


CoCa: Contrastive Captioners are Image-Text Foundation Models Visually Explained
This DataCamp community tutorial, edited for clarity and accuracy, explores image-text foundation models, focusing on the innovative Contrastive Captioner (CoCa) model. CoCa uniquely combines contrastive and generative learning objectives, integrating the strengths of models like CLIP and SIMVLM into a single architecture.
Foundation Models: A Deep Dive
Foundation models, pre-trained on massive datasets, are adaptable for various downstream tasks. While NLP has seen a surge in foundation models (GPT, BERT), vision and vision-language models are still evolving. Research has explored three primary approaches: single-encoder models, image-text dual-encoders with contrastive loss, and encoder-decoder models with generative objectives. Each approach has limitations.
Key Terms:
- Foundation Models: Pre-trained models adaptable for diverse applications.
- Contrastive Loss: A loss function comparing similar and dissimilar input pairs.
- Cross-Modal Interaction: Interaction between different data types (e.g., image and text).
- Encoder-Decoder Architecture: A neural network processing input and generating output.
- Zero-Shot Learning: Predicting on unseen data classes.
- CLIP: A contrastive language-image pre-training model.
- SIMVLM: A simple visual language model.
Model Comparisons:
- Single Encoder Models: Excel at vision tasks but struggle with vision-language tasks due to reliance on human annotations.
- Image-Text Dual-Encoder Models (CLIP, ALIGN): Excellent for zero-shot classification and image retrieval, but limited in tasks requiring fused image-text representations (e.g., Visual Question Answering).
- Generative Models (SIMVLM): Use cross-modal interaction for joint image-text representation, suitable for VQA and image captioning.
CoCa: Bridging the Gap
CoCa aims to unify the strengths of contrastive and generative approaches. It uses a contrastive loss to align image and text representations and a generative objective (captioning loss) to create a joint representation.
CoCa Architecture:
CoCa employs a standard encoder-decoder structure. Its innovation lies in a decoupled decoder:
- Lower Decoder: Generates a unimodal text representation for contrastive learning (using a [CLS] token).
- Upper Decoder: Generates a multimodal image-text representation for generative learning. Both decoders use causal masking.
Contrastive Objective: Learns to cluster related image-text pairs and separate unrelated ones in a shared vector space. A single pooled image embedding is used.
Generative Objective: Uses a fine-grained image representation (256-dimensional sequence) and cross-modal attention to predict text autoregressively.
Conclusion:
CoCa represents a significant advancement in image-text foundation models. Its combined approach enhances performance in various tasks, offering a versatile tool for downstream applications. To further your understanding of advanced deep learning concepts, consider DataCamp's Advanced Deep Learning with Keras course.
Further Reading:
- Learning Transferable Visual Models From Natural Language Supervision
- Image-Text Pre-training with Contrastive Captioners
The above is the detailed content of CoCa: Contrastive Captioners are Image-Text Foundation Models Visually Explained. For more information, please follow other related articles on the PHP Chinese website!

Hot AI Tools

Undresser.AI Undress
AI-powered app for creating realistic nude photos

AI Clothes Remover
Online AI tool for removing clothes from photos.

Undress AI Tool
Undress images for free

Clothoff.io
AI clothes remover

Video Face Swap
Swap faces in any video effortlessly with our completely free AI face swap tool!

Hot Article

Hot Tools

Notepad++7.3.1
Easy-to-use and free code editor

SublimeText3 Chinese version
Chinese version, very easy to use

Zend Studio 13.0.1
Powerful PHP integrated development environment

Dreamweaver CS6
Visual web development tools

SublimeText3 Mac version
God-level code editing software (SublimeText3)

Hot Topics










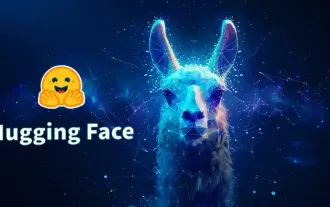
Meta's Llama 3.2: A Leap Forward in Multimodal and Mobile AI Meta recently unveiled Llama 3.2, a significant advancement in AI featuring powerful vision capabilities and lightweight text models optimized for mobile devices. Building on the success o
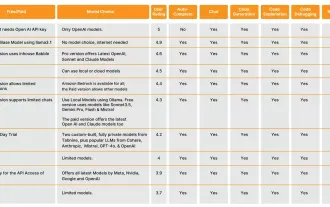
Hey there, Coding ninja! What coding-related tasks do you have planned for the day? Before you dive further into this blog, I want you to think about all your coding-related woes—better list those down. Done? – Let’
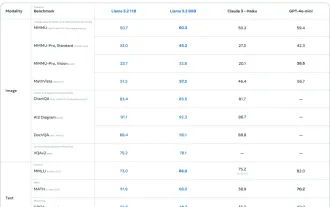
This week's AI landscape: A whirlwind of advancements, ethical considerations, and regulatory debates. Major players like OpenAI, Google, Meta, and Microsoft have unleashed a torrent of updates, from groundbreaking new models to crucial shifts in le
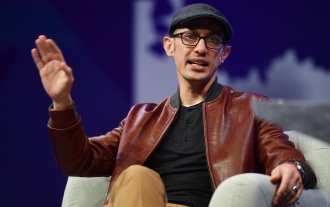
Shopify CEO Tobi Lütke's recent memo boldly declares AI proficiency a fundamental expectation for every employee, marking a significant cultural shift within the company. This isn't a fleeting trend; it's a new operational paradigm integrated into p
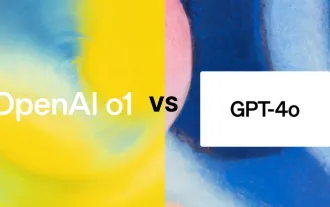
Introduction OpenAI has released its new model based on the much-anticipated “strawberry” architecture. This innovative model, known as o1, enhances reasoning capabilities, allowing it to think through problems mor
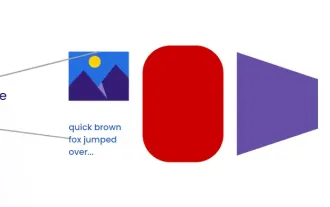
Introduction Imagine walking through an art gallery, surrounded by vivid paintings and sculptures. Now, what if you could ask each piece a question and get a meaningful answer? You might ask, “What story are you telling?
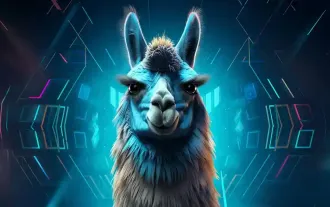
Meta's Llama 3.2: A Multimodal AI Powerhouse Meta's latest multimodal model, Llama 3.2, represents a significant advancement in AI, boasting enhanced language comprehension, improved accuracy, and superior text generation capabilities. Its ability t
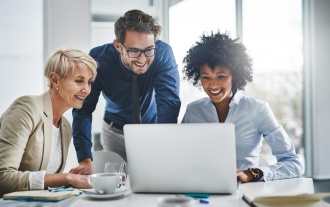
For those of you who might be new to my column, I broadly explore the latest advances in AI across the board, including topics such as embodied AI, AI reasoning, high-tech breakthroughs in AI, prompt engineering, training of AI, fielding of AI, AI re
