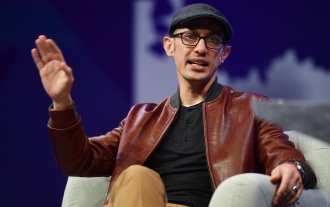
Shopify CEO Tobi Lütke's recent memo boldly declares AI proficiency a fundamental expectation for every employee, marking a significant cultural shift within the company. This isn't a fleeting trend; it's a new operational paradigm integrated into p
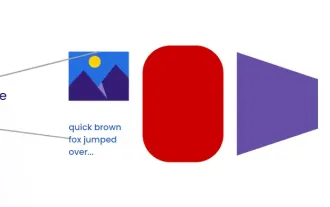
Introduction Imagine walking through an art gallery, surrounded by vivid paintings and sculptures. Now, what if you could ask each piece a question and get a meaningful answer? You might ask, “What story are you telling?
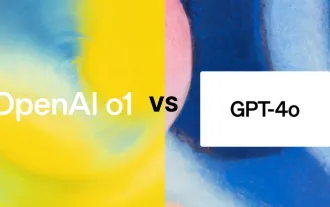
Introduction OpenAI has released its new model based on the much-anticipated “strawberry” architecture. This innovative model, known as o1, enhances reasoning capabilities, allowing it to think through problems mor
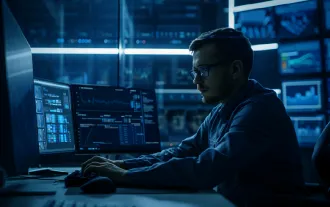
SQL's ALTER TABLE Statement: Dynamically Adding Columns to Your Database In data management, SQL's adaptability is crucial. Need to adjust your database structure on the fly? The ALTER TABLE statement is your solution. This guide details adding colu
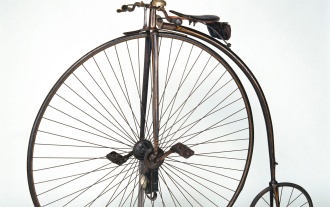
The 2025 Artificial Intelligence Index Report released by the Stanford University Institute for Human-Oriented Artificial Intelligence provides a good overview of the ongoing artificial intelligence revolution. Let’s interpret it in four simple concepts: cognition (understand what is happening), appreciation (seeing benefits), acceptance (face challenges), and responsibility (find our responsibilities). Cognition: Artificial intelligence is everywhere and is developing rapidly We need to be keenly aware of how quickly artificial intelligence is developing and spreading. Artificial intelligence systems are constantly improving, achieving excellent results in math and complex thinking tests, and just a year ago they failed miserably in these tests. Imagine AI solving complex coding problems or graduate-level scientific problems – since 2023
